CDA-MBPO:Corrected Data Aggregation for Model-Based Policy Optimization
ICASSP 2024 - 2024 IEEE International Conference on Acoustics, Speech and Signal Processing (ICASSP)(2024)
摘要
Model-based reinforcement learning has shown promise in sample efficiency but suffers from errors accumulated during multi-step model sampling. To tackle this issue, we propose corrected data aggregation for model-based policy optimization. This approach involves aligning simulated trajectories with their real counterparts from random starting states and with varying sampling lengths to create paired real-simulated samples. The R-Q discriminator is incorporated to assess the quality of the simulated samples by computing the R-Q difference, modeled as a Gaussian distribution within each paired sample. We update the Q network and the dynamics model using all real samples and the simulated samples whose R-Q difference fall below a predefined threshold. The experimental results demonstrate that our method outperforms state-of-the-art model-based methods in sample efficiency and asymptotic performance across challenging tasks. Our code is available at https://github.com/duxin0618/CDA-MBPO.
更多查看译文
关键词
Model learning,Reinforcement learning,Data aggregation,Discriminator
AI 理解论文
溯源树
样例
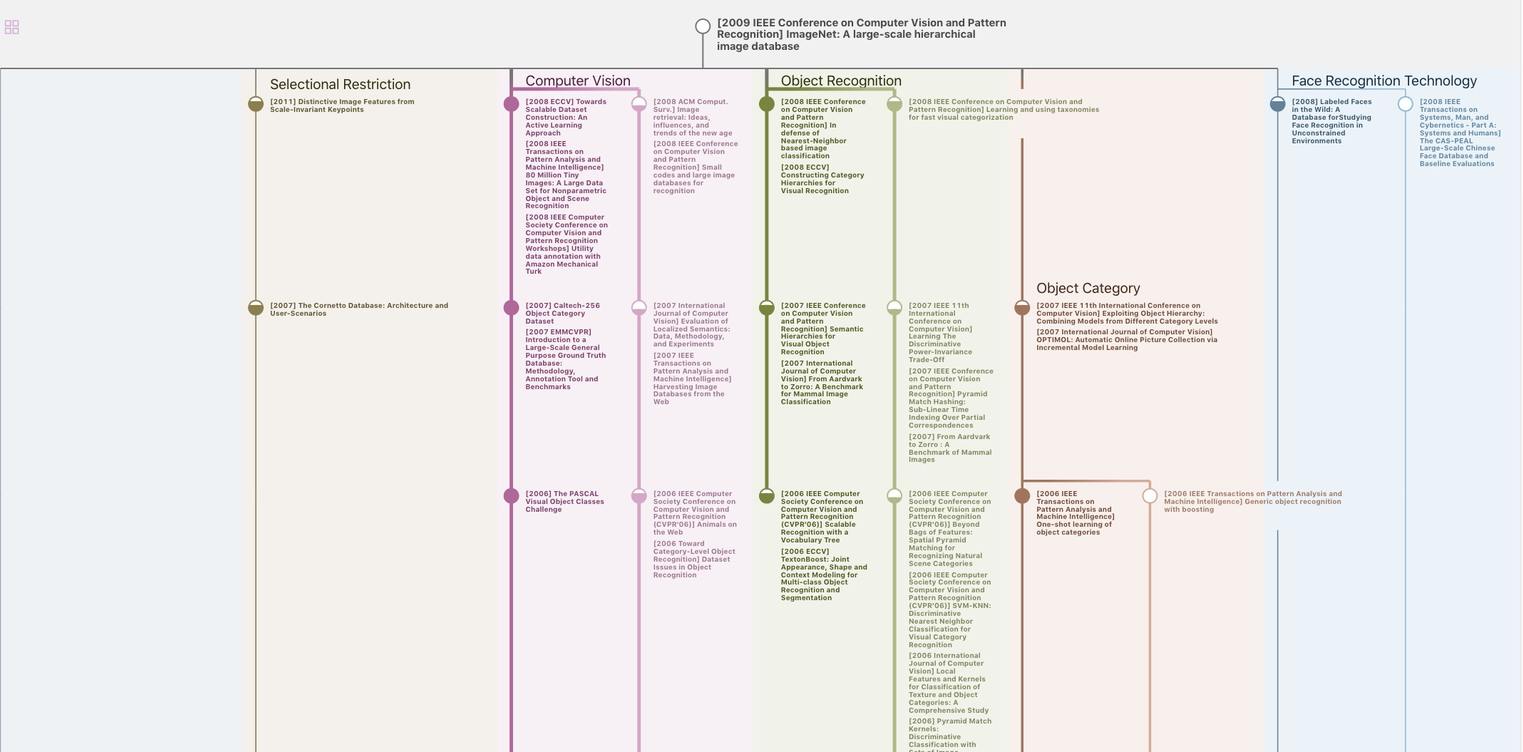
生成溯源树,研究论文发展脉络
Chat Paper
正在生成论文摘要