Leveraging Noisy Labels of Nearest Neighbors for Label Correction and Sample Selection
ICASSP 2024 - 2024 IEEE International Conference on Acoustics, Speech and Signal Processing (ICASSP)(2024)
摘要
Dealing with noisy labels (LNL) emerges as a critical challenge when applying deep learning (DL) in practical settings. Previous methodologies primarily concentrated on harnessing model predictions to mitigate the impact of noisy labels. Nevertheless, their efficacy is strongly contingent on the accuracy of model predictions, a factor that cannot be assured in the context of LNL. Our empirical analysis shows that in noisy datasets, the spatial information of latent feature representation combined with original noisy labels is more robust than the methods using model predictions. To mitigate the unreliability introduced by model predictions, we propose a novel Feature Representation method, which utilizes noisy labels of nearest neighbors for label Correction and sample Selection (FRCS). Extensive experiments on various benchmark datasets demonstrate the superiority of FRCS compared with SOTA methods. Our codes are available at https://github.com/tianfangjh/FRCS-Noisy-Labels.
更多查看译文
关键词
Noisy label,Label correction,Sample selection,Feature Representation
AI 理解论文
溯源树
样例
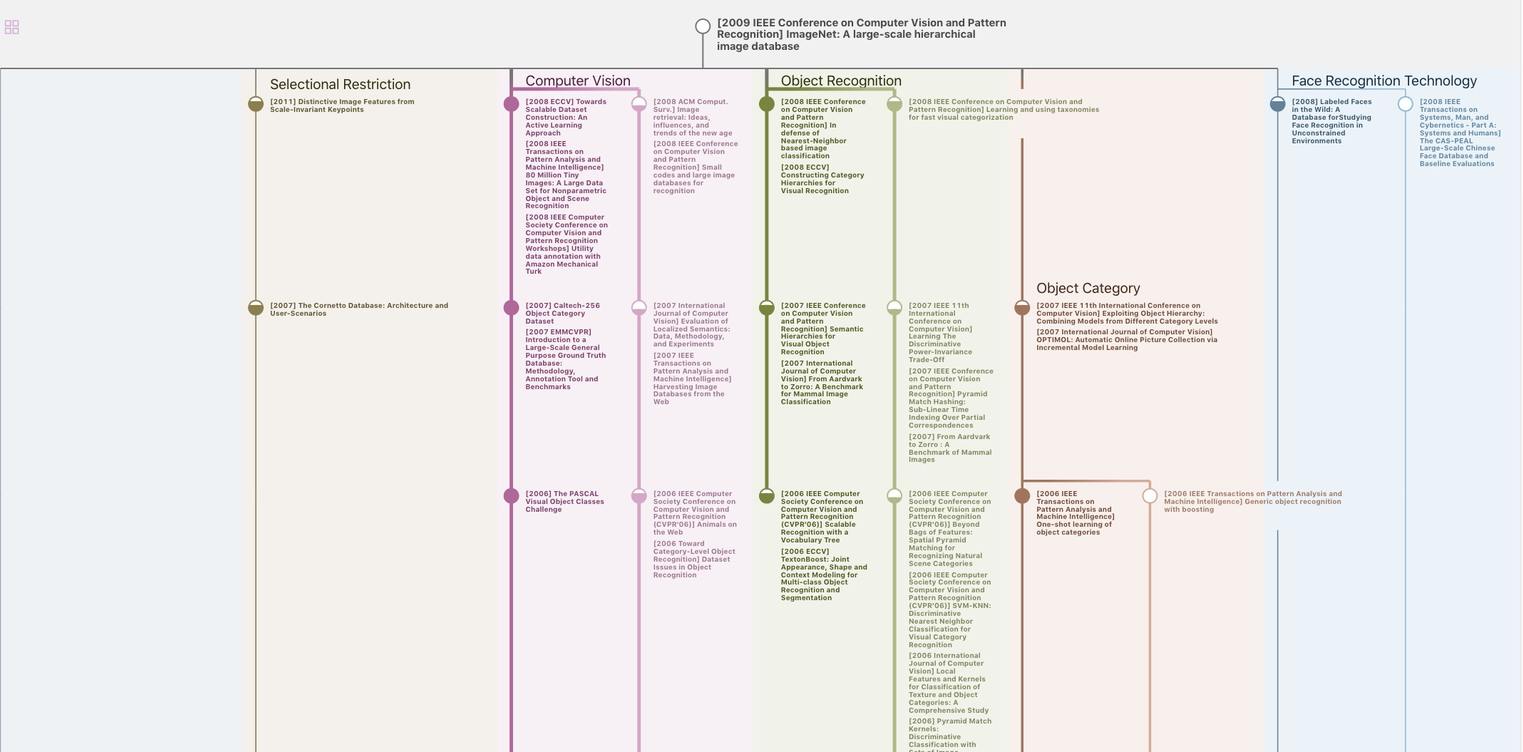
生成溯源树,研究论文发展脉络
Chat Paper
正在生成论文摘要