Adaptive Optimal Control via Q-Learning for Itô Fuzzy Stochastic Nonlinear Continuous-Time Systems With Stackelberg Game.
IEEE Trans. Fuzzy Syst.(2024)
摘要
In order to solve the two-player Stackelberg game for the continuous-time nonlinear stochastic system, using the Takagi–Sugeno (T-S) fuzzy stochastic model, this paper defines the novel
$Q$
-functions and suggests an adaptive dynamic programming (ADP)-based approach that is completely model-free. First, based on the T-S fuzzy model, the overall fuzzy control policies with corresponding cost functions are designed where coupled penalty functions are considered. Subsequently, we create a novel two-level algorithm based on integral reinforcement learning and provide the proof of convergence to overcome challenge of computing the optimal cost functions analytically. On this basis, in order to achieve entirely model-free learning, which is the first attempt in solving fuzzy stochastic nonlinear continuous-time systems with the Stackelberg game problem, the innovative action-dependent
$Q$
-functions are developed. Fuzzy linearization technique and
$Q$
-learning algorithm are ingeniously combined in this article to solve their respective difficulties. In addition, the Lyapunov approach under the ADP-based control scheme ensures the stability of the closed-loop nonlinear stochastic system based on fuzzy approximation and is characterized by asymptotic stability. Finally, a numerical simulation is offered to show the efficacy of the existing ADP-based control technique.
更多查看译文
关键词
Adaptive dynamic programming (ADP),fuzzy stochastic nonlinear system,$Q$ -learning,stackelberg game
AI 理解论文
溯源树
样例
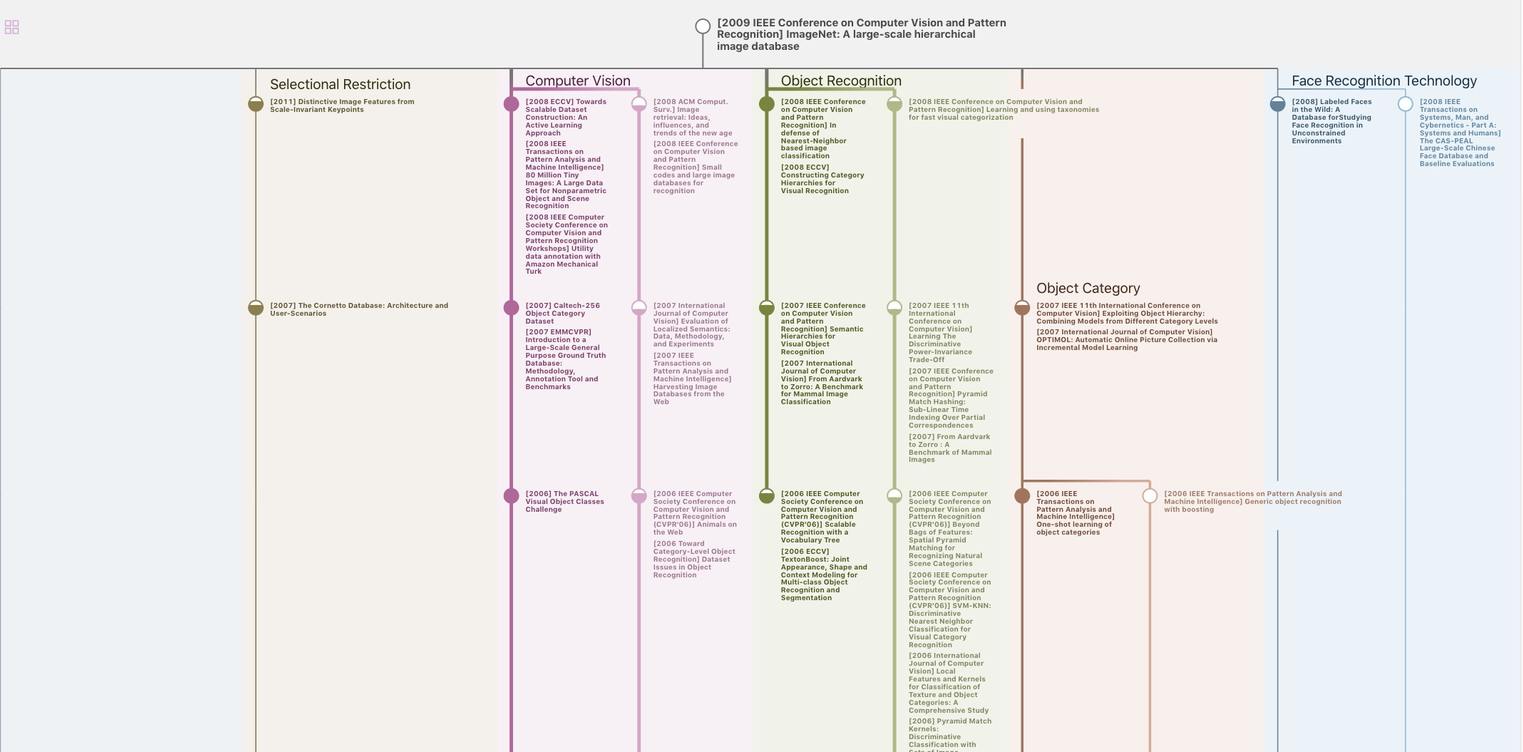
生成溯源树,研究论文发展脉络
Chat Paper
正在生成论文摘要