Training Generative Adversarial Network-Based Vocoder with Limited Data Using Augmentation-Conditional Discriminator
ICASSP 2024 - 2024 IEEE International Conference on Acoustics, Speech and Signal Processing (ICASSP)(2024)
摘要
A generative adversarial network (GAN)-based vocoder trained with an
adversarial discriminator is commonly used for speech synthesis because of its
fast, lightweight, and high-quality characteristics. However, this data-driven
model requires a large amount of training data incurring high data-collection
costs. This fact motivates us to train a GAN-based vocoder on limited data. A
promising solution is to augment the training data to avoid overfitting.
However, a standard discriminator is unconditional and insensitive to
distributional changes caused by data augmentation. Thus, augmented speech
(which can be extraordinary) may be considered real speech. To address this
issue, we propose an augmentation-conditional discriminator (AugCondD) that
receives the augmentation state as input in addition to speech, thereby
assessing the input speech according to the augmentation state, without
inhibiting the learning of the original non-augmented distribution.
Experimental results indicate that AugCondD improves speech quality under
limited data conditions while achieving comparable speech quality under
sufficient data conditions. Audio samples are available at
https://www.kecl.ntt.co.jp/people/kaneko.takuhiro/projects/augcondd/.
更多查看译文
关键词
Speech synthesis,neural vocoder,generative adversarial networks,limited data,data augmentation
AI 理解论文
溯源树
样例
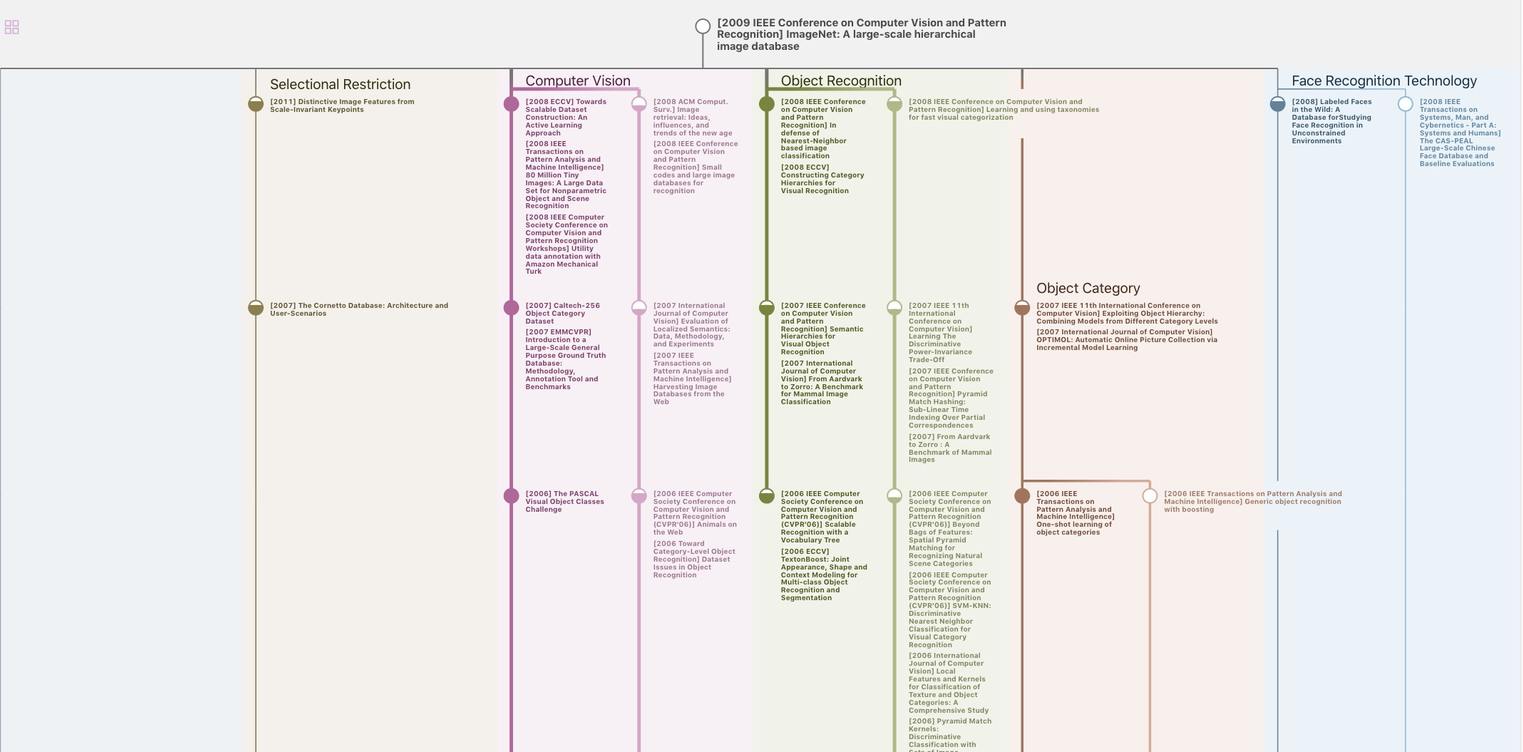
生成溯源树,研究论文发展脉络
Chat Paper
正在生成论文摘要