Fusing AraBERT and Graph Neural Networks for Enhanced Arabic Text Classification.
Arab Conference on Information Technology(2023)
摘要
Text classification is a fundamental task in natural language processing, and has been widely studied for various languages. However, Arabic text classification is challenging due to the complexity of Arabic (i.e., rich morphological structure), high degree of ambiguity, and optional diacritics in the writing system. Large-scale pre-trained contextualized models (e.g., AraBERT) can successfully capture semantics in the local context. On the other hand, graph-based models could capture the global context by incorporating long-distance semantics. A text classification model combining the local and global context can enhance classifier performance for highly complex and ambiguous languages. In this work, we introduce the Arabic BERT Graph Convolutional Network (AraBERT-GCN), that can leverage the advantage of using large-scale pre-trained models alongside graph convolutional networks. Experimental results show that AraBERT-GCN outperforms the state-of-the-art (SOTA) on our Arabic text datasets.
更多查看译文
关键词
Graph convolutional networks,Arabic text classification,AraBERT-GCN
AI 理解论文
溯源树
样例
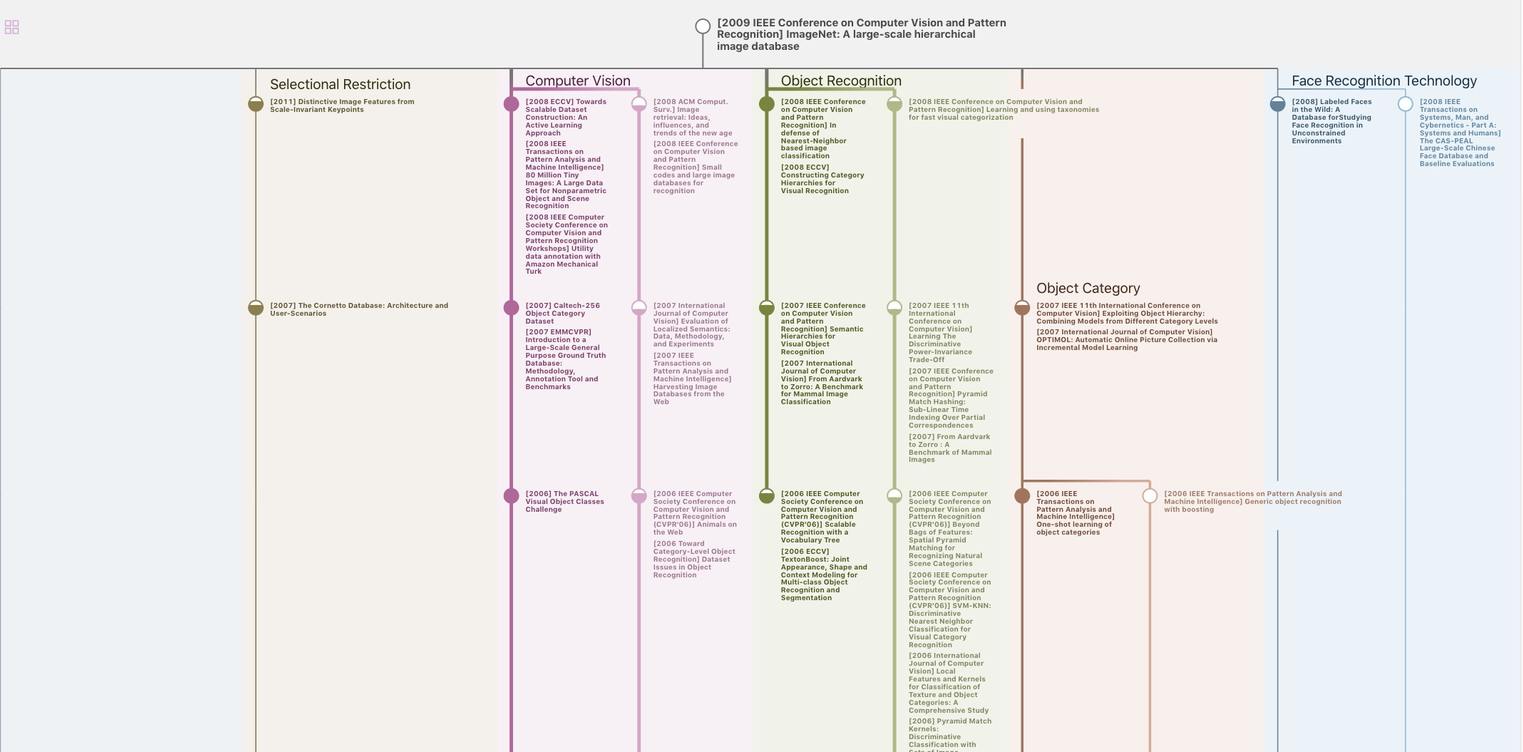
生成溯源树,研究论文发展脉络
Chat Paper
正在生成论文摘要