Frilled Lizard Optimization: A Novel Nature-Inspired Metaheuristic Algorithm for Solving Optimization Problems
crossref(2024)
摘要
This article introduces a novel nature-inspired metaheuristic algorithm called Frilled Lizard Optimization (FLO), which emulates the hunting behavior of frilled lizards in their natural habitat. FLO draws in-spiration from the sit-and-wait strategy observed in frilled lizards during hunting. The underlying theory of FLO is presented and mathematically formulated in two phases: (i) an exploration phase, simulating the frilled lizard's attack towards prey, and (ii) an exploitation phase, simulating the lizard's retreat to the top of the tree after feeding. To assess FLO's efficacy in solving optimization problems, the algorithm's performance is evaluated across fifty-two standard benchmark functions, encompassing unimodal, high-dimensional multimodal, fixed-dimensional multimodal, and the CEC 2017 test suite. Comparative analyses with twelve existing metaheuristic algorithms are conducted. The simulation results reveal that FLO, distinguished by its adeptness in exploration, exploitation, and balancing them during search process, outperforms competing algorithms. Additionally, FLO is implemented on twenty-two constrained optimization problems from the CEC 2011 test suite and four engineering design problems, demonstrating its effectiveness in addressing real-world optimization applications.
更多查看译文
AI 理解论文
溯源树
样例
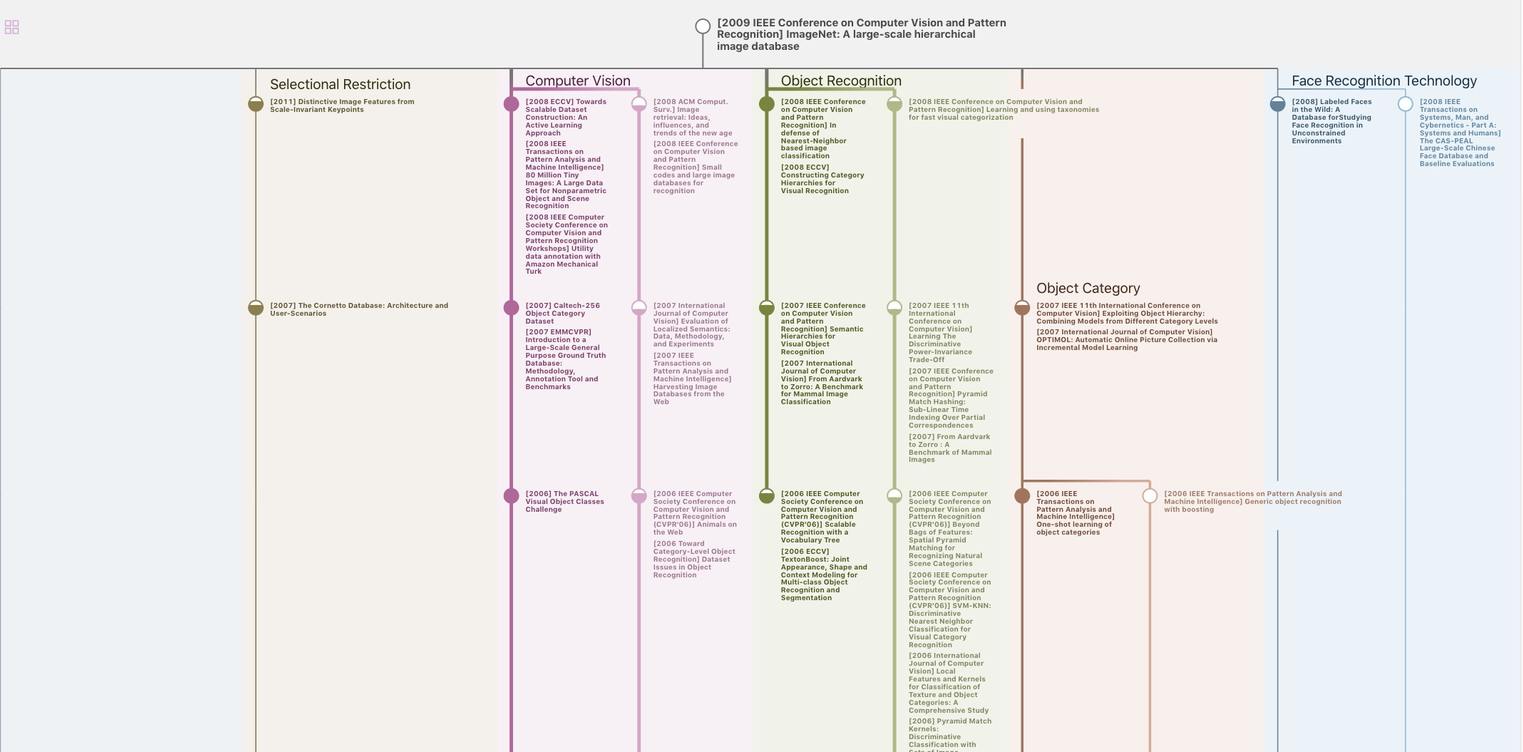
生成溯源树,研究论文发展脉络
Chat Paper
正在生成论文摘要