Enhancing GAN Performance Through Neural Architecture Search and Tensor Decomposition
ICASSP 2024 - 2024 IEEE International Conference on Acoustics, Speech and Signal Processing (ICASSP)(2024)
摘要
Generative Adversarial Networks (GANs) have emerged as a powerful tool for generating high-fidelity content. This paper presents a new training procedure that leverages Neural Architecture Search (NAS) to discover the optimal architecture for image generation while employing the Maximum Mean Discrepancy (MMD) repulsive loss for adversarial training. Moreover, the generator network is compressed using tensor decomposition to reduce its computational footprint and inference time while preserving its generative performance. Experimental results show improvements of 34% and 28% in the FID score on the CIFAR-10 and STL-10 datasets, respectively, with corresponding footprint reductions of 14× and 31× compared to the best FID score method reported in the literature. The implementation code is available at: https://github.com/PrasannaPulakurthi/MMD-AdversarialNAS.
更多查看译文
关键词
Neural Architecture Search,Maximum Mean Discrepancy,Generative Adversarial Networks
AI 理解论文
溯源树
样例
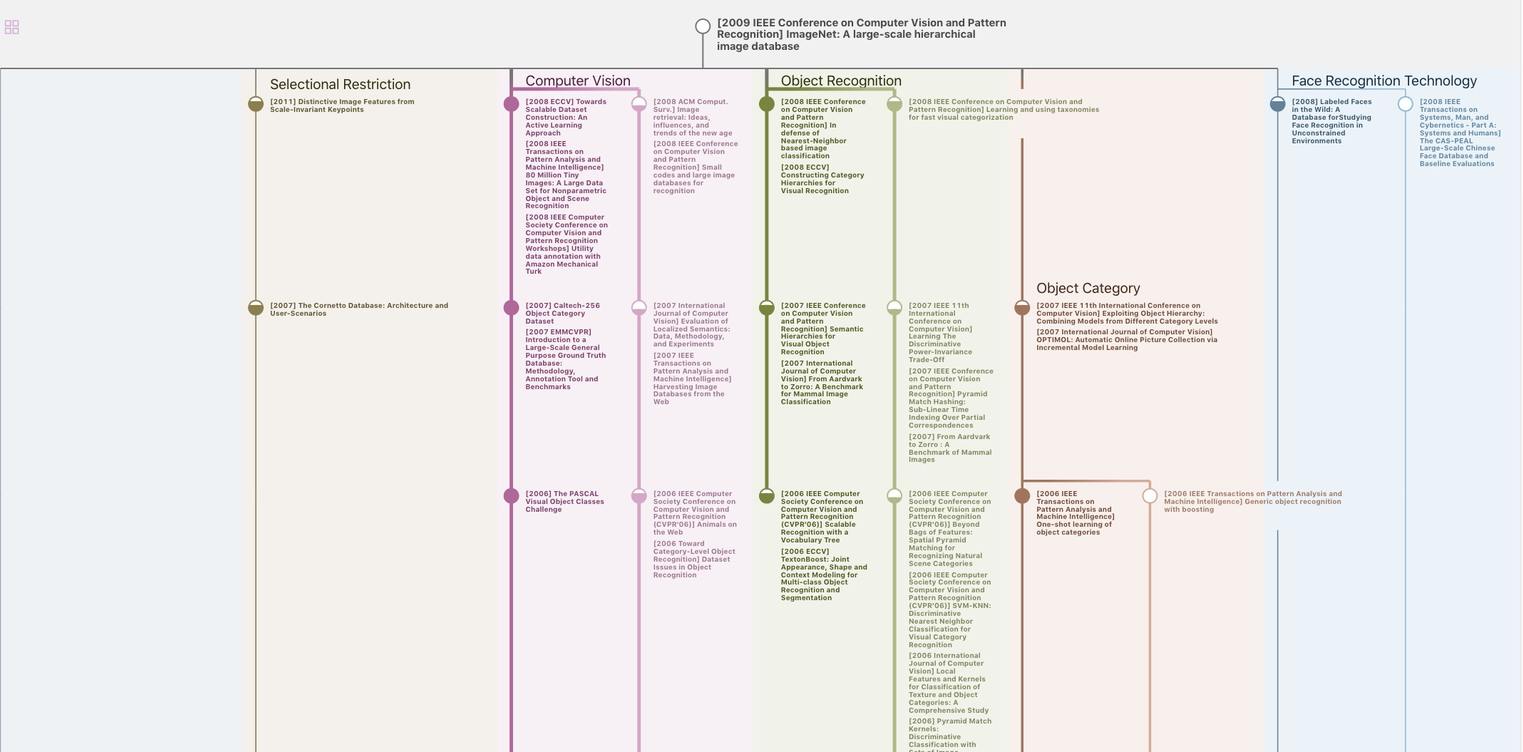
生成溯源树,研究论文发展脉络
Chat Paper
正在生成论文摘要