Multi-Modal Relational Side Information Graph Attention Networks for Recommender System.
International Conference on Machine Learning and Applications(2023)
摘要
With the rapid growth of online information, recommender systems have become an effective strategy for handling information overload. One of the most important factors affecting the user's purchase activities is the side information of the item. Recently, some research has shown that analyzing the side information (e.g., visual and textual information) in multiple modalities can improve the performance of the recommender system. Existing works on multimedia recommendation using graph neural networks largely depend on the interaction records, while less effort focuses on the deeper relationships between interactions and different types of side information. In this paper, we propose a novel graph structure by combining the edges of user-item interactions and relational edges of side information in different modalities. Each modality contains a specific graph structure to learn the representations of users and items to make the recommendation. We design a Multi-modal Relational Side Information Graph Attention Networks (MRSI-GAT) framework built upon the message-passing with attention mechanism of graph attention networks. We conduct experiments on two public datasets, Amazon and MovieLens, and the results of the model outperform the state-of-the-art methods.
更多查看译文
AI 理解论文
溯源树
样例
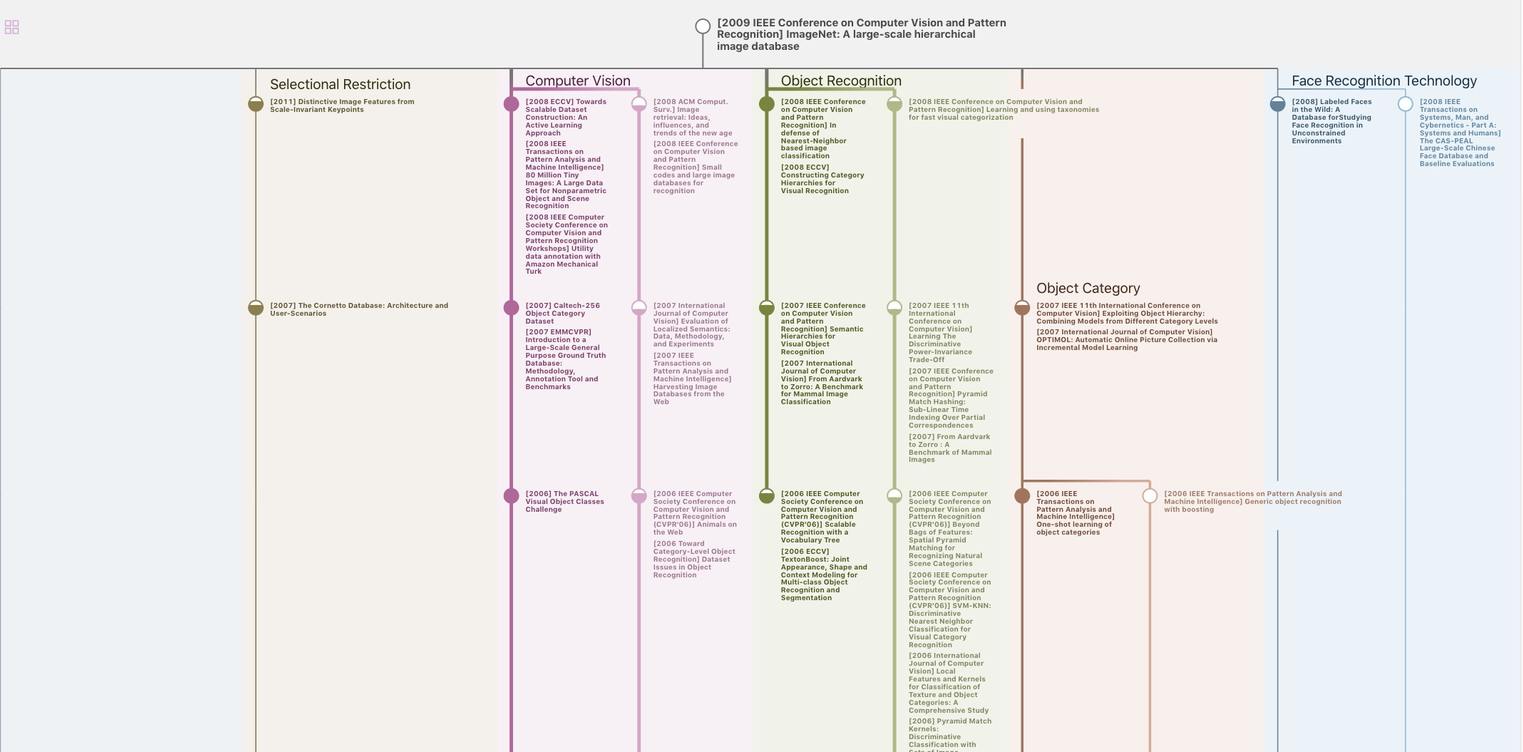
生成溯源树,研究论文发展脉络
Chat Paper
正在生成论文摘要