Robust Evolving Fuzzy Classifier Integrating Noise Smoothing and Soft Dimension Reduction
IEEE Transactions on Emerging Topics in Computational Intelligence(2024)
摘要
We propose a new evolving fuzzy classifier approach termed as
EFC-RNG (Evolving Fuzzy Classifier based on Robust Neural Gas)
acting in a fully single-pass, sample-wise incremental manner and embedding advanced robustness aspects i) by integrating a noise smoothing technique during the rule updates, ii) by an incremental feature weighting concept for the purpose of soft dimension reduction, and iii) by a specific supervised rule evolution criterion in order to handle class imbalance problems. The latter assures that in class overlapping regions, where majority classes dominate and overwhelm minority classes, minority classes become still active in separate rules (in their output consequences). The update of the rule centers is achieved through a neural gas based weighting concept. The noise smoothing concept is integrated through the definition of a new distance measure into the neural gas based objective function, leading to a new noise- and outlier-smoothened update of the rule centers with neural gas weights. The incremental feature weighting technique is based on the
feature relevance (FR)
concept, which integrates the entropy and mutual information of features and is sample-wise updated through a discretization approach based on histogram bins. The integration of the feature weights into the classifier updates is done in the rule evolution criterion, inducing a shrinking effect along unimportant features, thus decreasing the likelihood to evolve new unnecessary rules (avoidance of over-fitting due to the curse of dimensionality). Our approach was evaluated based on several randomly selected data streams from the UCI repository, containing some highly imbalanced data sets and streams with different input dimensionality and sample size. The evaluation is based on a profound comparison with related evolving fuzzy classifier methods, including a statistical preference analysis based on accumulated accuracy measures. Additionally, we evaluated our approach within the context of an online evolving multi-user classification framework, where multiple users with different experience levels may provide feedback in form of class labels and where our EFC-RNG approach served as evolving base classifier.
更多查看译文
关键词
Evolving fuzzy classifiers,evolving neural gas clustering,feature relevance,incremental feature weighting,noise and outlier smoothing,online data streams
AI 理解论文
溯源树
样例
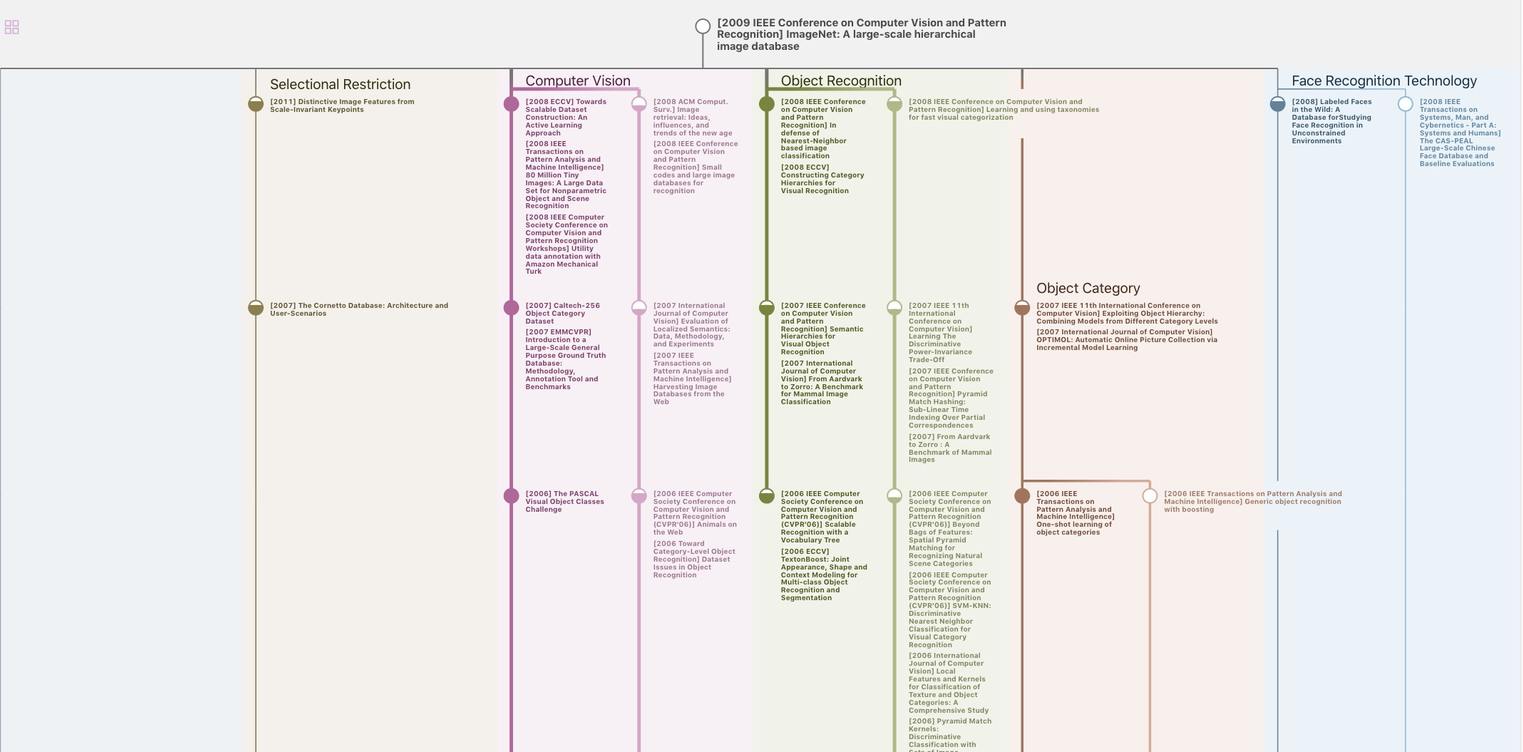
生成溯源树,研究论文发展脉络
Chat Paper
正在生成论文摘要