Large-Scale Multi-View Multiple Clustering
ICASSP 2024 - 2024 IEEE International Conference on Acoustics, Speech and Signal Processing (ICASSP)(2024)
摘要
Multi-view multiple clustering generates multiple clustering results to uncover diverse information in multi-view data, but existing solutions for this task are inefficient when processing large-scale data, and it is difficult to achieve high-quality and diverse clustering simultaneously. Therefore, we propose the Large-Scale Multi-View Multiple Clustering (LSMVMC) algorithm. Inspired by anchor graph techniques, we construct a relationship matrix using representative anchors in the neighborhood structure of the original samples, improving clustering speed without compromising quality. Deep matrix factorization decomposes the anchor graph into multiple subspaces through a multi-layer decomposition matrix. By assigning different weights to views, comprehensive consideration of view-specific information enables the generation of high-quality clusters in each subspace. We enhance cluster diversity by employing orthogonal subspaces to comprehensively consider redundancy. Experimental results on benchmark datasets demonstrate that our solution is generally more efficient and performs better than other large-scale multi-view data processing methods.
更多查看译文
关键词
multi-view multiple clustering,largescale data,anchor graph,clustering diversity
AI 理解论文
溯源树
样例
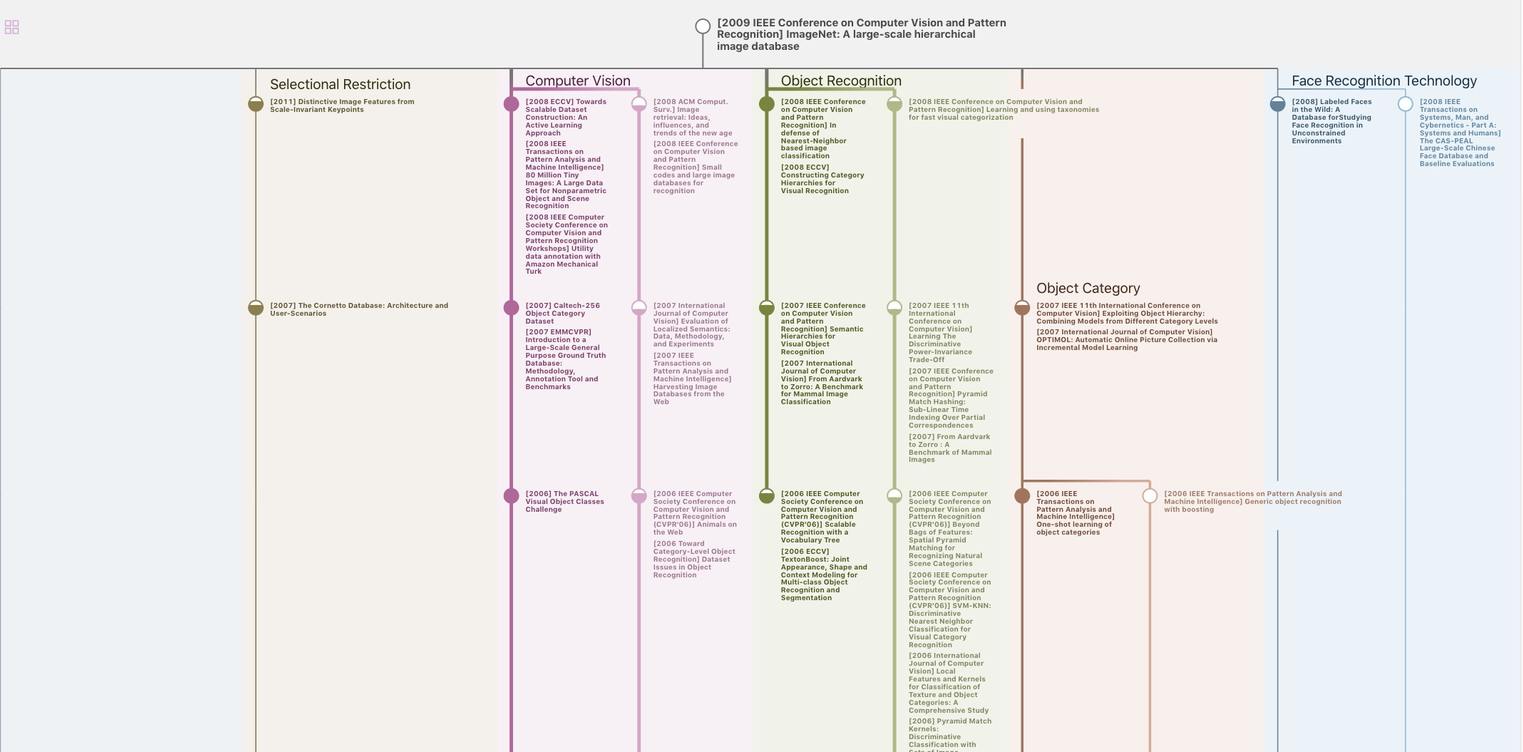
生成溯源树,研究论文发展脉络
Chat Paper
正在生成论文摘要