FedAQT: Accurate Quantized Training with Federated Learning
ICASSP 2024 - 2024 IEEE International Conference on Acoustics, Speech and Signal Processing (ICASSP)(2024)
摘要
Federated learning (FL) has been widely used to train neural networks with the decentralized training procedure where data is only accessed on clients’ devices for privacy preservation. However, the limited computation resources on clients’ devices prevent FL of large models. To overcome the constraint, one possible method is to reduce the computation memory usage with quantized neural networks such as quantization aware training on a centralized server. However, directly applying the quantization aware methods does not reduce the memory consumption on the clients’ devices of FL because the full-precision model is still used in the forward propagation of the model computation. To enable FL of the Conformer based ASR models, we propose FedAQT, an accurate quantized training framework under FL by training with quantized variables directly on clients’ devices. We empirically show that our method can achieve comparable WER with only 60% memory of the full-precision model.
更多查看译文
关键词
speech recognition,federated learning,quantization
AI 理解论文
溯源树
样例
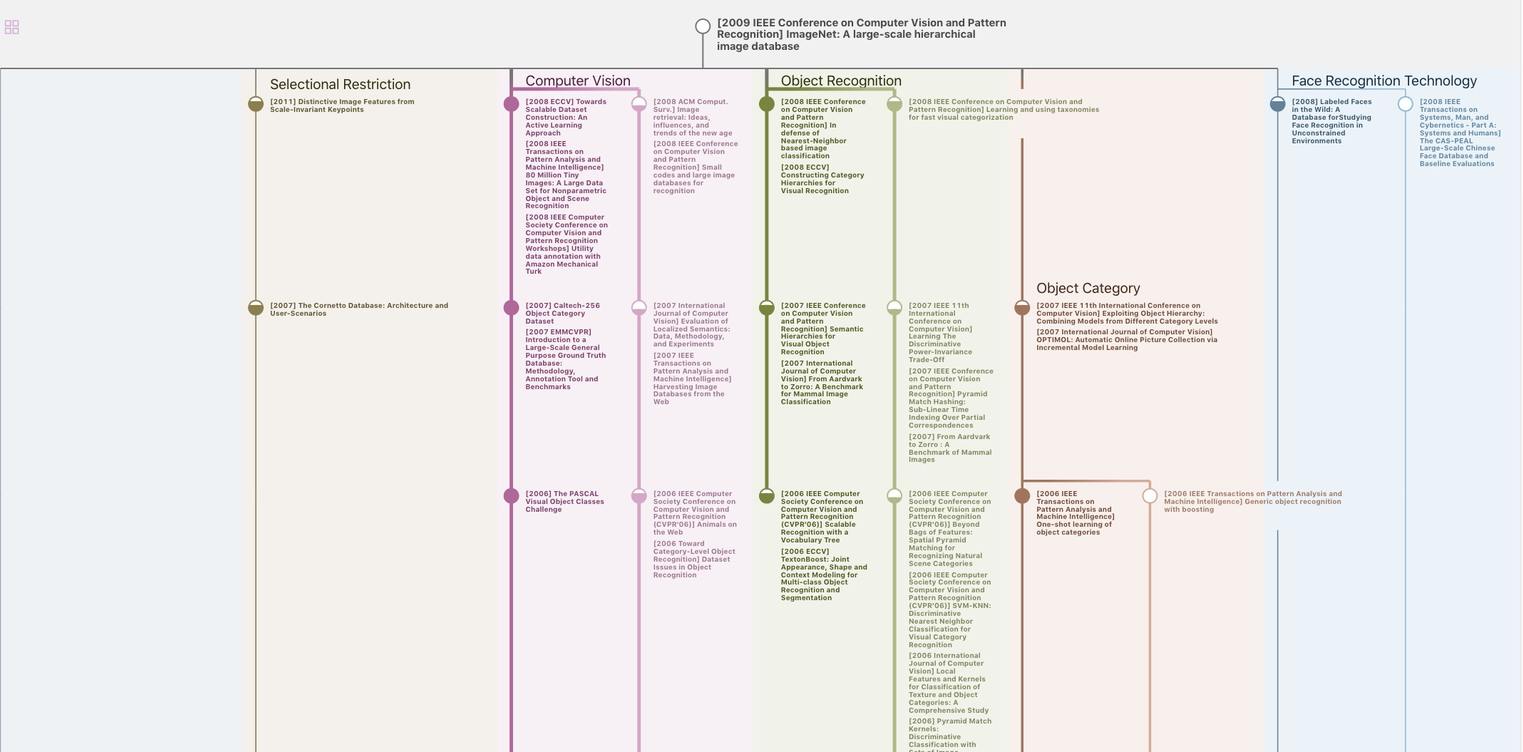
生成溯源树,研究论文发展脉络
Chat Paper
正在生成论文摘要