Weakly-Supervised Crowd Counting with Token Attention and Fusion: A Simple and Effective Baseline
ICASSP 2024 - 2024 IEEE International Conference on Acoustics, Speech and Signal Processing (ICASSP)(2024)
摘要
Conventional crowd counting methods exploit a large number of point annotations to train regression-based neural networks for density map estimation. However, laborious point annotations of human heads (strong supervision) are required in training. This paper presents a simple and effective crowd counting method with only image-level count annotations, i.e., the number of people in an image (weak supervision). Specifically, we first investigate three backbone networks and find the significance of the global information extracted by self-attention for weakly-supervised crowd counting. Then, we propose an effective network composed of a Transformer backbone and token channel attention module (T-CAM) in the counting head, where the attention in channels of tokens can compensate for the self-attention between tokens of the Transformer. Finally, a simple token fusion is proposed to obtain global information. Experimental results on two representative crowd counting benchmarks show the superiority of the proposed method, with an average 10% relative improvement compared with baselines. The code is publicly available at https://github.com/WangyiNTU/WSCC_TAF.
更多查看译文
关键词
Crowd counting,weak supervision,vision transformer,token attention,token fusion
AI 理解论文
溯源树
样例
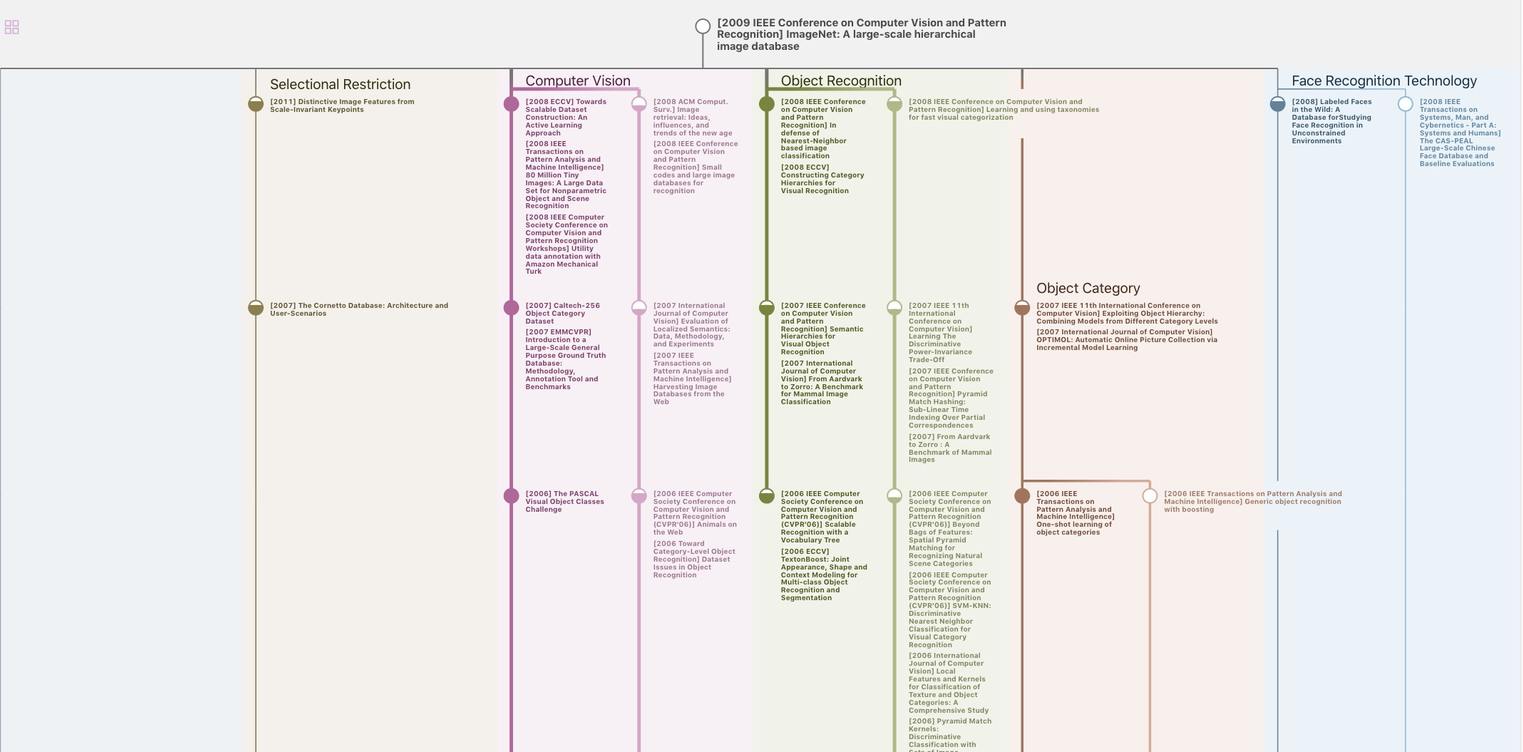
生成溯源树,研究论文发展脉络
Chat Paper
正在生成论文摘要