Proactive Caching in the Edge-Cloud Continuum with Federated Learning.
Consumer Communications and Networking Conference(2024)
摘要
In edge-cloud IoT scenarios, proactive caching strategies constitute an effective solution to optimize the use of resources while ensuring adequate Age of Information (Aol). However, the implementation of these strategies introduces significant privacy constraints, primarily stemming from the transmission of sensitive data to the cloud. To address such issue, Federated Learning (FL) has emerged as a promising approach which processes data at the edge, transmitting only the model updates to the cloud. This paper introduces CACHUUM (Cache Architecture for Cloud and Heterogeneous edge in the ContinUUM), a proactive and privacy-aware architecture designed to facilitate the deployment of various edge caching strategies within distributed edge environments. Our architecture supports three families of strategies: local, global and federated, each tailored to meet specific privacy requirements. Furthermore, our architecture is continuum-aware, accommodating different data caching locations, whether it be at the edge node, in the cloud, or somewhere in between. We demonstrate the effectiveness of CACHUUM on simulated IoT environments, by collecting metrics on forecast accuracy, caching precision and data overhead, for different strategies. The latter anticipate the optimal cache update timings for each IoT device, ensuring that Aol aligns with application requirements upon data request.
更多查看译文
关键词
Edge Caching,Federated Learning,Software Architecture,Performance Evaluation
AI 理解论文
溯源树
样例
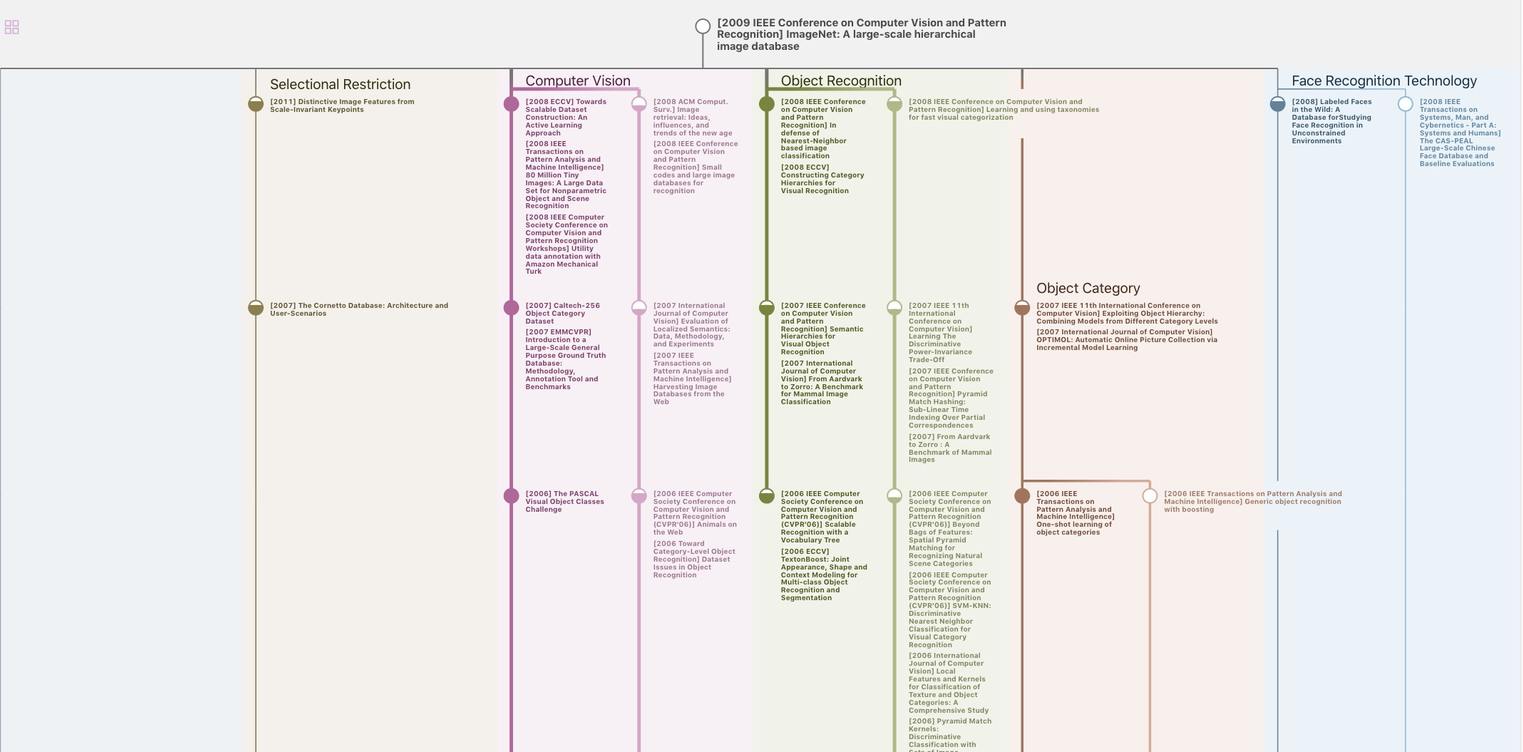
生成溯源树,研究论文发展脉络
Chat Paper
正在生成论文摘要