Variance-Dependent Regret Bounds for Non-stationary Linear Bandits
arxiv(2024)
摘要
We investigate the non-stationary stochastic linear bandit problem where the
reward distribution evolves each round. Existing algorithms characterize the
non-stationarity by the total variation budget B_K, which is the summation of
the change of the consecutive feature vectors of the linear bandits over K
rounds. However, such a quantity only measures the non-stationarity with
respect to the expectation of the reward distribution, which makes existing
algorithms sub-optimal under the general non-stationary distribution setting.
In this work, we propose algorithms that utilize the variance of the reward
distribution as well as the B_K, and show that they can achieve tighter
regret upper bounds. Specifically, we introduce two novel algorithms: Restarted
WeightedOFUL^+ and Restarted SAVE^+. These algorithms address
cases where the variance information of the rewards is known and unknown,
respectively. Notably, when the total variance V_K is much smaller than K,
our algorithms outperform previous state-of-the-art results on non-stationary
stochastic linear bandits under different settings. Experimental evaluations
further validate the superior performance of our proposed algorithms over
existing works.
更多查看译文
AI 理解论文
溯源树
样例
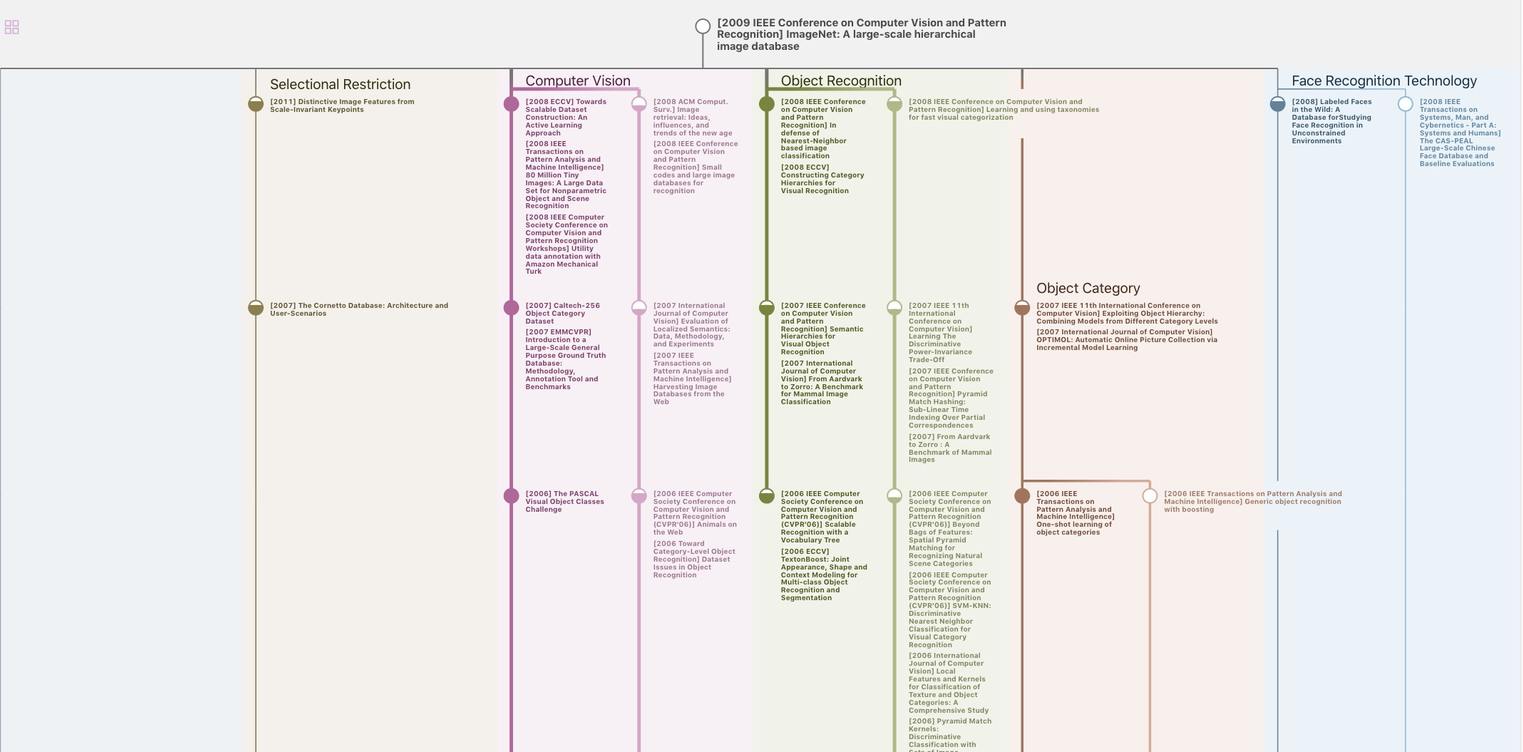
生成溯源树,研究论文发展脉络
Chat Paper
正在生成论文摘要