Balanced Learning for Multi-Domain Long-Tailed Speaker Recognition
ICASSP 2024 - 2024 IEEE International Conference on Acoustics, Speech and Signal Processing (ICASSP)(2024)
摘要
This paper considers two types of imbalance problems commonly inherent in large-scale datasets: multiple domain and class imbalance. Class imbalance causes the algorithm to be biased toward the majority classes, and multiple-domain data results in significant performance disparities for different domains. To tackle these challenges, we propose a novel learning approach for multi-domain imbalanced datasets, featuring two techniques: (i) distribution-aware partial mask and (ii) domain-wise interprototype loss function. The distribution-aware partial mask selects negative class centers based on class-level distribution and domain labels, adjusting the ratio of positive and negative updates for prototype vectors and enhancing discriminative feature learning within each domain. Additionally, the domain-wise interprototype loss enforces orthogonality among prototype vectors within each domain, leading to increased discriminativeness. We demonstrate the superiority of our approach over baselines through experiments on publicly available speaker recognition datasets, including CN-Celeb and Mozilla Common Voice.
更多查看译文
关键词
Speaker Recognition,Imbalanced Learning,Multiple-Domain Learning,Long-tailed Distribution
AI 理解论文
溯源树
样例
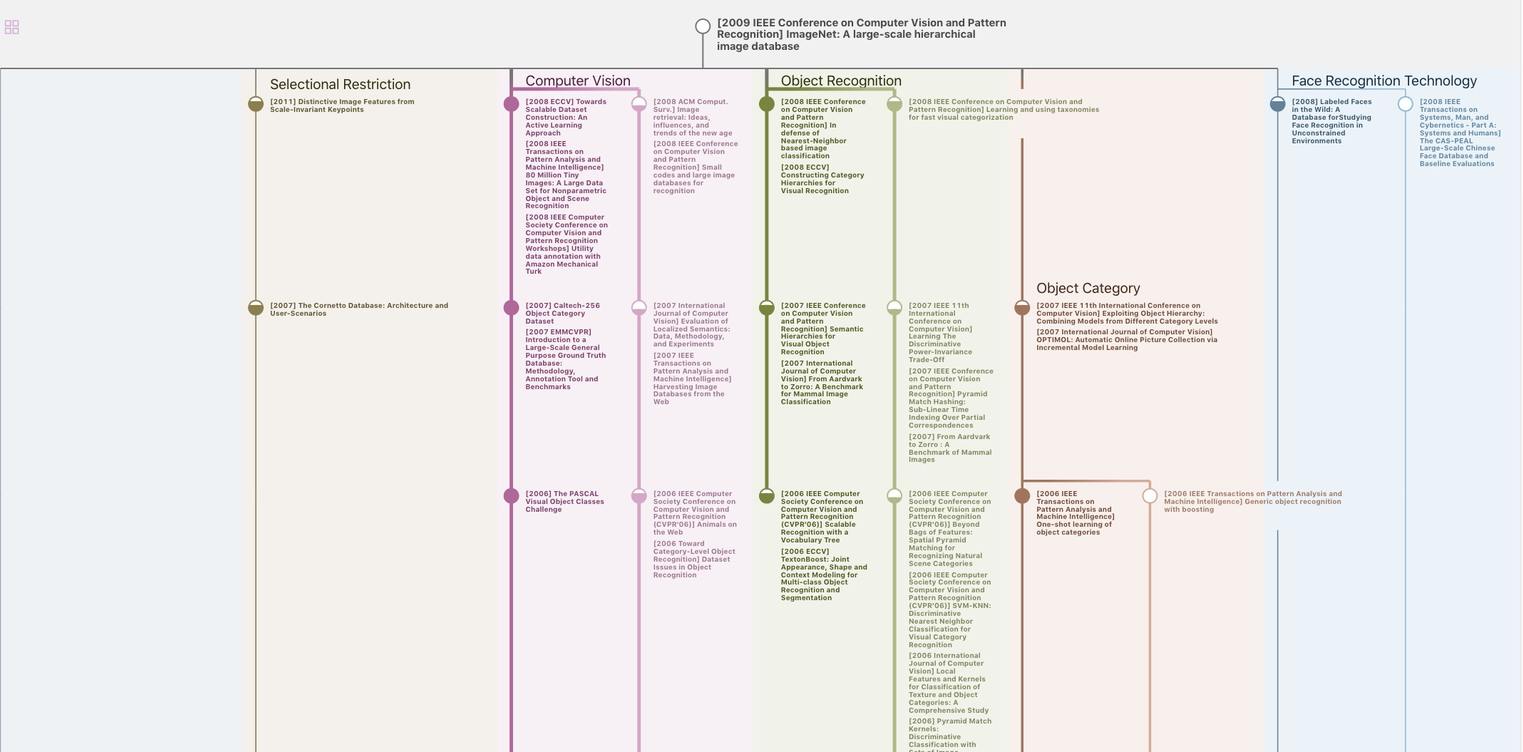
生成溯源树,研究论文发展脉络
Chat Paper
正在生成论文摘要