A New Pre-Training Paradigm for Offline Multi-Agent Reinforcement Learning with Suboptimal Data
ICASSP 2024 - 2024 IEEE International Conference on Acoustics, Speech and Signal Processing (ICASSP)(2024)
摘要
Offline multi-agent reinforcement learning (MARL) with pre-training paradigm, which uses a large quantity of trajectories for offline pre-training and online deployment, has become fashionable lately. While performing well on various tasks, conventional pre-trained decision-making models based on imitation learning typically require many expert trajectories or demonstrations, which limits the development of pre-trained policies in multi-agent case. To address this problem, we propose a new setting, where a multi-agent policy is pre-trained offline using suboptimal (non-expert) data and then tested online with the expectation of high rewards. In this practical setting inspired by contrastive learning , we propose YANHUI, a simple yet effective framework utilizing a well-designed reward contrast function for multi-agent policy representation learning from a dataset including various reward-level data instead of just expert trajectories. Furthermore, we enrich the multi-agent policy pre-training with mixture-of-experts to dynamically represent it. With the same quantity of offline StarCraft Multi-Agent Challenge datasets, YANHUI achieves significant improvements over offline MARL baselines. In particular, our method surprisingly competes in performance with earlier state-of-the-art approaches, even with 10% of the expert data used by other baselines and the rest replaced by poor data.
更多查看译文
关键词
Multi-agent system,reinforcement learning,pre-training
AI 理解论文
溯源树
样例
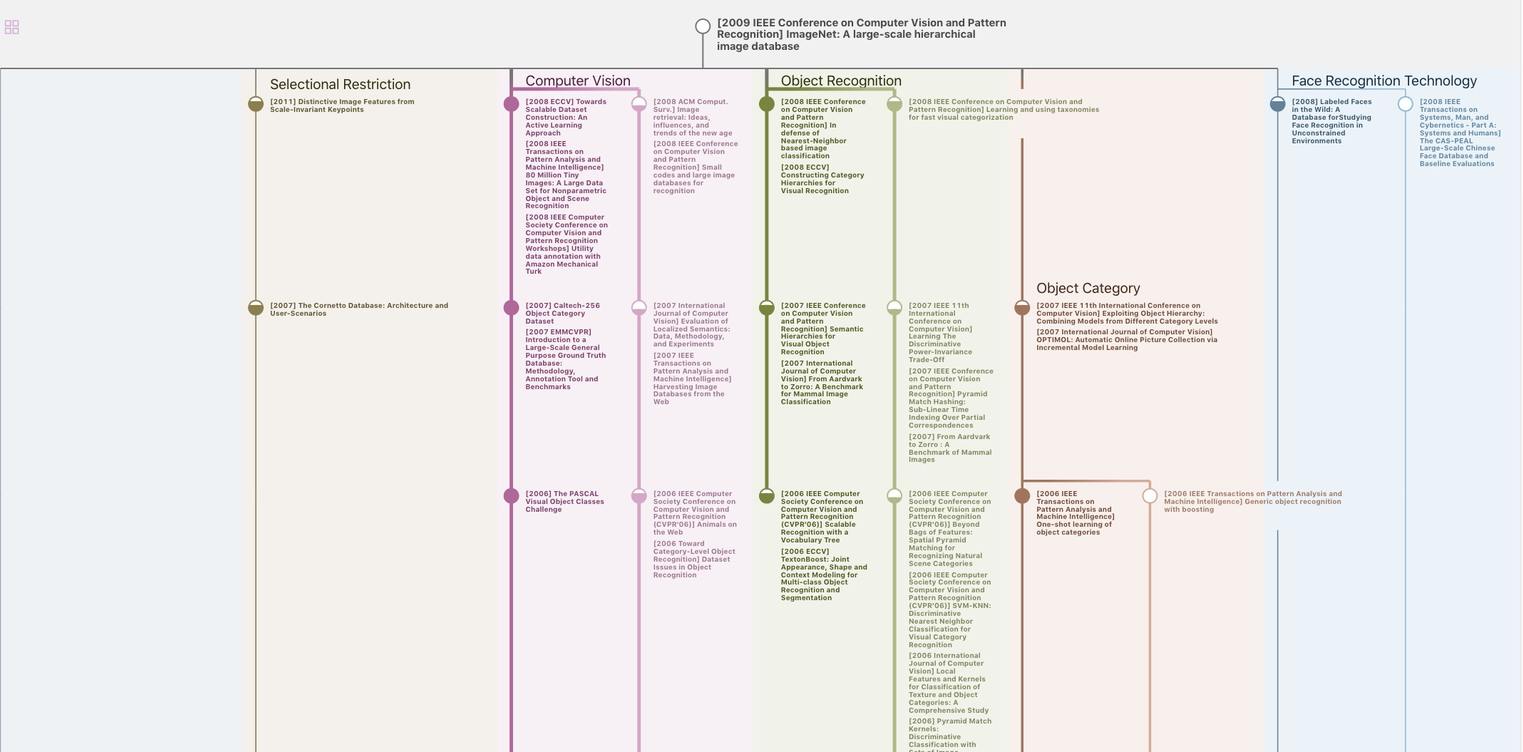
生成溯源树,研究论文发展脉络
Chat Paper
正在生成论文摘要