Large Language Models As A Proxy For Human Evaluation In Assessing The Comprehensibility Of Disordered Speech Transcription
ICASSP 2024 - 2024 IEEE International Conference on Acoustics, Speech and Signal Processing (ICASSP)(2024)
摘要
Automatic Speech Recognition (ASR) systems, despite significant advances in recent years, still have much room for improvement particularly in the recognition of disordered speech. Even so, erroneous transcripts from ASR models can help people with disordered speech be better understood, especially if the transcription doesn’t significantly change the intended meaning. Evaluating the efficacy of ASR for this use case requires a methodology for measuring the impact of transcription errors on the intended meaning and comprehensibility. Human evaluation is the gold standard for this, but it can be laborious, slow, and expensive. In this work, we tune and evaluate large language models for this task and find them to be a much better proxy for human evaluators than other metrics commonly used. We further present a case-study using the presented approach to assess the quality of personalized ASR models to make model deployment decisions and correctly set user expectations for model quality as part of our trusted tester program.
更多查看译文
关键词
Automatic speech recognition,model quality evaluation,speech disorders
AI 理解论文
溯源树
样例
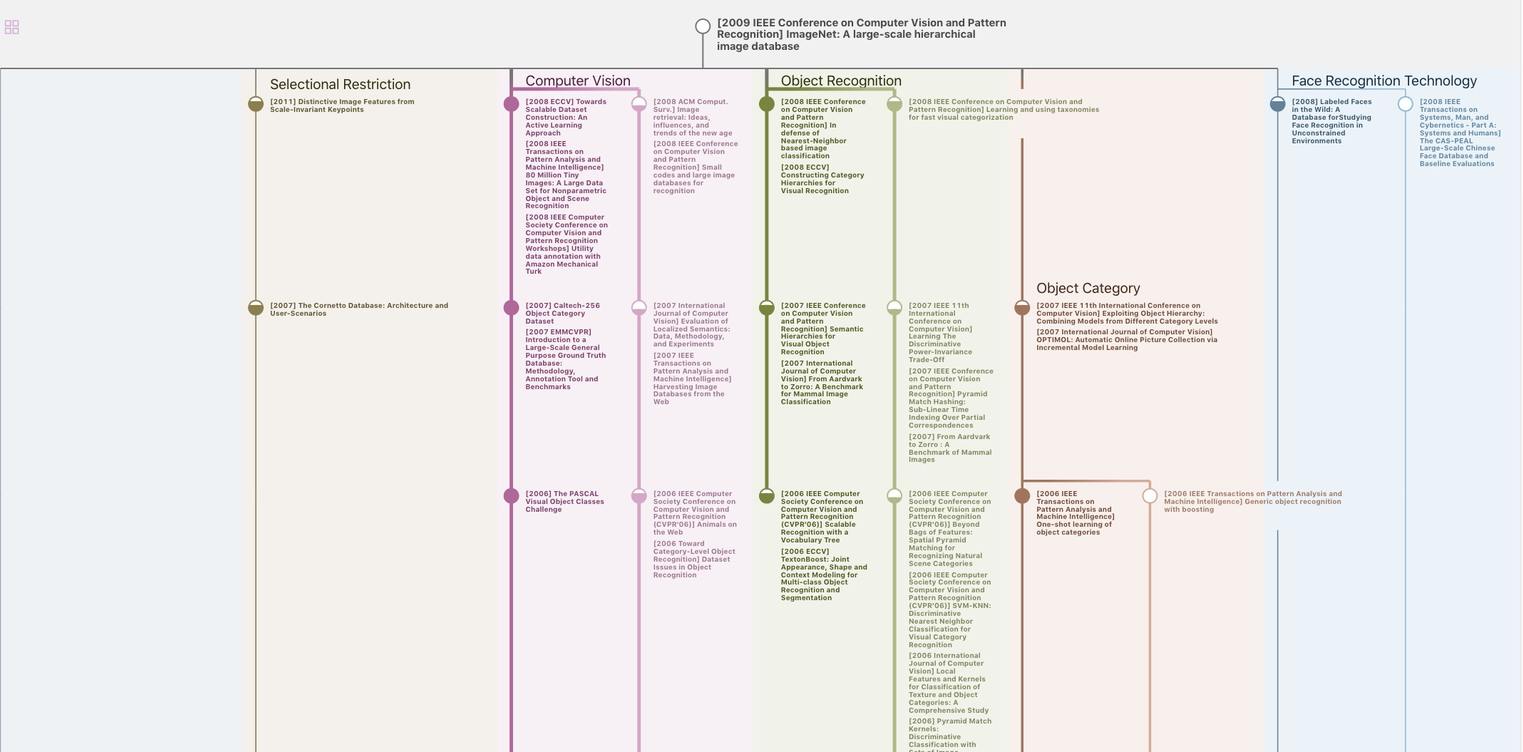
生成溯源树,研究论文发展脉络
Chat Paper
正在生成论文摘要