Domain-Adaptive Semantic Segmentation Emerges From Vision-Language Supervised Domain-Debiased Self-Training
ICASSP 2024 - 2024 IEEE International Conference on Acoustics, Speech and Signal Processing (ICASSP)(2024)
摘要
Unsupervised domain adaptive semantic segmentation leverages synthetic data to train a segmentation model and transfers it to unlabeled real images. Due to the style difference, the transferred model suffers from the domain gap. Even worse, some classes exhibit the extreme domain gap, where the feature distributions undergo a complete shift between the two domains. To alleviate it, we propose a domain-debiased self-training strategy with CLIP to distill its domain-agnostic knowledge. Specifically, we enforce the consistency between the feature maps from our segmentation model and the image encoder of CLIP. Meanwhile, the text embeddings from the text encoder for each class serve as a domain-agnostic classifier to support a domain-debiased feature learning condition. Experimental results under standard UDA settings demonstrate that our proposed strategy consistently improves the UDA segmentation performance based on different backbones and with different large pre-trained models.
更多查看译文
关键词
Unsupervised domain adaptation,semantic segmentation,vision language models,CLIP,feature distillation
AI 理解论文
溯源树
样例
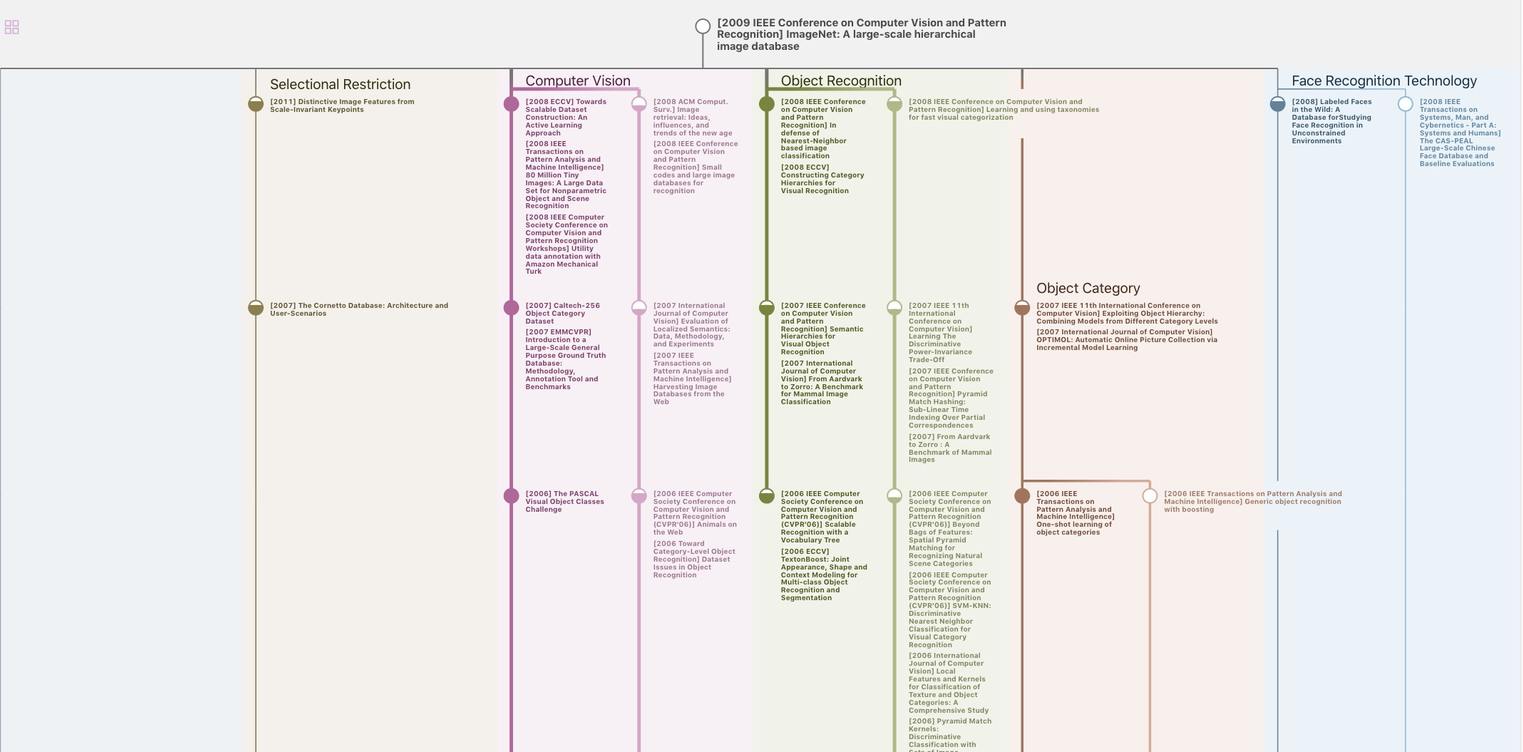
生成溯源树,研究论文发展脉络
Chat Paper
正在生成论文摘要