FDC-NeRF: Learning Pose-Free Neural Radiance Fields with Flow-Depth Consistency.
IEEE International Conference on Acoustics, Speech, and Signal Processing(2024)
摘要
Learning neural radiance fields (NeRF) without camera poses has been widely studied. However, recent methods lack explicit and effective supervision for pose estimation, resulting in ambiguous optimization of camera pose and NeRF geometry during joint training, particularly in scenarios involving large camera movements. In this paper, we propose FDCNeRF that leverages the direction information contained in the RGB-based optical flow and depth-based virtual flow as a direct guidance for camera pose optimization to reduce pose-geometry ambiguity. Additionally, we introduce Adaptive Pose-Aware Sampling (APAS) to replace the previous random ray sampling strategy, which reduces the difficulty of pose learning in early stages and preserves the diversity of rays in later stages. Experiments on the challenging Tanks and Temples dataset demonstrate that our method achieves state-of-the-art results in both novel view synthesis quality and pose estimation accuracy.
更多查看译文
关键词
Neural Radiance Fields,Pose Estimation,Novel View Synthesis,3D Reconstruction
AI 理解论文
溯源树
样例
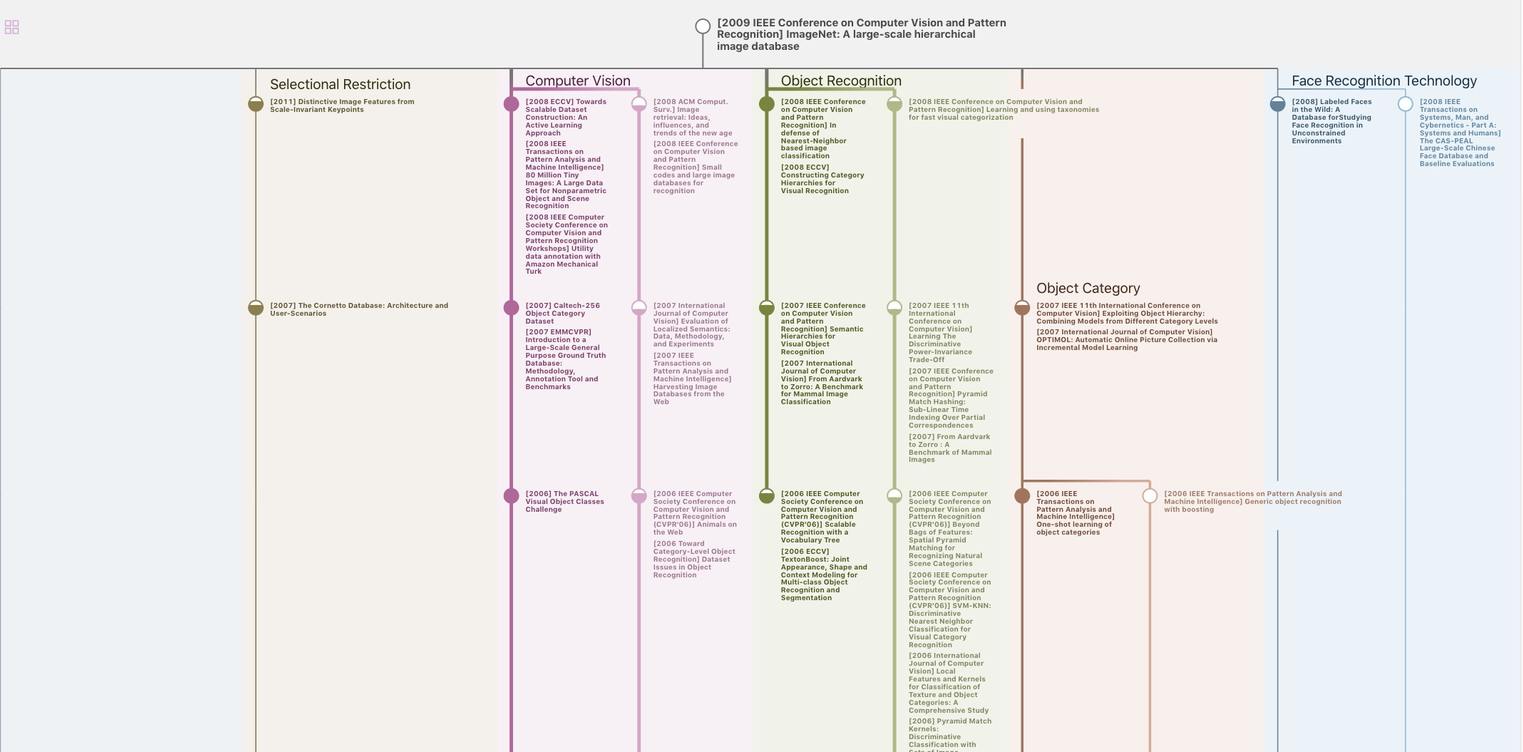
生成溯源树,研究论文发展脉络
Chat Paper
正在生成论文摘要