Scientific literature on carbon dioxide removal much larger than previously suggested: insights from an AI-enhanced systematic map
crossref(2024)
摘要
Abstract Carbon dioxide removal (CDR) is a critical component of any strategy to limit global warming to well below 2°C and rapidly gaining attention in climate research and policymaking. Despite its importance, there have been few attempts to systematically evaluate the scientific evidence on CDR. Here we use an approach rooted in artificial intelligence to produce a comprehensive systematic map of the CDR literature. In particular, we hand-label 5,339 documents to train machine learning classifiers with high levels of precision and recall to identify a total of 28,976 CDR studies across different technology domains and disciplines published in the period 1990-2022 which is at least 2-3 times more than previous studies suggested. We paint a granular picture of available CDR research in terms of the CDR methods studied, the geographical focus of research, the research method applied, and the broad area of research. The field has grown considerably faster than the climate change literature as a whole. This is driven mainly by the rapid expansion of literature on biochar, which made up about 62% of CDR publications in 2022. Beyond this stark concentration of CDR research on a few individual CDR methods, we find that most studies (86%) focus on improving the CDR methods themselves, but there is little research on their societal implications and ethical foundations. Citations patterns from the most recent IPCC report strongly differ from publication patterns on CDR in terms of its attention to CDR methods, research design and methodological context, as does attention to CDR methods in policy and practice in terms of real-world deployments, patenting activity, as well as public investments. As the importance of CDR grows for meeting the Paris climate goals, we believe that the accompanying literature database will be of additional value for the upcoming IPCC assessment, but also for science, policy and practice.
更多查看译文
AI 理解论文
溯源树
样例
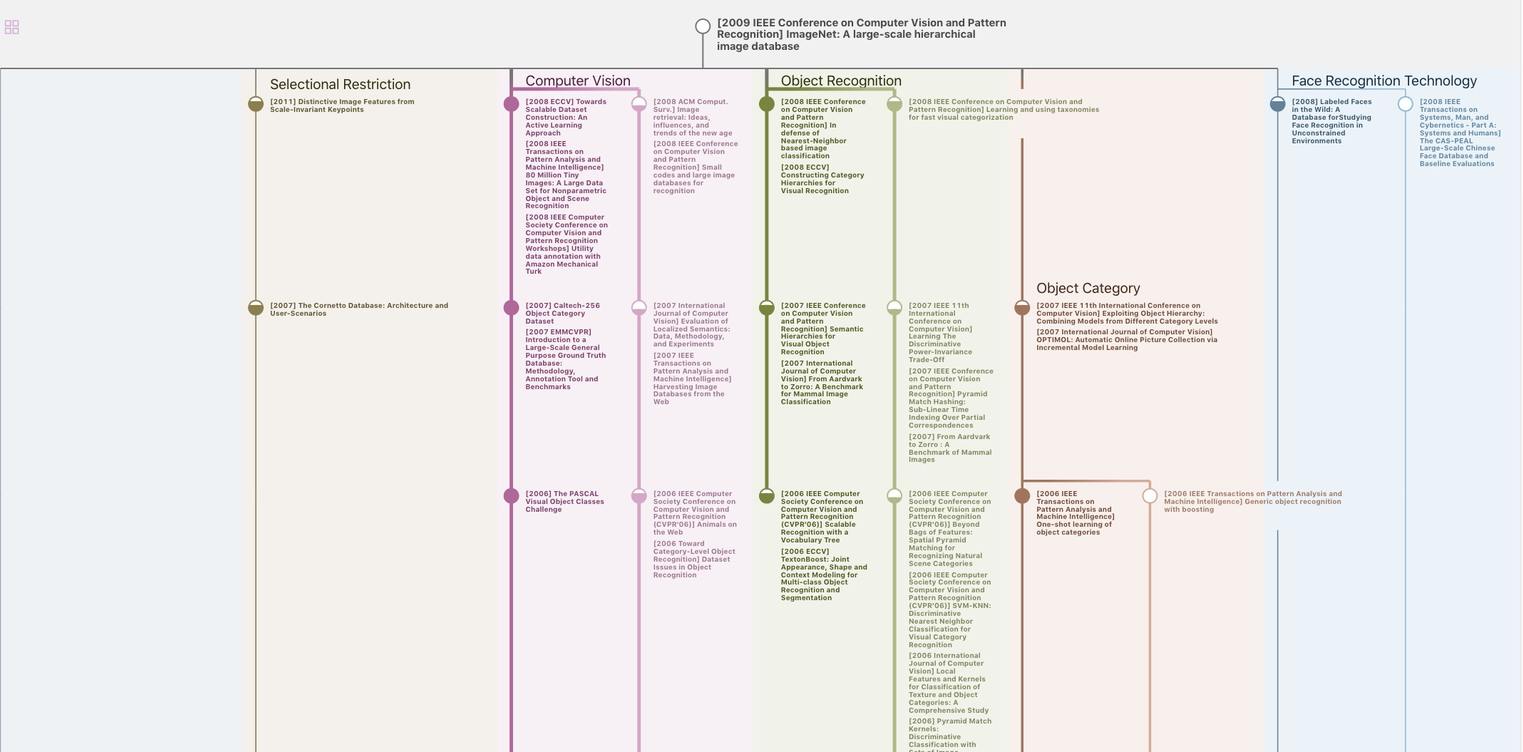
生成溯源树,研究论文发展脉络
Chat Paper
正在生成论文摘要