On-Device Domain Learning for Keyword Spotting on Low-Power Extreme Edge Embedded Systems
CoRR(2024)
摘要
Keyword spotting accuracy degrades when neural networks are exposed to noisy
environments. On-site adaptation to previously unseen noise is crucial to
recovering accuracy loss, and on-device learning is required to ensure that the
adaptation process happens entirely on the edge device. In this work, we
propose a fully on-device domain adaptation system achieving up to 14
gains over already-robust keyword spotting models. We enable on-device learning
with less than 10 kB of memory, using only 100 labeled utterances to recover 5
accuracy after adapting to the complex speech noise. We demonstrate that domain
adaptation can be achieved on ultra-low-power microcontrollers with as little
as 806 mJ in only 14 s on always-on, battery-operated devices.
更多查看译文
AI 理解论文
溯源树
样例
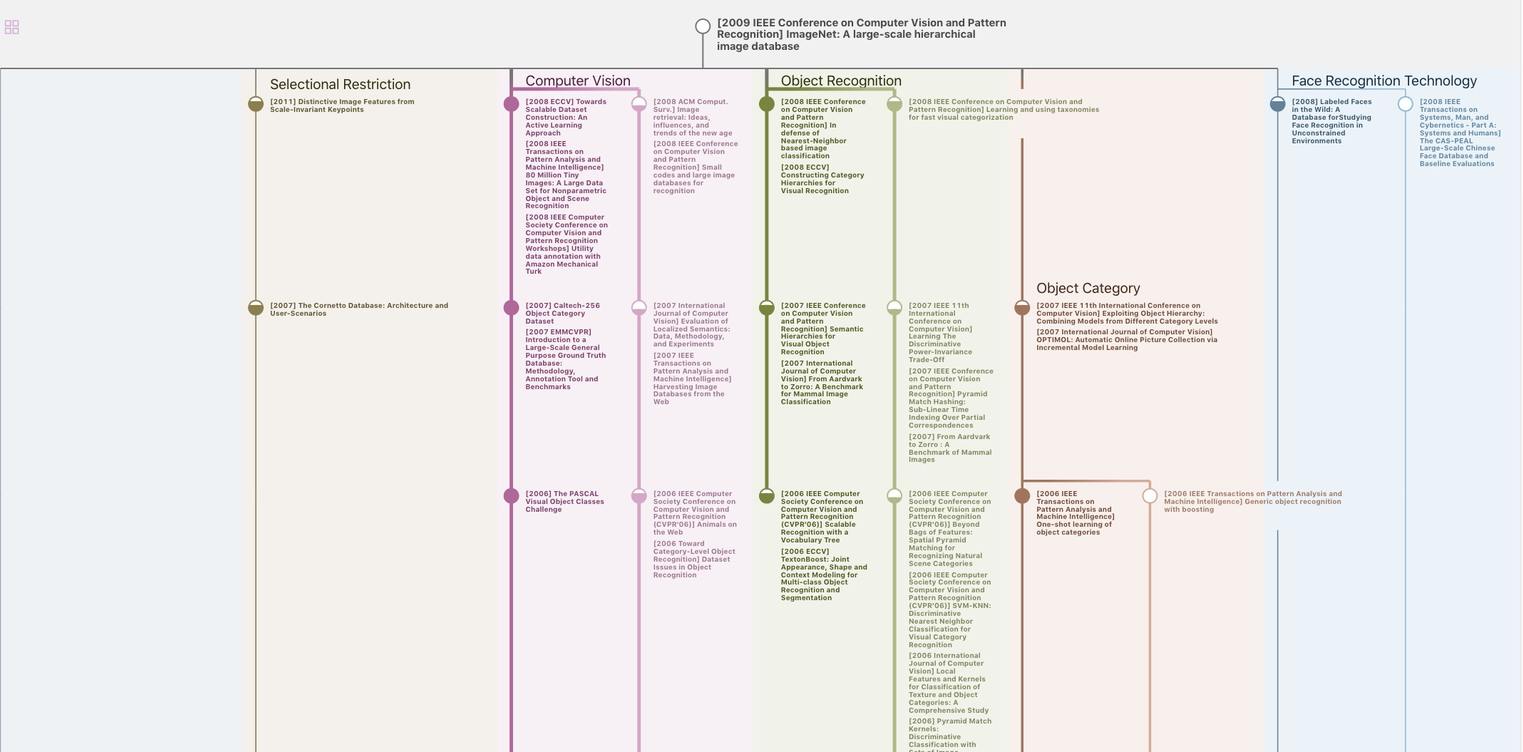
生成溯源树,研究论文发展脉络
Chat Paper
正在生成论文摘要