Max-AST: Combining Convolution, Local and Global Self-Attentions for Audio Event Classification
ICASSP 2024 - 2024 IEEE International Conference on Acoustics, Speech and Signal Processing (ICASSP)(2024)
摘要
In the domain of audio transformer architectures, prior research has extensively investigated isotropic architectures that capture the global context through full self-attention and hierarchical architectures that progressively transition from local to global context utilising hierarchical structures with convolutions or window-based attention. However, the idea of imbuing each individual block with both local and global contexts, thereby creating a hybrid transformer block, remains relatively under-explored in the field.To facilitate this exploration, we introduce Multi Axis Audio Spectrogram Transformer (Max-AST), an adaptation of MaxViT to the audio domain. Our approach leverages convolution, local window-attention, and global grid-attention in all the transformer blocks. The proposed model excels in efficiency compared to prior methods and consistently outperforms state-of-the-art techniques, achieving significant gains of up to 2.6% on the AudioSet full set. Further, we performed detailed ablations to analyse the impact of each of these components on audio feature learning. The source code is available at https://github.com/ta012/MaxAST.git
更多查看译文
关键词
Transformer,Convolution,Self-Attention,Audio Classification,Local-Global Context
AI 理解论文
溯源树
样例
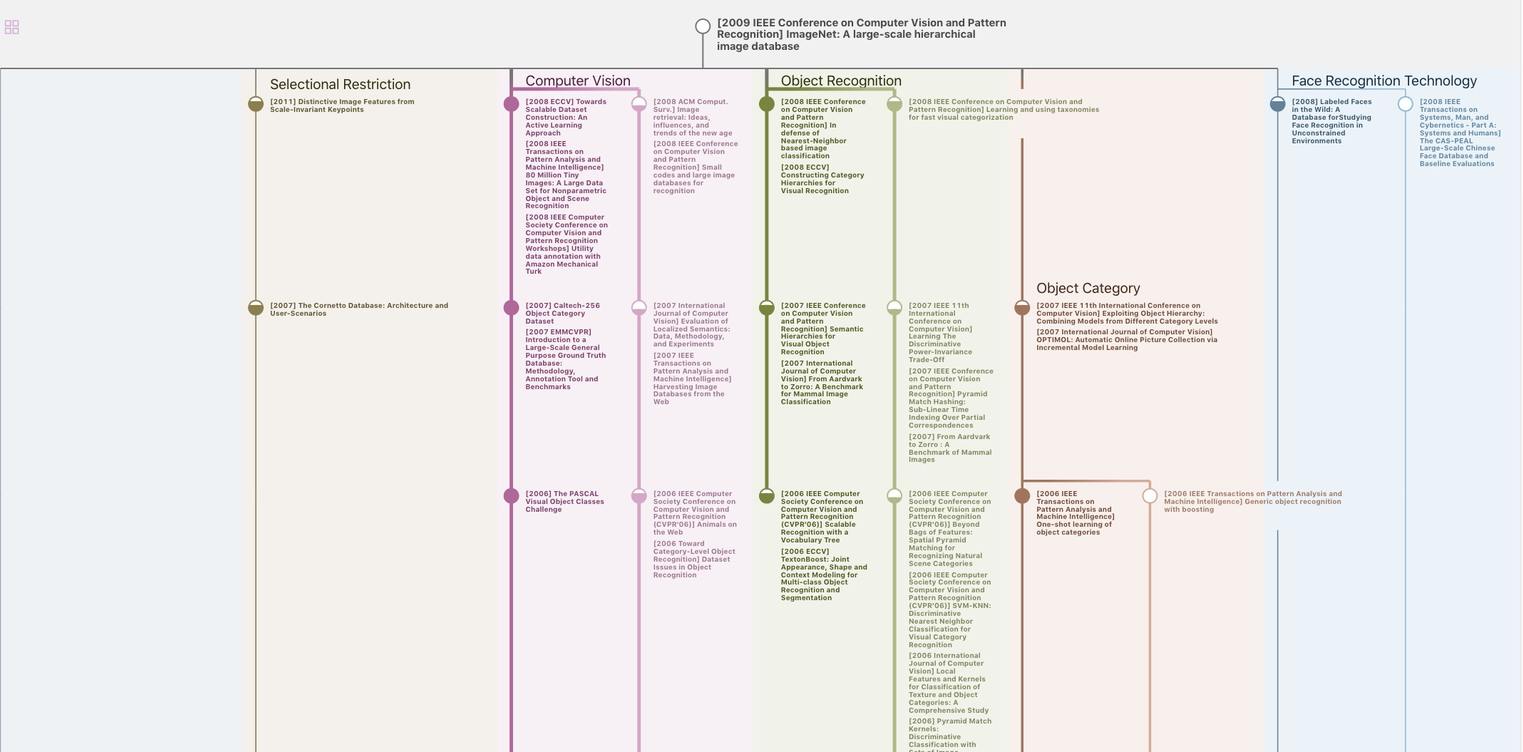
生成溯源树,研究论文发展脉络
Chat Paper
正在生成论文摘要