TDSCCNet: twin-depthwise separable convolution connect network for change detection with heterogeneous images
GEOCARTO INTERNATIONAL(2024)
摘要
The task of change detection (CD) in optical and SAR images is an ever-evolving and demanding subject within the realm of remote sensing (RS). It holds great significance to identify the target areas by using complementary information between the two. Due to the distinct imaging mechanisms employed by optical and SAR sensors, effectively and accurately identifying changing regions can be challenging. To this end, a novel heterogeneous RS images CD network (Twin-Depthwise Separable Convolution Connect Network, TDSCCNet) is proposed in this paper. Image domain transformation is a front-end task, while the back-end employs a single-branch bilayer depthwise separable convolution-connected encoder-decoder to accomplish CD work. Specifically, first, the cycle-consistent adversarial network (CycleGAN) serves to integrate the optical and SAR visual domains, and a consistent feature expression is obtained. Second, the single-branch encoder structure of bilayer depthwise separable convolution is employed to realize change feature extraction. Finally, the multiscale connected decoder reconstructed by change map is utilized to reconstruct the original images and solve the local discontinuities and holes in the binary change map. Multiscale loss is designated for optimizing the global and local effects to alleviate the class imbalance problem. It was tested on four representative datasets from Gloucester, Shuguang Village, Italy and WV-3 datasets with an overall accuracy of 97.36%, 97.01%, 97.62%, and 98.01% respectively. By comparing the existing methods, experimental results confirmed the effectiveness of the proposed method.
更多查看译文
关键词
Heterogeneous images,change detection,image domain transformation,TDSCCNet,deep learning
AI 理解论文
溯源树
样例
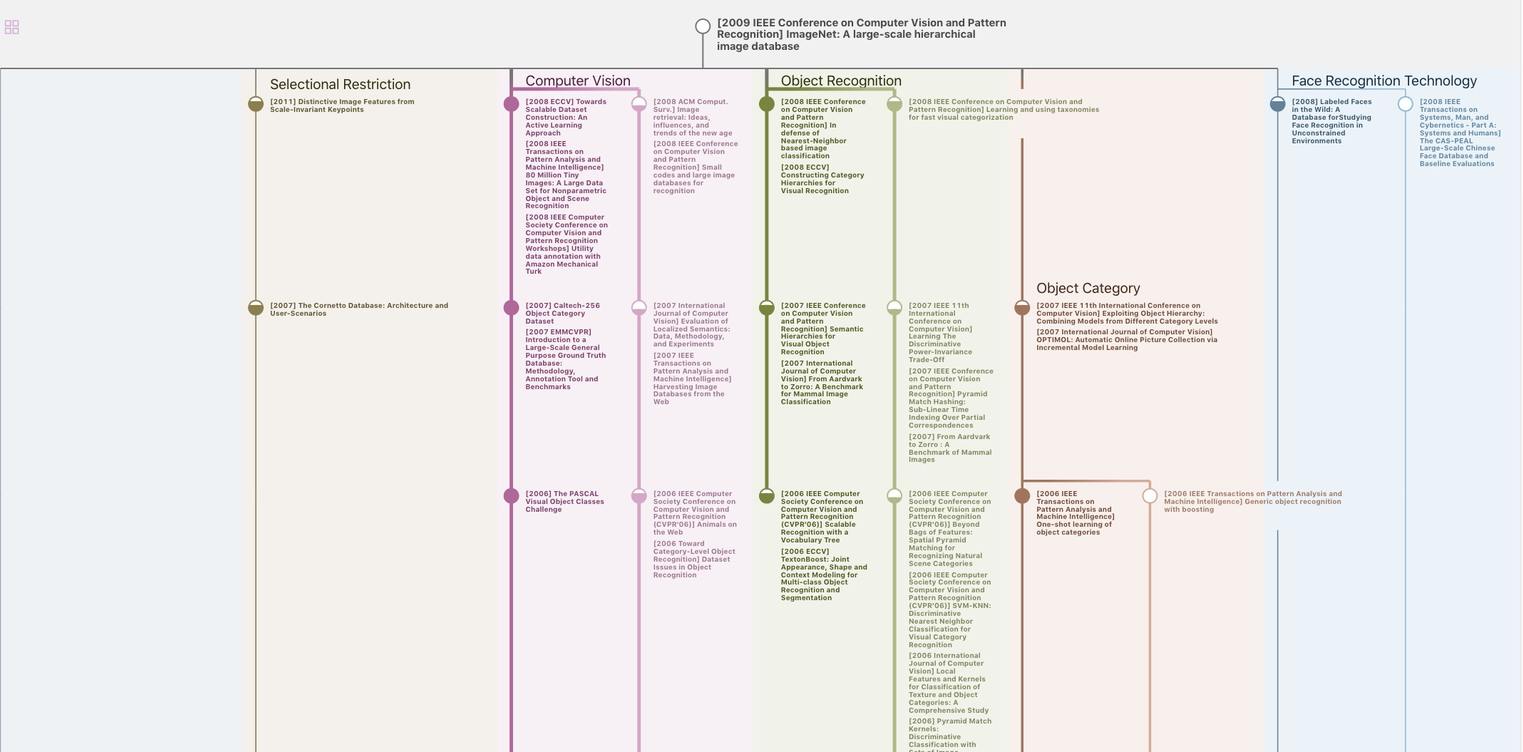
生成溯源树,研究论文发展脉络
Chat Paper
正在生成论文摘要