3DAttGAN: A 3D Attention-Based Generative Adversarial Network for Joint Space-Time Video Super-Resolution
IEEE Transactions on Emerging Topics in Computational Intelligence(2024)
摘要
Joint space-time video super-resolution aims to increase both the spatial resolution and the frame rate of a video sequence. As a result, details become more apparent, leading to a better and more realistic viewing experience. This is particularly valuable for applications such as video streaming, video surveillance (object recognition and tracking), and digital entertainment. Over the last few years, several joint space-time video super-resolution methods have been proposed. While those built on deep learning have shown great potential, their performance still falls short. One major reason is that they heavily rely on two-dimensional (2D) convolutional networks, which restricts their capacity to effectively exploit spatio-temporal information. To address this limitation, we propose a novel generative adversarial network for joint space-time video super-resolution. The novelty of our network is twofold. First, we propose a three-dimensional (3D) attention mechanism instead of traditional two-dimensional attention mechanisms. Our generator uses 3D convolutions associated with the proposed 3D attention mechanism to process temporal and spatial information simultaneously and focus on the most important channel and spatial features. Second, we design two discriminator strategies to enhance the performance of the generator. The discriminative network uses a two-branch structure to handle the intra-frame texture details and inter-frame motion occlusions in parallel, making the generated results more accurate. Experimental results on the Vid4, Vimeo-90 K, and REDS datasets demonstrate the effectiveness of the proposed method.
更多查看译文
关键词
Video space-time super-resolution,generative adversarial network,3D attention mechanism,quality enhancement
AI 理解论文
溯源树
样例
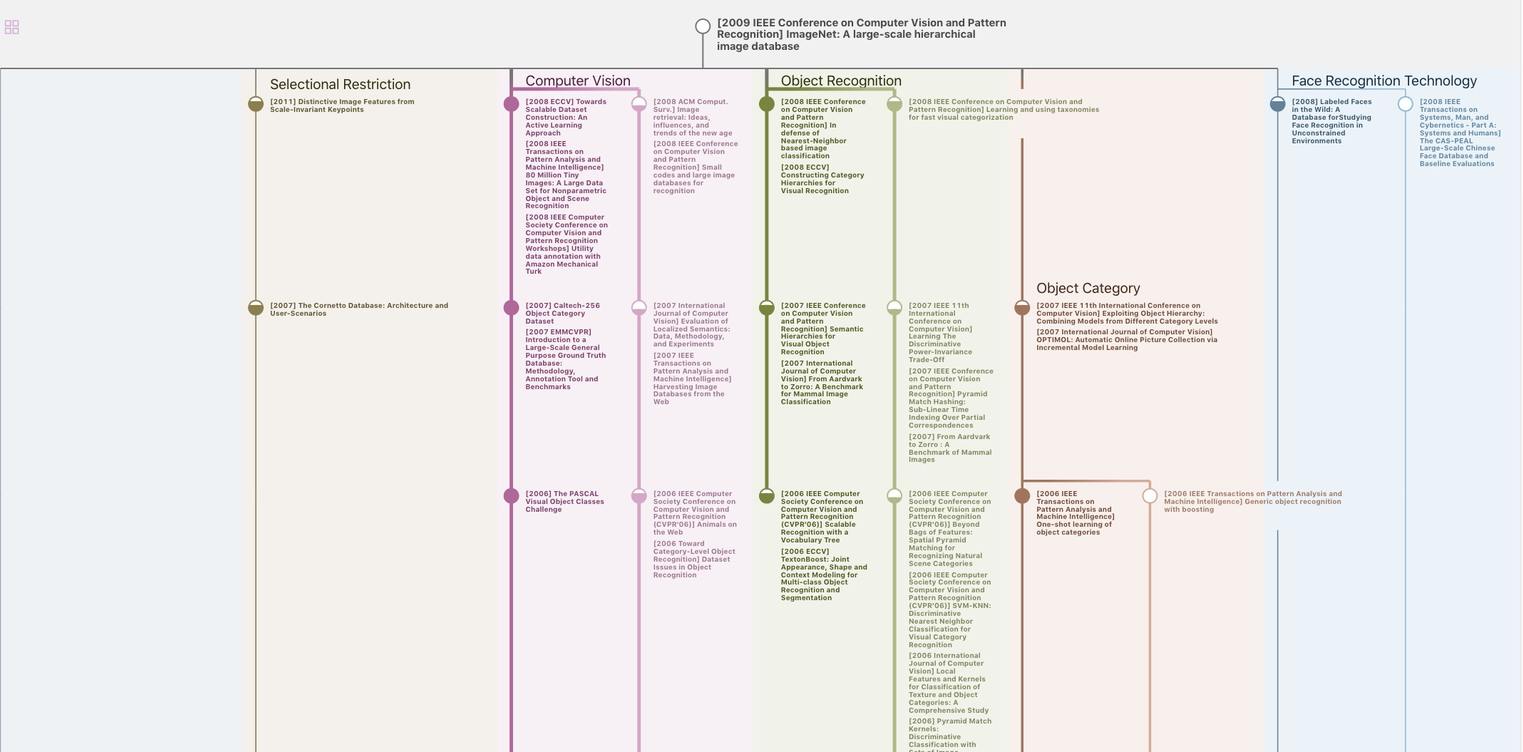
生成溯源树,研究论文发展脉络
Chat Paper
正在生成论文摘要