A feature correlation reinforce clustering and evolutionary algorithm for the green bike-sharing reposition problem
Computers & Operations Research(2024)
摘要
This paper tackles the green bike-sharing reposition problem (GBSRP) considering the reposition quality and carbon emission during the reposition simultaneously. With the expanding scale of today’s bike-sharing system (BSS), the environmental pollution during the reposition becomes obvious. GBSRP aims to satisfy the inventory request of every bike-sharing station through an identified route set while minimizing the routing fuel consumption and relevant carbon emission. This paper provides an effective feature correlation mechanism for both the clustering strategy and algorithm design, which aims at solving large-scale reposition. The original problem is clustered through the feature correlation reinforce clustering (FCRC) strategy. Then, a multi-objective evolutionary algorithm based on decomposition combined with feature correlation mechanism (FC-MOEA/D) is designed considering both the carbon emission and reposition quality. Finally, the carbon emissions of each module of the Comprehensive Modal Emissions Modeling under different reposition qualities are analyzed. The Pareto front of each instance is displayed and compared based on different clustering strategies and algorithms. The results demonstrate the competitiveness of the FCRC strategy and FC-MOEA/D algorithm. We also further discuss the relevant strategies for different reposition quality preferences and how they minimize the carbon emission during the reposition for BSS managers reference.
更多查看译文
关键词
Routing,Bike-sharing reposition,Carbon emission,Multi-objective optimization,Feature correlation reinforce clustering
AI 理解论文
溯源树
样例
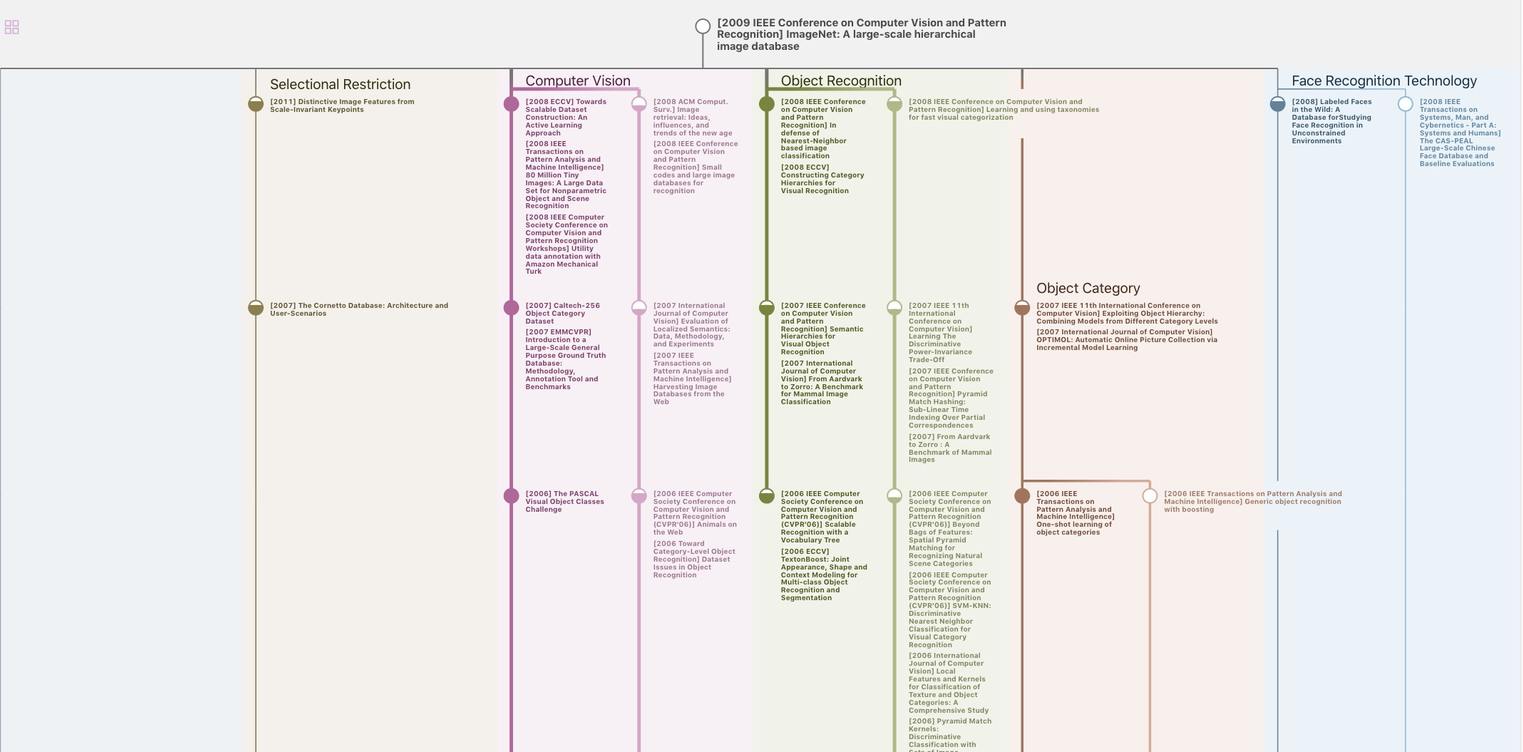
生成溯源树,研究论文发展脉络
Chat Paper
正在生成论文摘要