WPS:A whole phenology-based spectral feature selection method for mapping winter crop from time-series images
ISPRS Journal of Photogrammetry and Remote Sensing(2024)
摘要
Accurately obtaining the spatial distribution and planting patterns of crops is very important for agricultural planning and food security. At present, time-series images have been proved to be an effective tool to characterize crop seasonal growth patterns, and identifying crop information by measuring the time-series similarity between unknown classes and known crop phenology curves is also considered to be a useful way. However, the existing methods of selecting feature ignore the connection between each phenological stage of crops and the unique growth rules of the whole phenology, which makes it difficult to select time-series spectral features that are potentially important for crop mapping. In order to make up for this problem, a Whole Phenology-based Spectral Feature Selection (WPS) method was proposed. The aim was to select the time-series feature sets with great differences among winter crops from a large number of spectral features, so as to improve the mapping accuracy of winter rapeseed and winter wheat. Firstly, spectral separability between all classes is calculated. Secondly, the key phenological periods of winter crops were selected according to the importance of temporal features, and the spectral feature sets with high separability were selected according to the key phenological periods. Finally, a Time-weighted Dynamic Time Warping (TWDTW) algorithm was used to generate the winter rapeseed and winter wheat maps of two cities in the middle and lower reaches of the Yangtze River. The mapping accuracy of the two crops is more than 92%, which matches the crop planting area well. The research shows that combining the WPS method with the TWDTW mapping method has great potential to accurately map crop types based on satellite time-series images.
更多查看译文
关键词
Phenological spectral feature,Feature selection,Winter crop mapping,Spectral separability,Time-weighted dynamic time warping
AI 理解论文
溯源树
样例
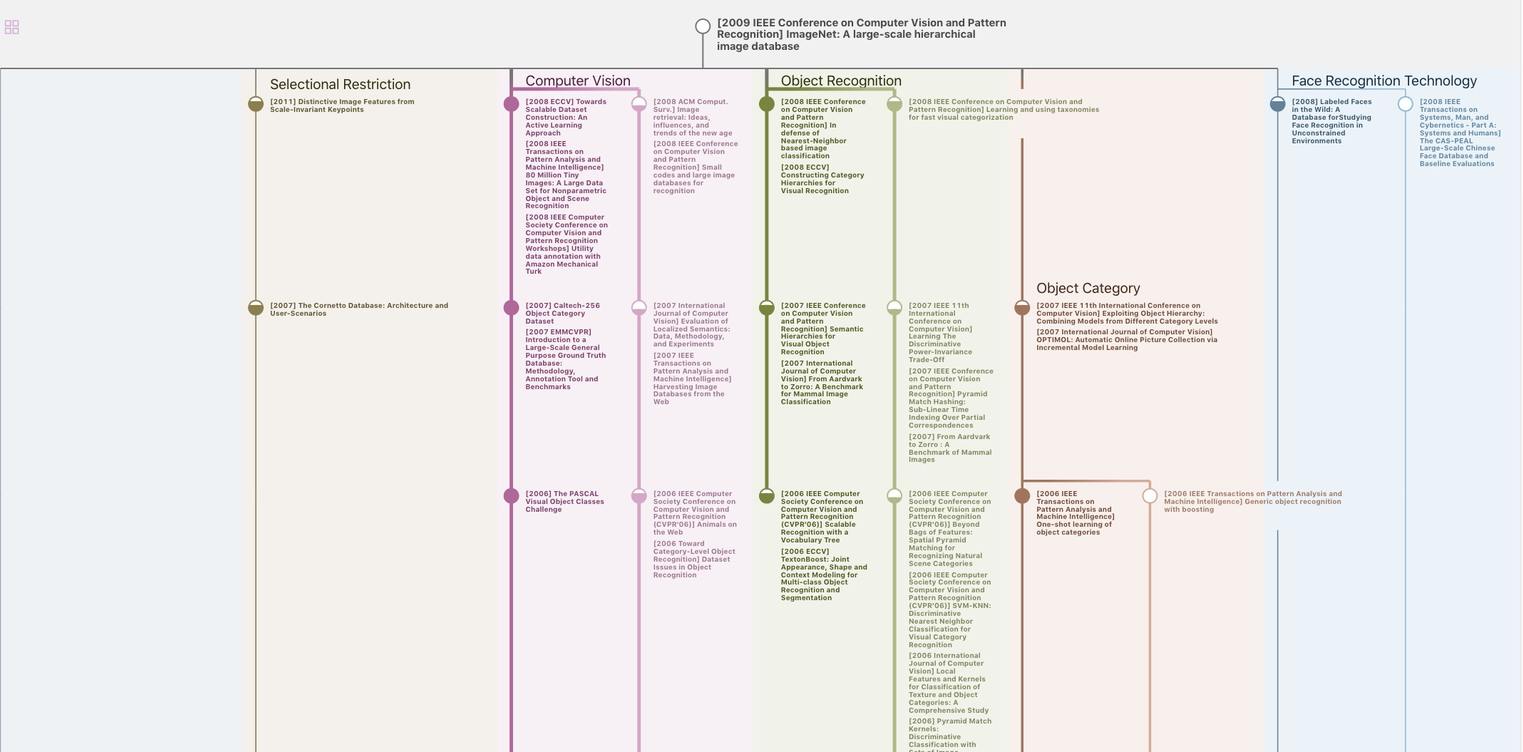
生成溯源树,研究论文发展脉络
Chat Paper
正在生成论文摘要