Rating-Augmented No-Reference Point Cloud Quality Assessment Using Multi-Task Learning
ICASSP 2024 - 2024 IEEE International Conference on Acoustics, Speech and Signal Processing (ICASSP)(2024)
摘要
The diversity and multi-dimensionality of point cloud make the no-reference point cloud quality assessment challenging. However, existing learning-based methods pay little attention on the distribution inconsistency of quality scores among different datasets, which would induce training bias during the regression stage. Consequently, most of current metrics suffer from generalization degradation. Inspired by the human progressive learning process, we present a novel multi-task no-reference point cloud assessment framework concerning both the quality score regression and classification. Particularly, a rating-augmented constraint with respect to the subjective rating process is utilized to suppress the training bias induced by regression. Experimental results show that our method achieves competitive performance in comparison with state-of-the-art methods. Moreover, the proposed rating-augmented multi-task learning scheme can effectively promote the generalization performance.
更多查看译文
关键词
point cloud quality assessment,no-reference,multi-grain,rating augmentation,multi-modal
AI 理解论文
溯源树
样例
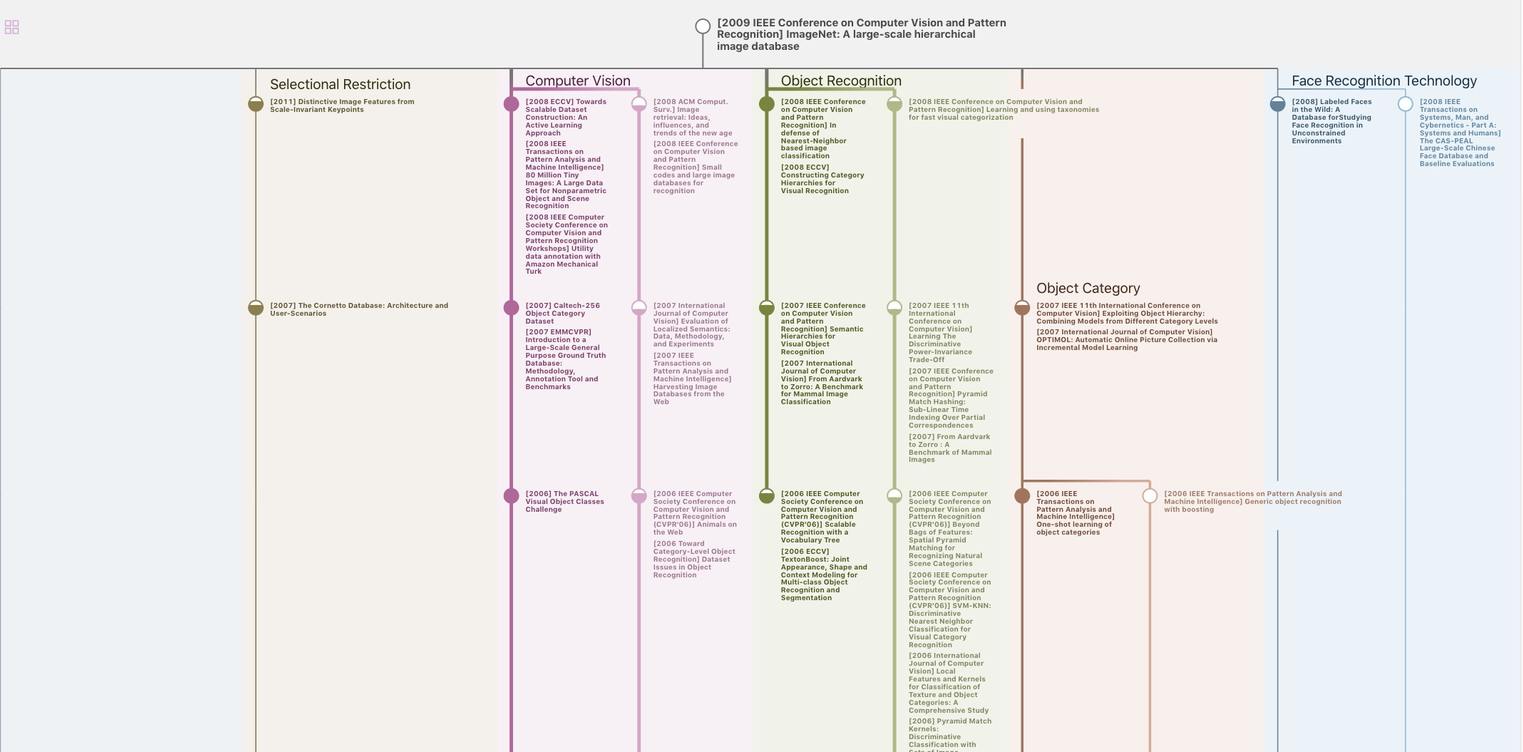
生成溯源树,研究论文发展脉络
Chat Paper
正在生成论文摘要