Distill Vision Transformers to CNNs via Teacher Collaboration
ICASSP 2024 - 2024 IEEE International Conference on Acoustics, Speech and Signal Processing (ICASSP)(2024)
摘要
The vision transformer (ViT) has recently emerged as a leading approach in various domains, outperforming other methods. Therefore, it is logical to explore the possibility of transferring the superior knowledge from ViT to more compact and cost-effective convolutional neural networks (CNNs). However, due to substantial architectural disparities in representation and logits between these models, conventional knowledge distillation methods have proven ineffective in this context. To address this issue, a novel cross-architecture knowledge distillation scheme based on teacher collaboration is proposed to alleviate the architecture gap. Two different teachers, i.e. one ViT and one CNN, are utilized to simultaneously distill the student by feature reaggregation and logit correction. The experiments show that the proposed scheme outperforms conventional methods on CIFAR-100 dataset. The code is available at https://github.com/SunkiLin/RCD.
更多查看译文
关键词
Knowledge Distillation,Vision Transformer,Convolutional Neural Network,Cross Architecture
AI 理解论文
溯源树
样例
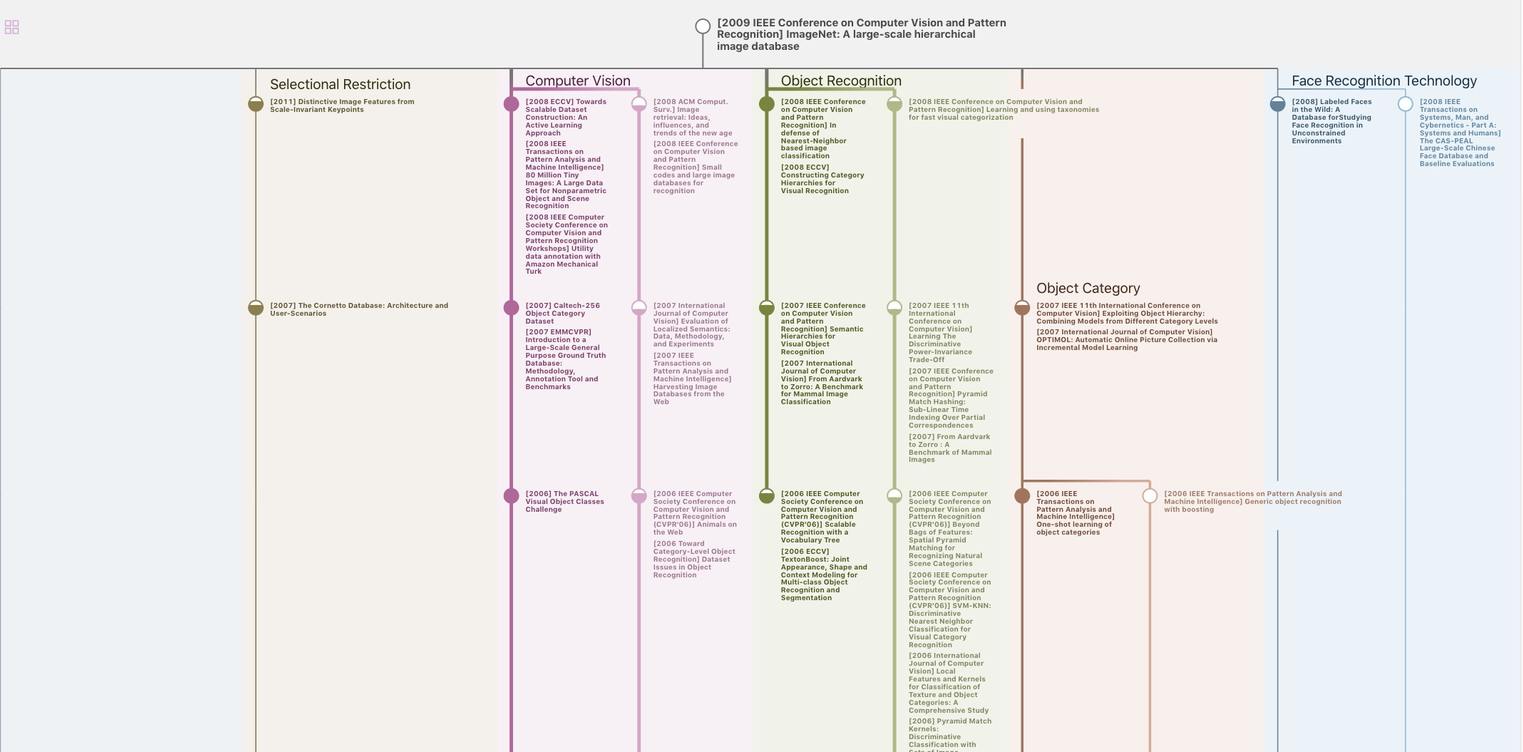
生成溯源树,研究论文发展脉络
Chat Paper
正在生成论文摘要