Exploring Soft Prompt Initialization Strategy for Few-Shot Continual Text Classification
ICASSP 2024 - 2024 IEEE International Conference on Acoustics, Speech and Signal Processing (ICASSP)(2024)
摘要
Few-shot continual learning (FSCL) is a challenging setting as it requires models to learn new knowledge with a few examples over time, and fast adapt to new tasks without forgetting previous knowledge. Prompt-tuning, as an efficient learning approach for language models, has shown competitive performance in data-efficient learning for various NLP tasks, motivating us to explore how to effectively perform prompt-tuning in FSCL for text classification. In this work, we focus on studying prompt-tuning for continual classification, aiming to alleviate catastrophic forgetting and improve knowledge transfer with few-shot data in FSCL. After carefully analyzing the limited representation capability of existing soft-prompt initialization methods, we propose Task-Aware Initialization (TAI), a novel initialization approach that can combine the information from both context and label space. Extensive experiments with different language models including recent instruction-finetuned LLM in two FSCL settings (shot-invariant and shot-variant) demonstrate the superiority of TAI over current approaches.
更多查看译文
关键词
Prompt-tunning,continual learning,text classification,few-shot learning,prompt initialization
AI 理解论文
溯源树
样例
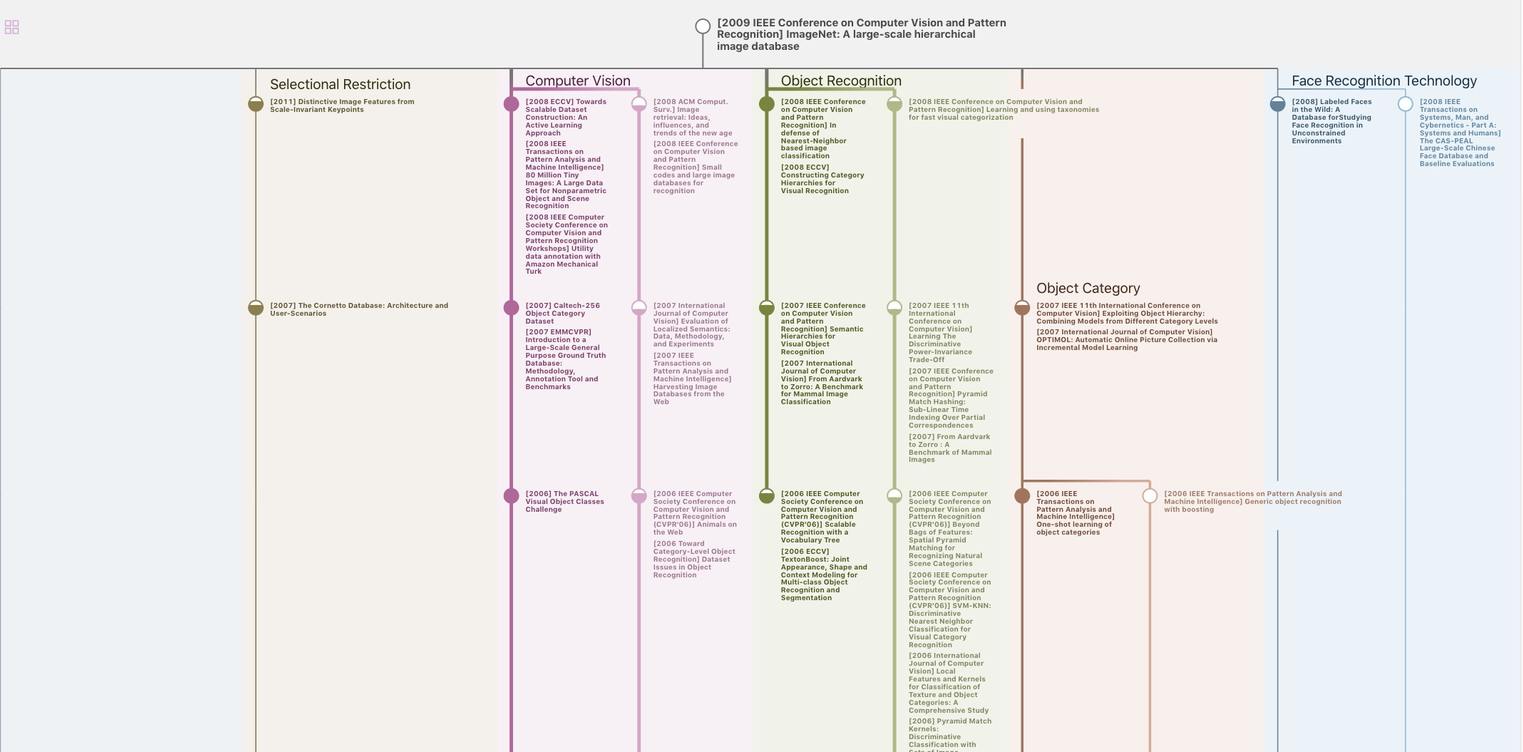
生成溯源树,研究论文发展脉络
Chat Paper
正在生成论文摘要