RM-Net: An Encrypted Traffic Classification Method Based on Deep Learning for Low Labeled Rate Scenarios
2023 China Automation Congress (CAC)(2023)
摘要
The development of Internet has led to increasingly serious network security issues. Although encryption technology applied to network communication do users the favour of protecting data privacy to a certain extent, the existence of encrypted traffic also provides a cover for malicious attacks in the network. Accurate identification of encrypted traffic is crucial for maintaining network security. However, improving classification performance for low labeled rate scenarios remains a challenge in current research. Therefore, we propose an encryption traffic classification method based on Deep Learning, named RM-Net. This method combines ResNet, MobileNet and LSTM models and fully leverages their advantages to extract data features from both spatial and temporal dimensions, while continuously learning complex relationships between features. To verify the effectiveness of proposed model, we conduct multiple experiments using real network encrypted traffic data under a 5 % labeled rate scenario, and compare them with ResNet and LSTM. The experimental results show that our method performs well in accuracy, precision, recall and Fl-score metrics, achieving an accuracy rate of 97.72 % with only 5 % labeled data.
更多查看译文
关键词
Network Security,Intelligent Network,Traffic Classification,Low labeled Rate,Depthwise Residual Module
AI 理解论文
溯源树
样例
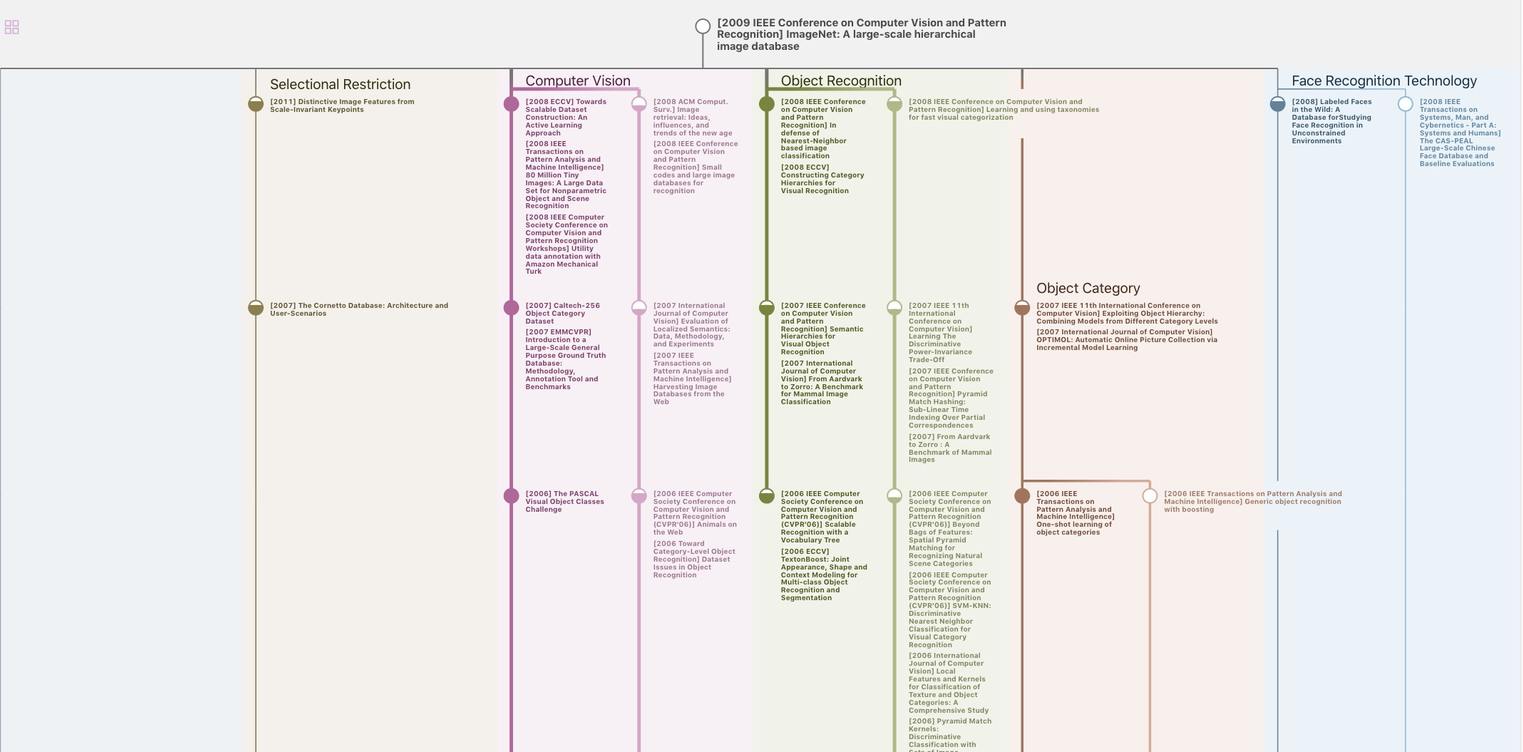
生成溯源树,研究论文发展脉络
Chat Paper
正在生成论文摘要