A reinforcement learning approach using Markov decision processes for battery energy storage control within a smart contract framework
Journal of Energy Storage(2024)
摘要
With the increasing penetration of renewable energy sources (RESs), the necessity for employing smart methods to control and manage energy has become undeniable. This study introduces a real-time energy management system based on a multi-agent system supervised by a smart contract, employing a bottom-up approach for a grid-connected DC micro-grid equipped with solar photovoltaic panels (PV), wind turbines (WT), micro-turbines (MT), and battery energy storage (BES). Each unit is controlled and managed through a distributed decision-making structure. The BES agent is governed by an intelligent structure based on a reinforcement learning model. To facilitate interaction and coordination among agents, a tendering process is employed, where each agent, under its supervised control structure, presents its offer for the tendered item at each time period. The tendering organization allocates demand using the first-price sealed-bid algorithm among bidders to optimize energy cost in the Microgrid. The proposed approach offers a real-time intelligent system capable of ensuring fault tolerance, stability, and reliability in the Microgrid. The main achievement of this study is the development of a robust real-time energy management system that integrates various renewable energy sources and battery storage while ensuring efficient operation and resilience in the face of faults or disruptions.
更多查看译文
关键词
Energy management system,Multi-agent system,Smart contract,Tendering process,First-price sealed-bid algorithm,Reinforcement learning,Markov decision process,Q-learning algorithm,Fault tolerance
AI 理解论文
溯源树
样例
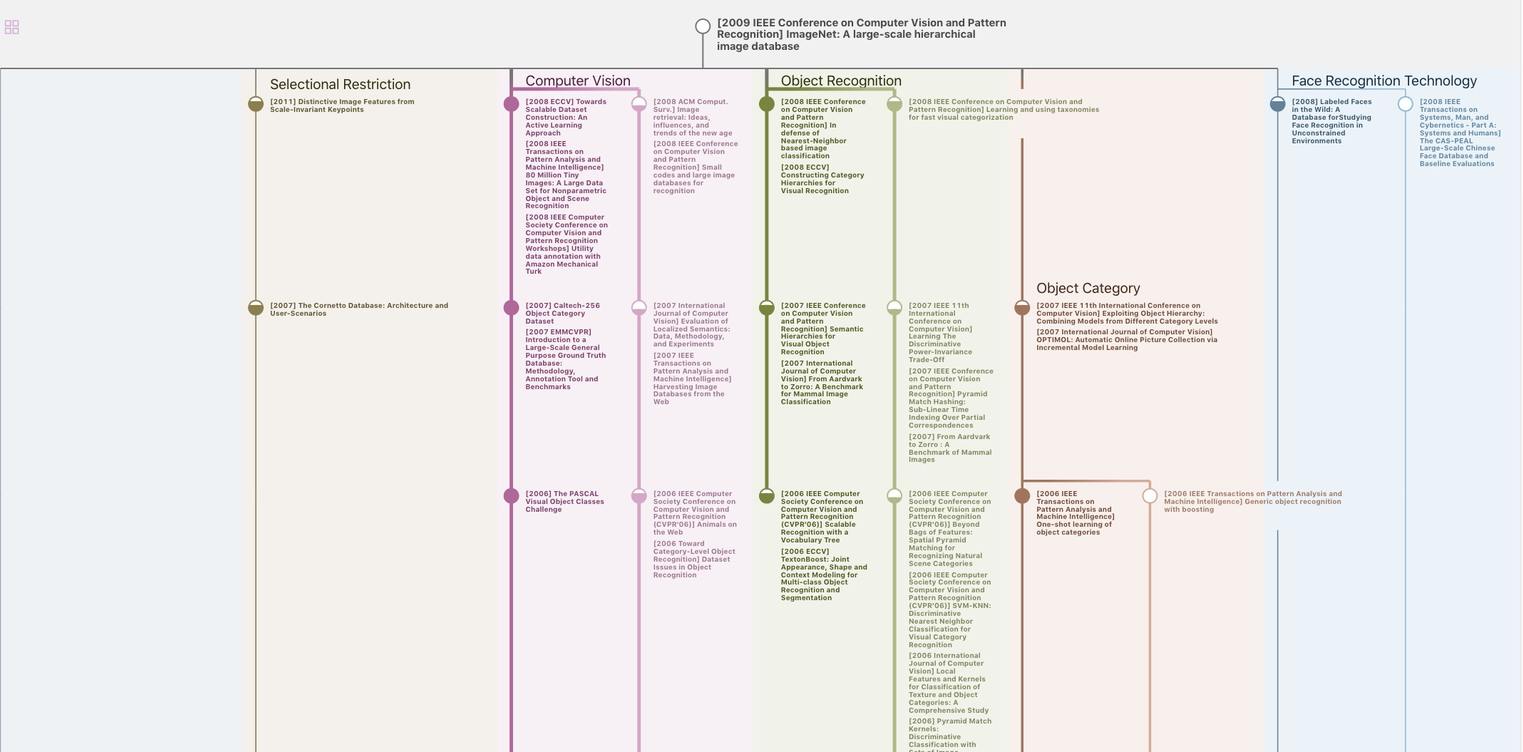
生成溯源树,研究论文发展脉络
Chat Paper
正在生成论文摘要