Graph-based Multimodal Topic Modeling with Word Relations and Object Relations
IEEE Transactions on Multimedia(2024)
摘要
In recent years, multimodal topic models have gained significant attention in various tasks involving short texts. Despite their impressive results, most models rely on bag-of-words assumptions for each modality, neglecting the intrinsic word relations in the textual modality and the underlying object relations in the visual modality. To address this limitation, we propose a novel approach that represents each document modality as a graph, harnessing the word relations and the visual object relations to guide the topic extraction process. Our approach is grounded in the insight that, in the textual modality, words with specific relations, such as co-occurrence relations, semantic relations and syntactic relations, are more likely to be assigned to the same topic. Similarly, in the visual modality, the relations between objects, such as spatial relations and contextual relations, can also provide valuable information for topic extraction. By leveraging graph-based representations, our model captures the inherent associations between words and visual objects, resulting in the generation of more coherent and interpretable topics. To infer the model's parameters, we develop an effective algorithm that integrates neural variational inference and contrastive learning. The experimental results on three datasets verify the effectiveness of our proposed model in terms of topic coherence, topic diversity and mean average precision, confirming that incorporating word relations and object relations through graph-based representations significantly enhances the quality of the extracted topics.
更多查看译文
关键词
multimodal topic model,neural variational inference,graph convolutional network
AI 理解论文
溯源树
样例
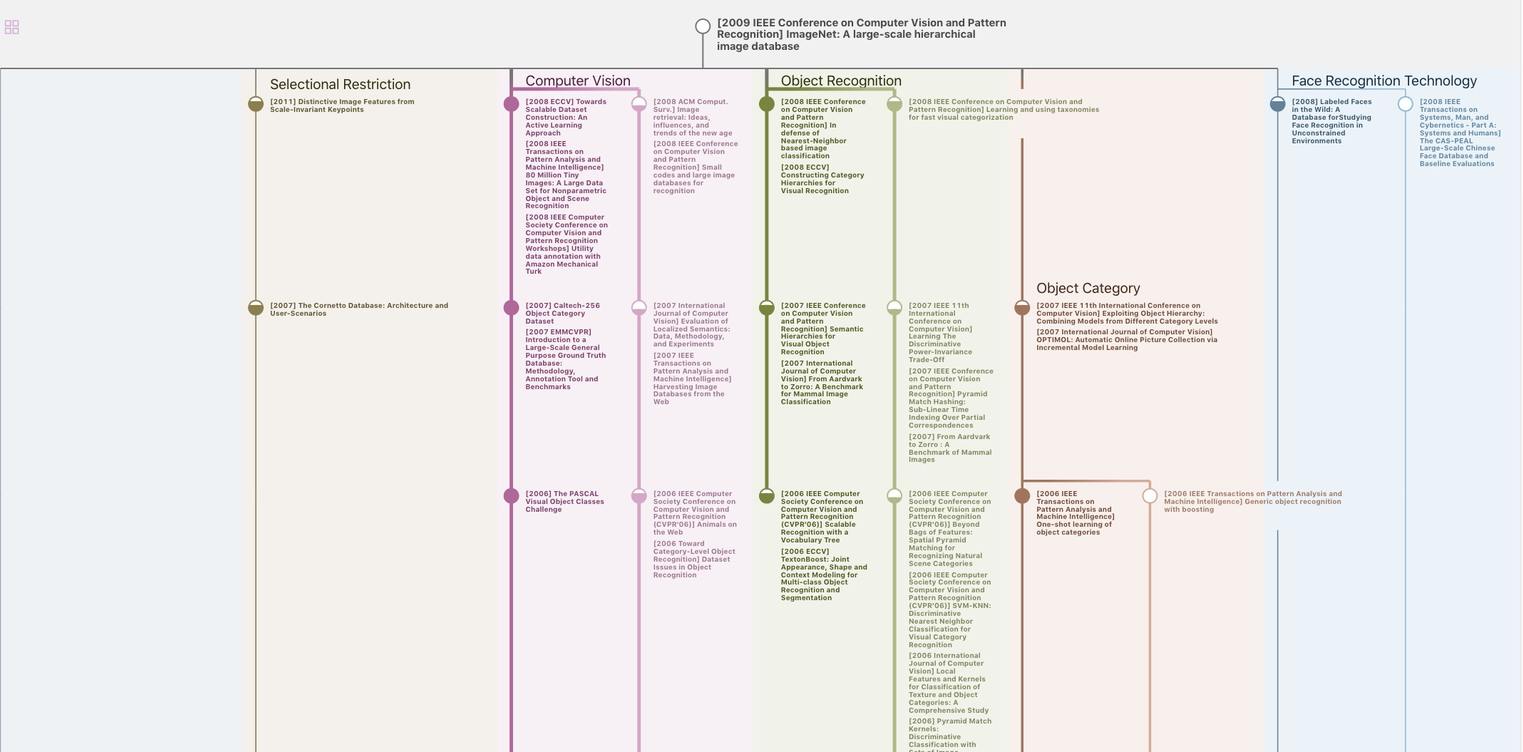
生成溯源树,研究论文发展脉络
Chat Paper
正在生成论文摘要