StableMiss+: Prediction with Incomplete Data Under Agnostic Mask Distribution Shift
ICASSP 2024 - 2024 IEEE International Conference on Acoustics, Speech and Signal Processing (ICASSP)(2024)
摘要
Missing data is ubiquitous in real-world scenarios. Recently, increasing attention has been given to prediction using only incomplete features together with a mask indicating the missing pattern. In this paper, we consider prediction with incomplete feature in the presence of distribution shift. In particular, we focus on the case where the joint distribution of complete feature and label is invariant, but the mask distribution may shift agnostically between training and testing. StableMiss is state-of-the-art in this problem. It removes correlations among feature, those among mask and those between feature and mask to avoid learning the correlations that possibly change under mask distribution shift. However, the correlations among feature can be helpful to prediction, since they do not change under mask distribution shift, and the optimal predictor, namely conditional expectation of label given incomplete feature, depends on them. To address this issue, we preserve the correlations among feature and simultaneously remove those among mask and those between feature and mask. Extensive experiments show that our method outperforms the state-of-the-art methods, with 10% reduction in RMSE.
更多查看译文
关键词
Prediction,incomplete data,agnostic mask distribution shift
AI 理解论文
溯源树
样例
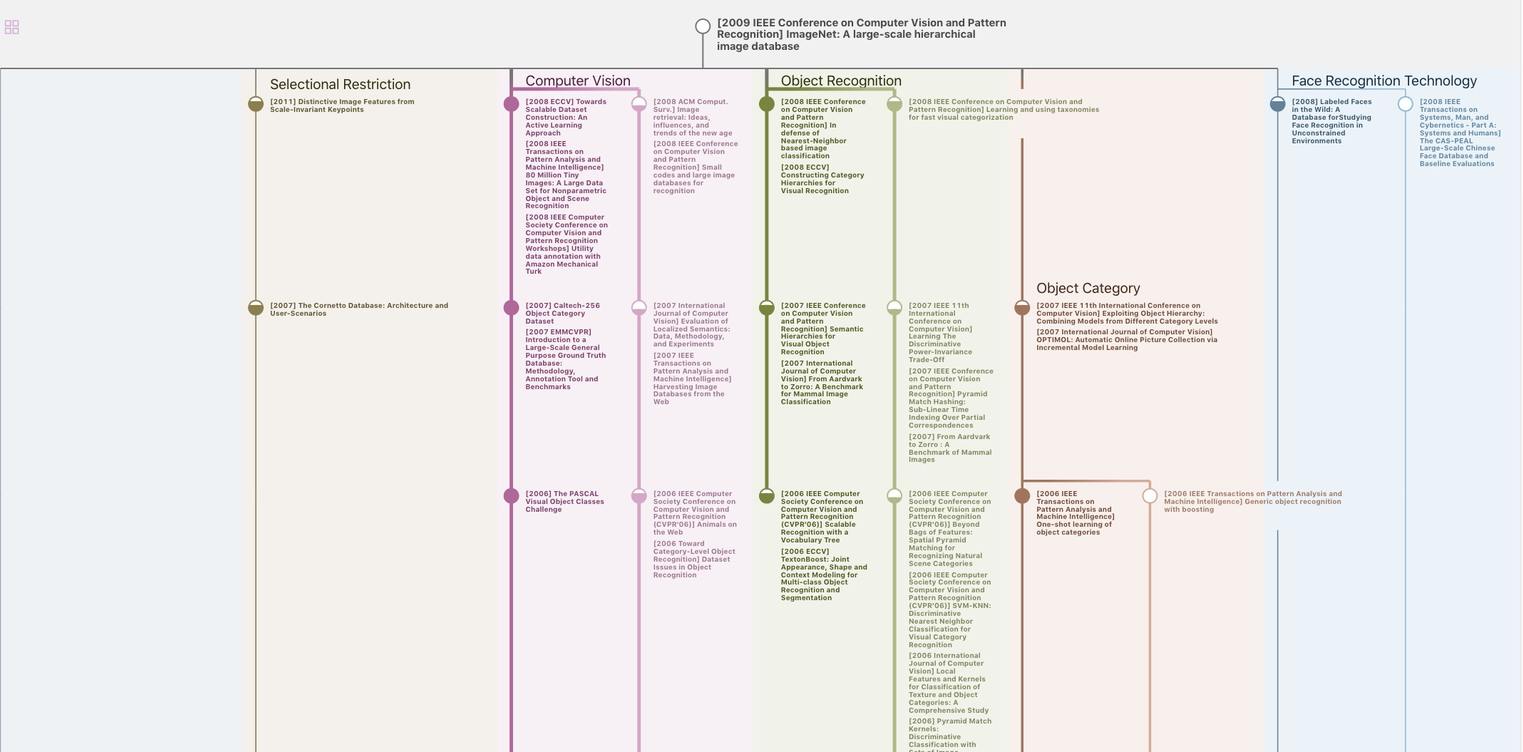
生成溯源树,研究论文发展脉络
Chat Paper
正在生成论文摘要