Human Guided Cross-Modal Reasoning with Semantic Attention Learning for Visual Question Answering
ICASSP 2024 - 2024 IEEE International Conference on Acoustics, Speech and Signal Processing (ICASSP)(2024)
摘要
One of the major difficulties in the Visual Question Answering (VQA) task of real-world images is the long-tailed distribution of concepts which makes the model vulnerable to negative linguistic biases. To imitate human learning and reasoning, researchers have designed reasoning models, which, however, is still a black-box process and cannot guarantee the visual interpretability of the final answer. How to guide the direction of the model reasoning and improve the generalization ability is a challenge to be solved. We proposed a novel Human-Guided Cross-Modal Reasoning (HGCMR) with semantic attention learning to improve the reasoning ability. The cross-modal reasoning module of HGCMR imitates the reasoning steps via semantic attention learning to generate the contextural image and question representation. The supervision module of HGCMR automatically extracts the human-guided attention distribution over object regions from the provided reasoning patterns, so as to guide the reasoning process. With the attended image and question representation and human reasoning supervision, the proposed HGCMR finally complete the question-answering task with an output classifier. By evaluating models on the real-world dataset GQA, our HGCMR improves compositional and grounding performance.
更多查看译文
关键词
Visual Question Answering,Cross-Modal Reasoning,Semantic Attention Learning
AI 理解论文
溯源树
样例
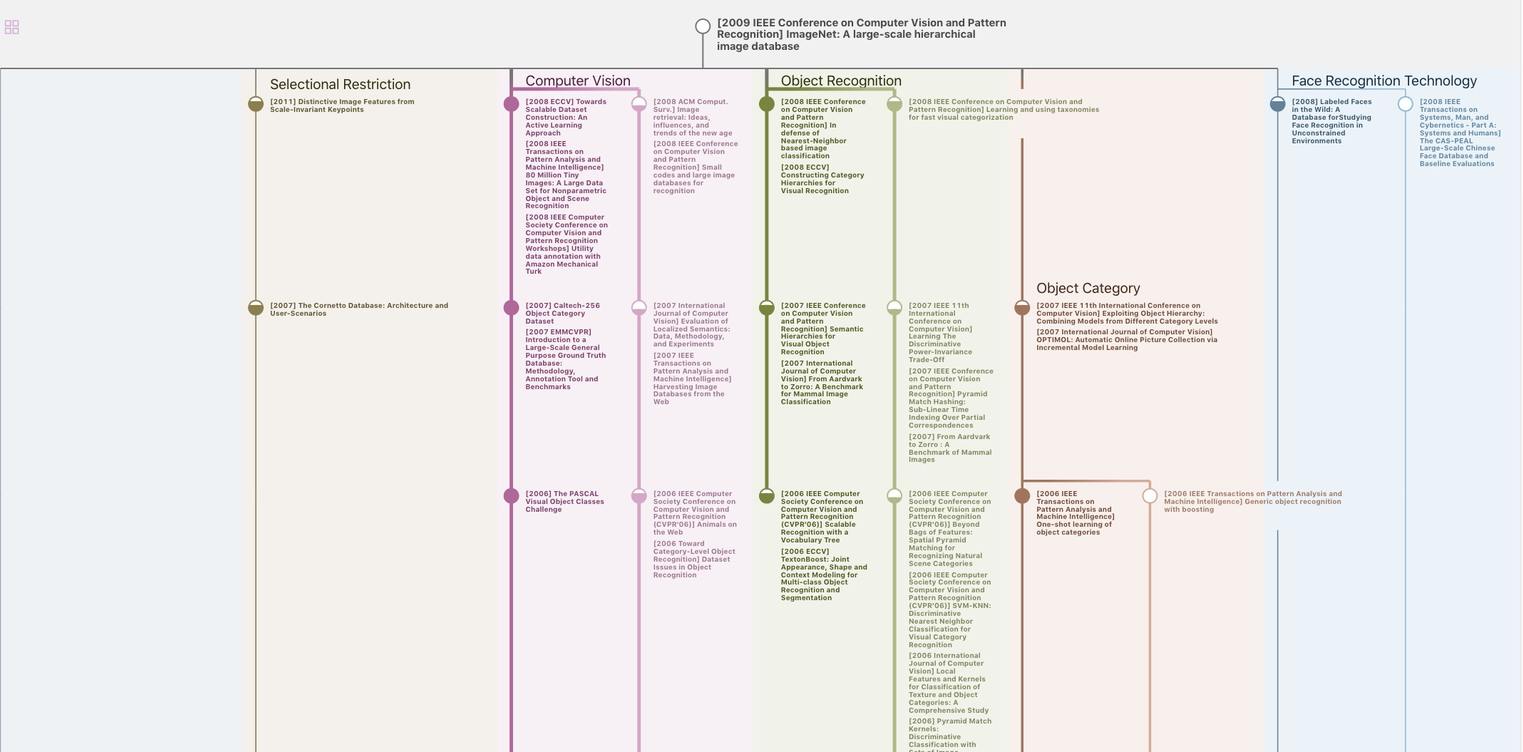
生成溯源树,研究论文发展脉络
Chat Paper
正在生成论文摘要