Enhancing generalized spectral clustering with embedding Laplacian graph regularization
CAAI TRANSACTIONS ON INTELLIGENCE TECHNOLOGY(2024)
摘要
An enhanced generalised spectral clustering framework that addresses the limitations of existing methods by incorporating the Laplacian graph and group effect into a regularisation term is presented. By doing so, the framework significantly enhances discrimination power and proves highly effective in handling noisy data. Its versatility enables its application to various clustering problems, making it a valuable contribution to unsupervised learning tasks. To optimise the proposed model, the authors have developed an efficient algorithm that utilises the standard Sylvester equation to compute the coefficient matrix. By setting the derivatives to zero, computational efficiency is maintained without compromising accuracy. Additionally, the authors have introduced smoothing strategies to make the non-convex and non-smooth terms differentiable. This enables the use of an alternative iteration re-weighted procedure (AIwRP), which distinguishes itself from other first-order optimisation algorithms by introducing auxiliary variables. The authors provide a provable convergence analysis of AIwRP based on the iteration procedures of unconstrained problems to support its effectiveness. Extensive numerical tests have been conducted on synthetic and benchmark databases to validate the superiority of their approaches. The results demonstrate improved clustering performance and computational efficiency compared to several existing spectral clustering methods, further reinforcing the advantages of their proposed framework. The source code is available at .
更多查看译文
关键词
clustering,graph theory,optimisation
AI 理解论文
溯源树
样例
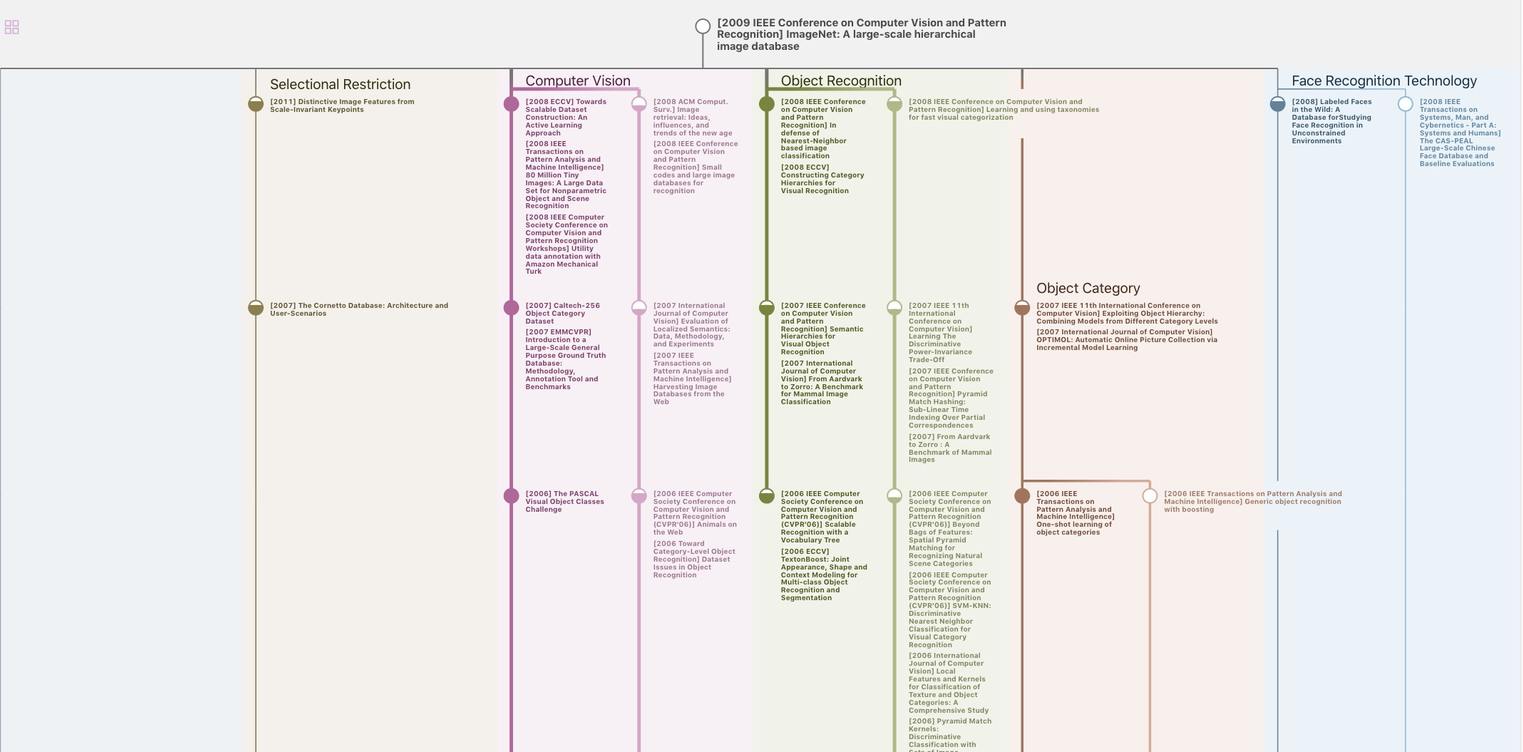
生成溯源树,研究论文发展脉络
Chat Paper
正在生成论文摘要