4DPM: Deepfake Detection with a Denoising Diffusion Probabilistic Mask
IEEE Signal Processing Letters(2024)
摘要
In the face of increasingly realistic fake human faces, research on enhancing the differences between real and fake images is valuable for improving the generalization capabilities of fake face detection models. In this letter, we propose a method called DPMask (Diffusion Probabilistic Mask) to amplify the distinctions between authentic and counterfeit human facial images. Specifically, we use a dataset consisting of real human facial images and Simplex noise to train a denoising diffusion probabilistic model for the proposed DPMask. Subsequently, we separately apply the DPMask and U-Net to real and fake human facial images to create noticeably distinct genuine and counterfeit human facial images. A lightweight classification network blue is further designed based on RepVGG to classify the newly generated real and fake human faces. Experimental results demonstrate that our model achieves high accuracy on a manually created fake face dataset (RFFD), a GAN-generated fake face dataset (Seq-DeepFake), and a DDPM-generated face dataset (HiFi-IFDL). Furthermore, the addition of DPMask significantly improves the performance of some public fake face detection models.
更多查看译文
关键词
Deepfake Detection,DDPM,DPMask,Lightweight Model
AI 理解论文
溯源树
样例
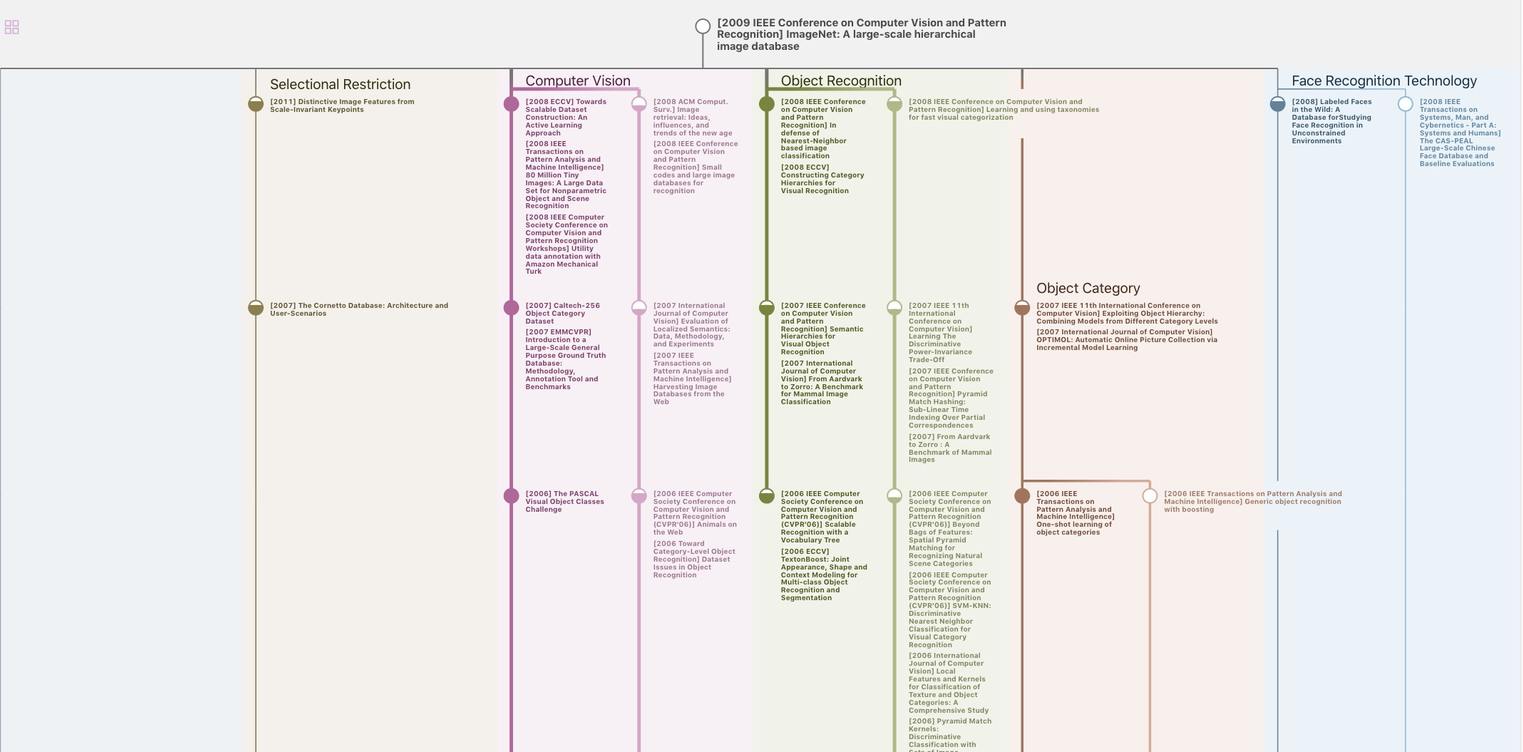
生成溯源树,研究论文发展脉络
Chat Paper
正在生成论文摘要