Representation Learning across Feature and Topology Views with Output Correction for Graph Convolutional Networks
ICASSP 2024 - 2024 IEEE International Conference on Acoustics, Speech and Signal Processing (ICASSP)(2024)
摘要
In Graph Convolutional Networks (GCNs), the aggregation of node features in graph convolutional learning is typically guided solely by the topology of the graphs. However, both network topology and node features provide unique and valuable information. Relying solely on topology cannot yield entirely accurate and comprehensive neighborhood information. This paper proposes Output Correction for GCNs (OC-GCN), which advocates for representation learning incorporating feature and topology views. Specifically, we employ two GCN encoders to extract node embeddings in both the feature and topology spaces. We first identify consistent and inconsistent nodes by comparing the pseudo-labels generated by the encoders. Subsequently, we regenerate representations of inconsistent nodes by aggregating the representations of consistent nodes within their respective neighborhoods. Our experiments demonstrate that OC-GCN significantly enhances the classification accuracy of inconsistent nodes. We conducted extensive experiments on benchmark datasets and observed that OC-GCN outperforms state-of-the-art baselines across various label rates.
更多查看译文
关键词
Graph convolutional networks,Pseudo-labels,Output correction,Semi-supervised classification
AI 理解论文
溯源树
样例
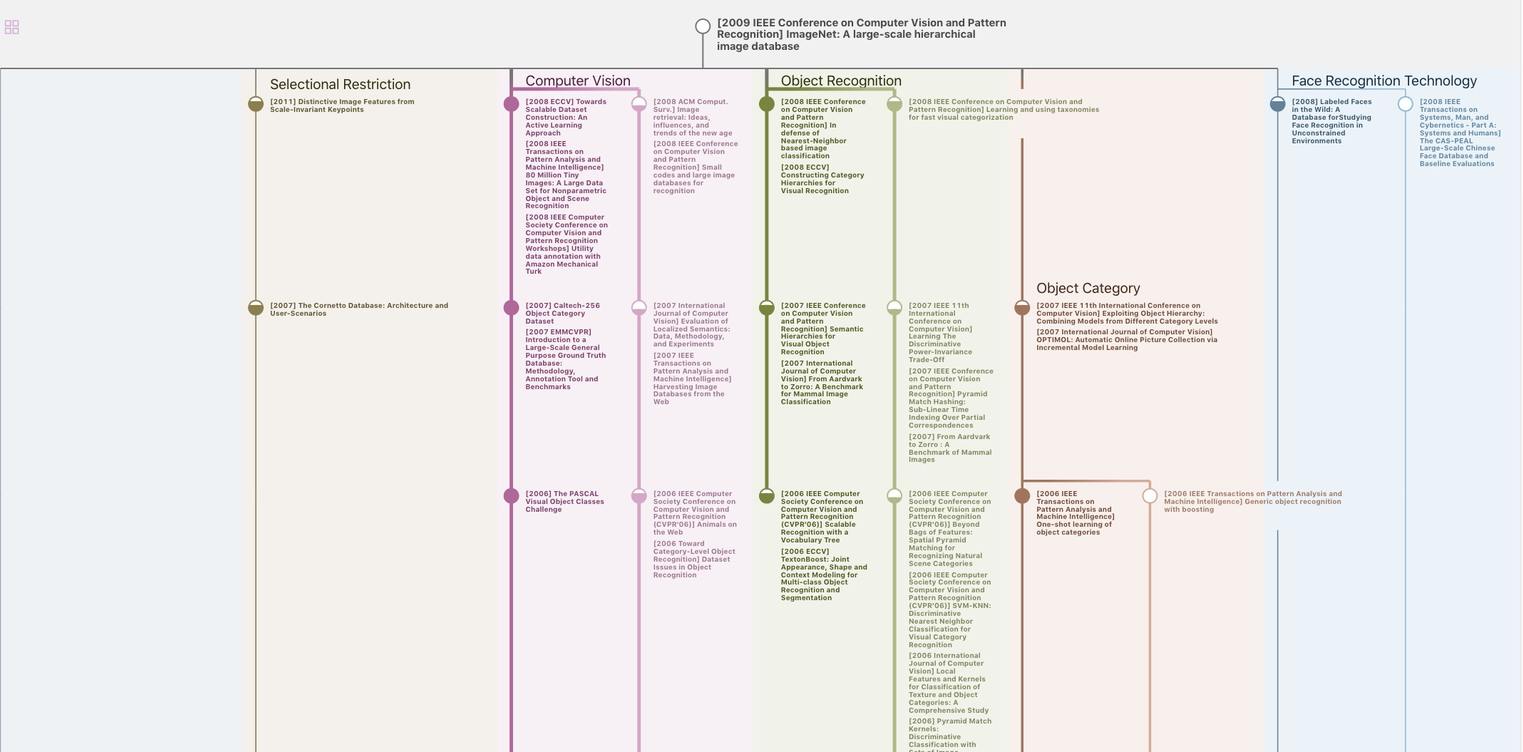
生成溯源树,研究论文发展脉络
Chat Paper
正在生成论文摘要