Predicting Fall Events by a Spatio-Temporal Topological Network with Multiple Wearable Sensors
ICASSP 2024 - 2024 IEEE International Conference on Acoustics, Speech and Signal Processing (ICASSP)(2024)
摘要
A key challenge in sensor-based fall prediction is the fact that a fall event can often occur in various configurations of fall poses together with their own spatio-temporal dependencies. This leads us to define a spatio-temporal model to explicitly characterize these internal configurations of poses. In particular, we introduce a graph neural network with spatio-temporal topological structure to encode such latent relations among poses by capturing representative patterns in fall events. Moreover, a human body orientation estimator is devised to capture human low limbs information, and as a result, separate pose dependencies are globally consistent. Empirical evaluations on two benchmark datasets and one in-house dataset suggest our approach significantly outperforms the state-of-the-art methods.
更多查看译文
关键词
Fall prediction,Spatio-temporal dependency,Inertial sensors,Graph neural network
AI 理解论文
溯源树
样例
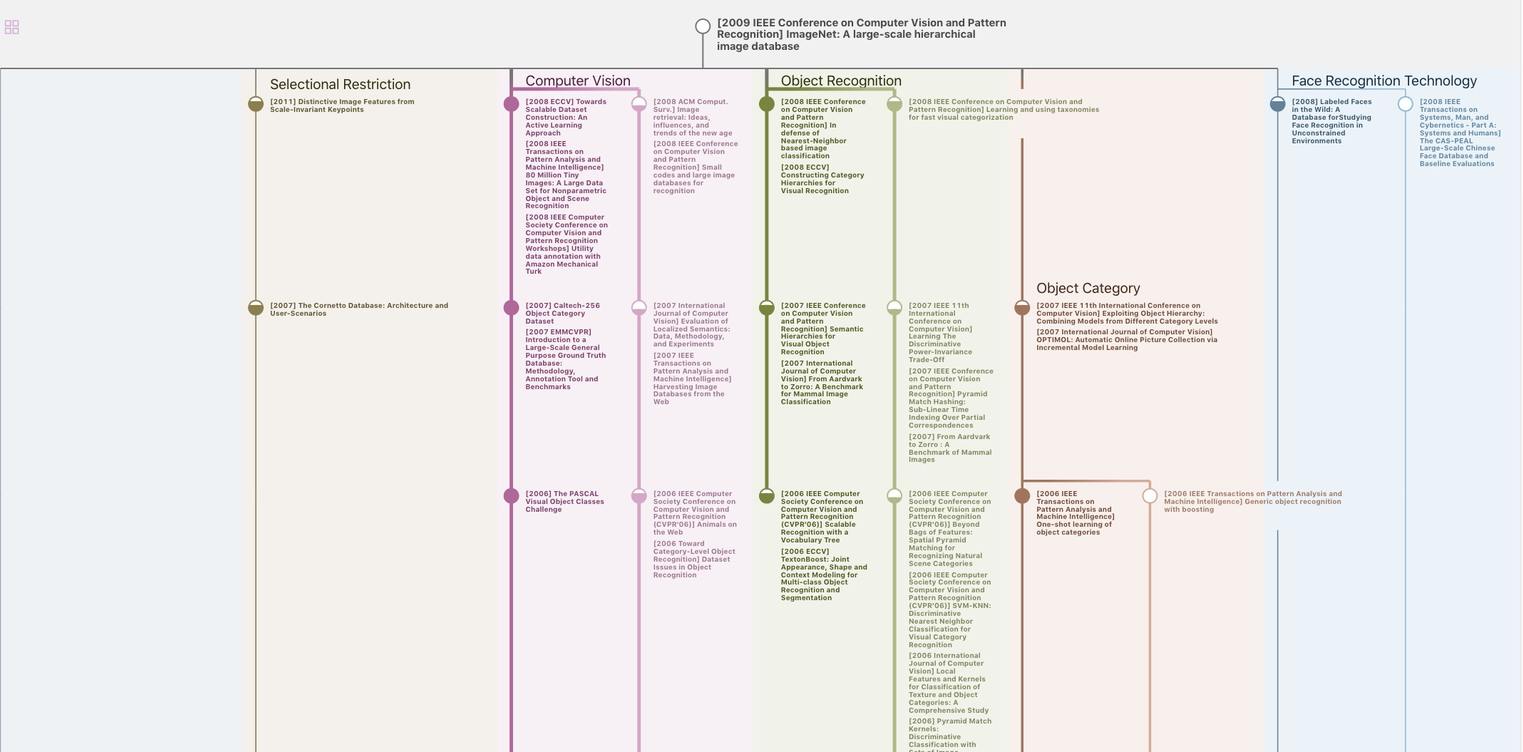
生成溯源树,研究论文发展脉络
Chat Paper
正在生成论文摘要