EPSet: Efficient and Privacy-Preserving Set Similarity Range Query over Encrypted Data
IEEE Transactions on Services Computing(2024)
摘要
Set similarity query is a fundamental query type in various applications, such as clinical diagnosis, online shopping, and mobile crowdsensing. Meanwhile, as the prevalence of outsourced query services, privacy-preserving set similarity query has been considerablely studied. However, to the best of our knowledge, most previously reported solutions suffer from applicability, efficiency, or security issues. Aiming at addressing these issues, we propose an efficient and privacy-preserving set similarity range query scheme (EPSet), where Jaccard similarity is employed as the similarity metric. Specifically, the set similarity range query is first transformed into multi-dimensional range queries by leveraging the triangle inequality of Jaccard distance. Then, a pivot-based k-d tree is designed for indexing the dataset and processing the set similarity query. After that, we design homomorphic encryption based privacy-preserving filter/refinement protocols, respectively named as PPF and PPR, to protect set similarity query privacy, and propose our EPSet scheme. The security of our scheme is proved under the simulation-based real/ideal model, and the performance is validated thorugh the extensive experiment evaluation.
更多查看译文
关键词
Set Similarity Range Query,Jaccard Similarity,Cloud Computing,k-d Tree,Triangle Inequality
AI 理解论文
溯源树
样例
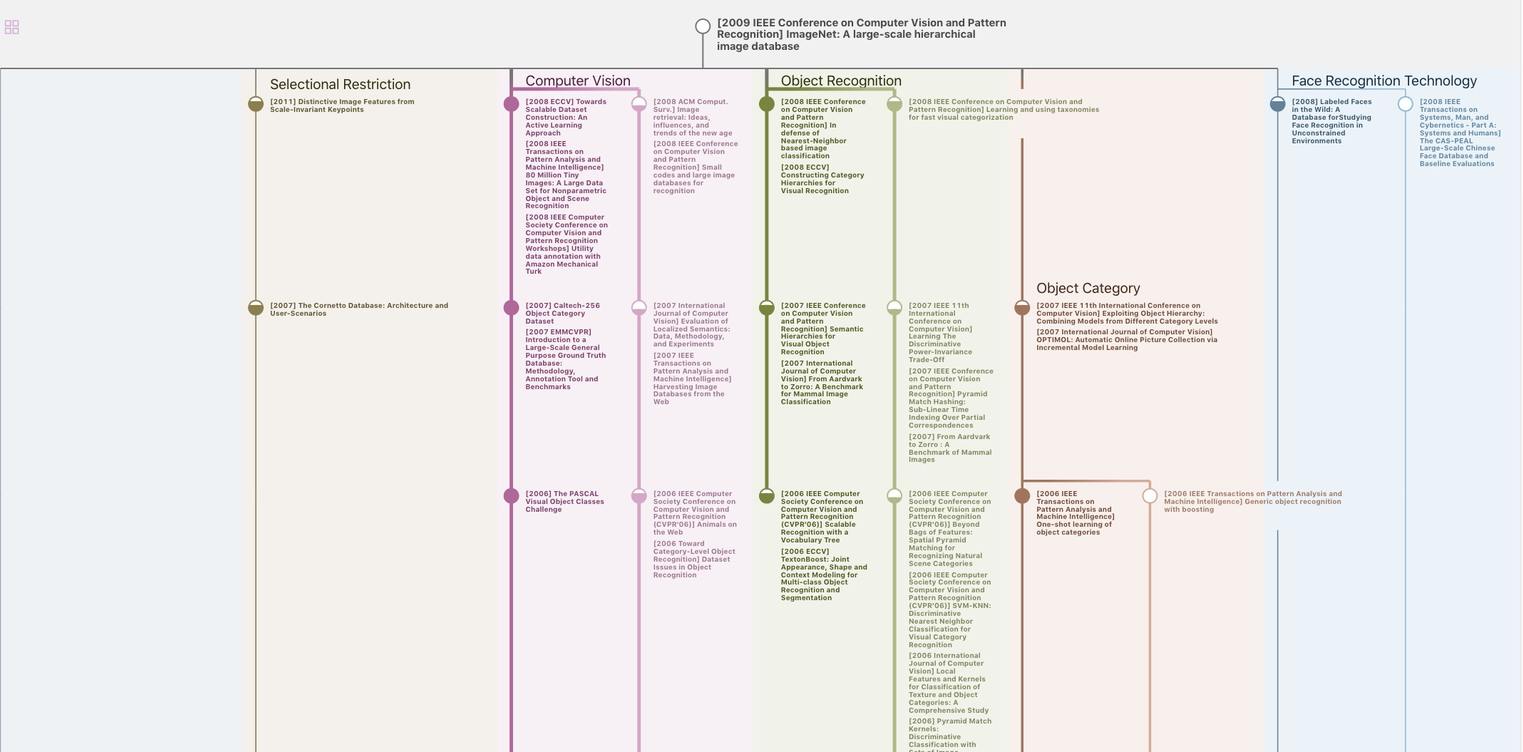
生成溯源树,研究论文发展脉络
Chat Paper
正在生成论文摘要