Low-Correlation Node Filter-Based Neural Subgraph Matching
2023 China Automation Congress (CAC)(2023)
摘要
To overcome the limitations of approximate subgraph matching in terms of time and accuracy, researchers have proposed algorithms based on graph neural networks(GNNs), such as NeuroMatch. However, GNNs-based approximate subgraph matching algorithms, during the matching process, are unable to differentiate low-correlated nodes in the target graph from the query graph, resulting in a decrease in query efficiency. Additionally, they cannot determine the matching relationships between nodes, which limits the application domains and tasks, such as graph matching verification, integration of graph datasets. In this paper, we propose the Low-correlation Node Filtrate-based Neural Subgraph Matching(LNFSM). We establish a candidate space set and filter out low-correlation nodes in the target graph. Then, the matching relationship between the nodes of the query graph and the subgraph of the target graph is determined by combining the filtered candidate space and the ordered embedding space. Experimental results on multiple datasets in domains such as COX2 and AIDS, demonstrate the effectiveness and efficiency of our method compared to approximate matching algorithms such as ISORANKN, FastPFP, and NeuroMatch. Compared to NeuroMatch, our method reduces redundant queries during the matching process, and expanding the application domain and task scope.
更多查看译文
关键词
Subgraph matching,Approximate subgraph matching,Node embedding,Graph neural networks
AI 理解论文
溯源树
样例
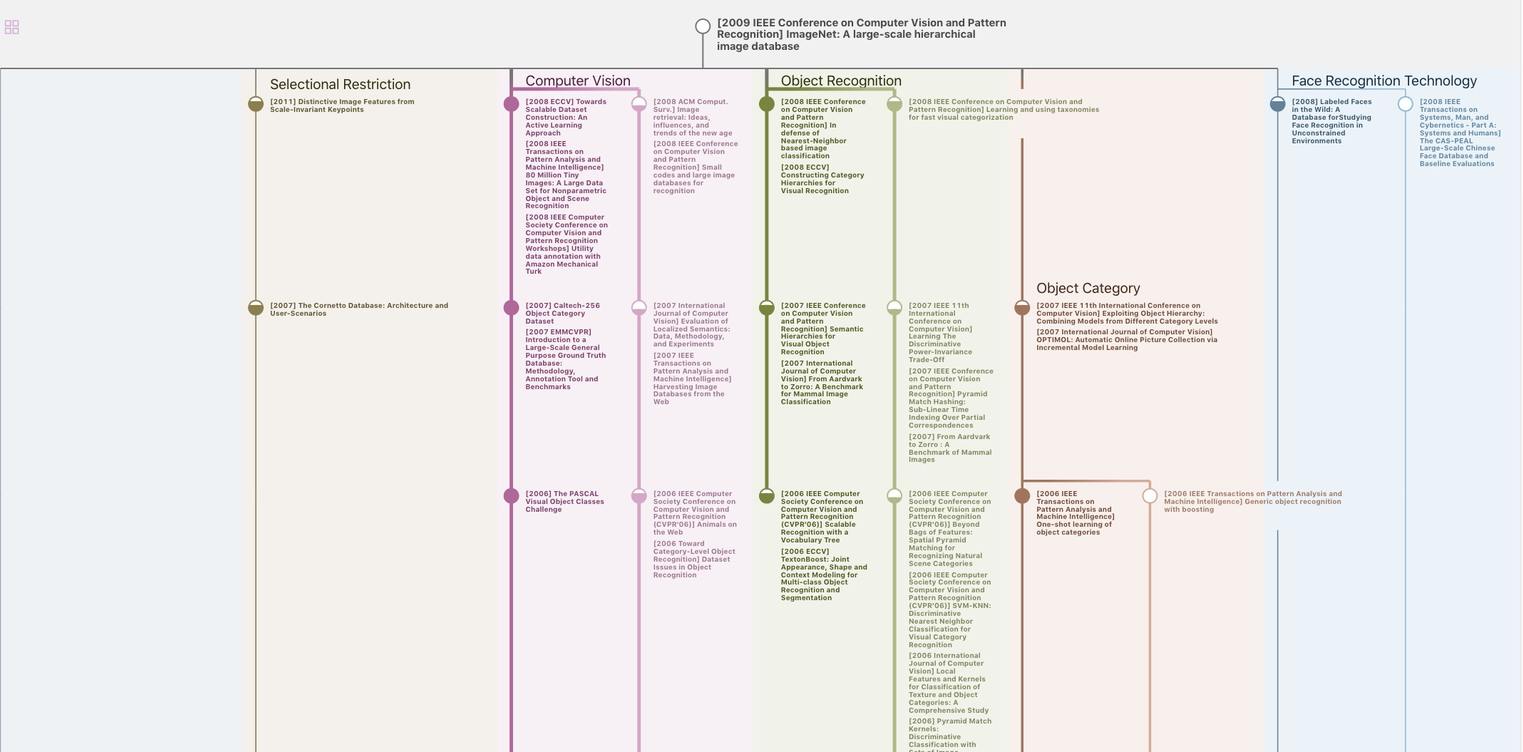
生成溯源树,研究论文发展脉络
Chat Paper
正在生成论文摘要