DI-MVS: Learning Efficient Multi-View Stereo With Depth-Aware Iterations
ICASSP 2024 - 2024 IEEE International Conference on Acoustics, Speech and Signal Processing (ICASSP)(2024)
摘要
Learning-based Multi-View Stereo (MVS) methods aim to reconstruct 3D scenes from a set of 2D calibrated images. However, existing learning-based MVS methods often overlook depth maps that include the geometric shapes of the scene when constructing the cost volume. This can result in suboptimal reconstructions, particularly in low-texture or repetitive-texture regions where valuable geometric information is absent. To address this issue, we develop DI-MVS, a coarse-to-fine framework that effectively incorporates context-guided depth geometry into the cost volume using a depth-aware iterator. First, we employ the proposed depth-aware cost completion module to update the cost volume, followed by 2D ConvGRUs to iteratively optimize depth maps efficiently. Second, we propose a hybrid loss strategy that combines two loss functions’ strengths to improve depth estimation’s robustness. Extensive experiments demonstrate that DI-MVS outperforms state-of-the-art methods on the DTU dataset and the Tanks & Temples benchmark. The source code is available at: https://github.com/JianfeiJ/DI-MVS.
更多查看译文
关键词
Multi-view Stereo,3D Reconstruction,Depth Estimation,Cost Volume
AI 理解论文
溯源树
样例
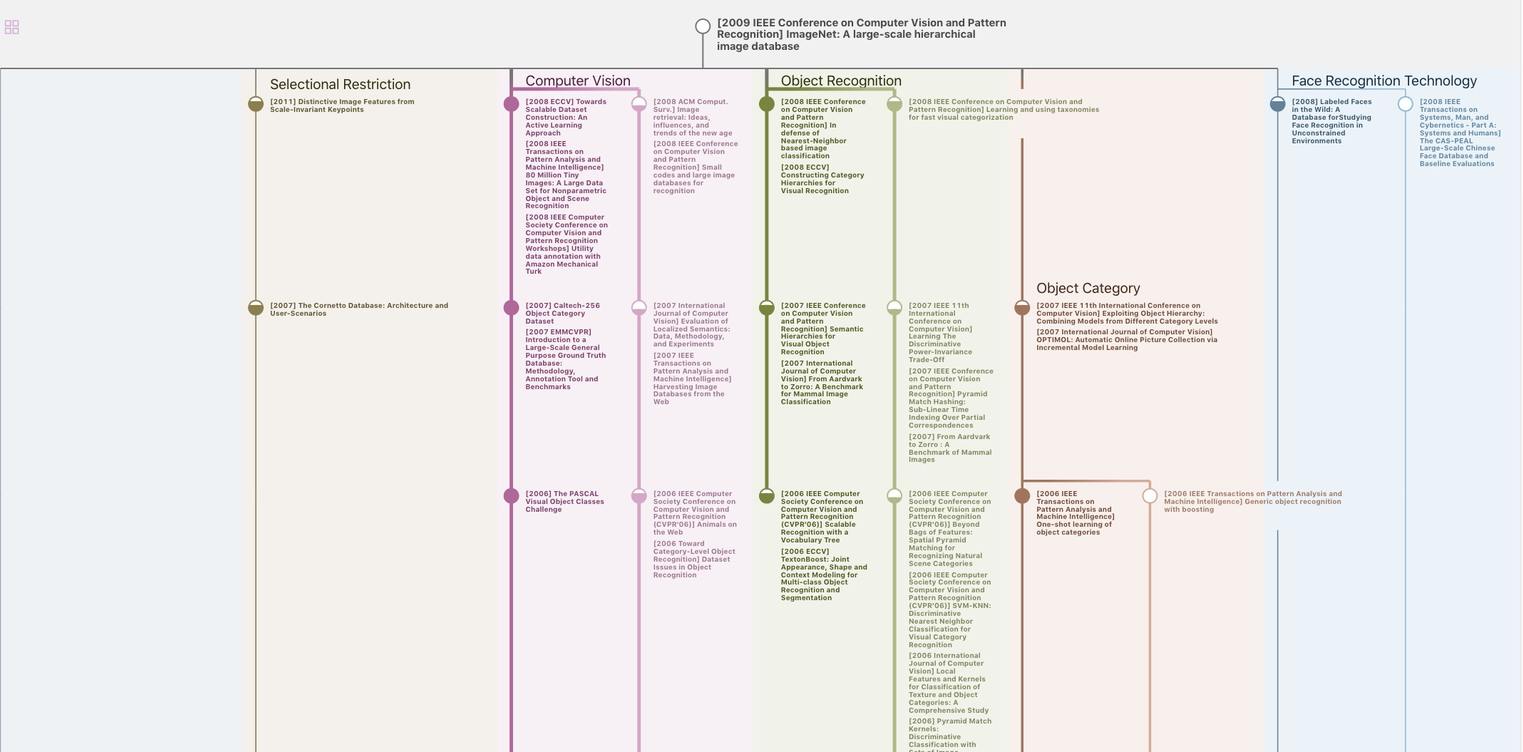
生成溯源树,研究论文发展脉络
Chat Paper
正在生成论文摘要