Novel Distribution Distance Based on Inconsistent Adaptive Region for Change Detection Using Hyperspectral Remote Sensing Images
IEEE TRANSACTIONS ON GEOSCIENCE AND REMOTE SENSING(2024)
摘要
Change detection with remote sensing images (RSIs) plays an important role in the community of remote sensing applications. However, when change detection is conducted with hyperspectral RSIs (HRSIs), how to measure the change magnitude between bitemporal HRSIs becomes challenging due to the high dimension of HRSIs. In this article, a novel distribution distance based on inconsistent adaptive region ( D-2 IAR) change detection approach is proposed to measure the change magnitude between bitemporal HRSIs for improving the performance of change detection with HRSIs. First, a band selection algorithm called optimal neighborhood reconstruction (ONR) is employed to reduce the dimensions of HRSIs. Then, an adaptive region around each pixel is generated to explore the contextual feature around each pixel, and kernel density estimation (KDE) is suggested to estimate the spectral distribution of the pixels within an adaptive region. A distribution distance is defined based on the adaptive region to measure the change magnitude between bitemporal HRSIs. Finally, the change magnitude between pairwise adaptive regions is measured by the proposed distance between the pairwise distributions. Experimental results based on four datasets and comparisons with eight methods indicated the feasibility and superiorities of the proposed D-2 IAR-based change detection approach with HRSIs. The improvement rates are approximately 0.13%-24.04% for overall accuracy. The code and datasets can be available at: https://github.com/ImgSciGroup/2024-HSICD.
更多查看译文
关键词
Feature extraction,Atmospheric measurements,Shape,Hyperspectral imaging,Deep learning,Vectors,Tensors,Hyperspectral remote sensing images (HRSIs),land cover change detection (LCCD),nonsubsampled shearlet transform (NSST),spatial context
AI 理解论文
溯源树
样例
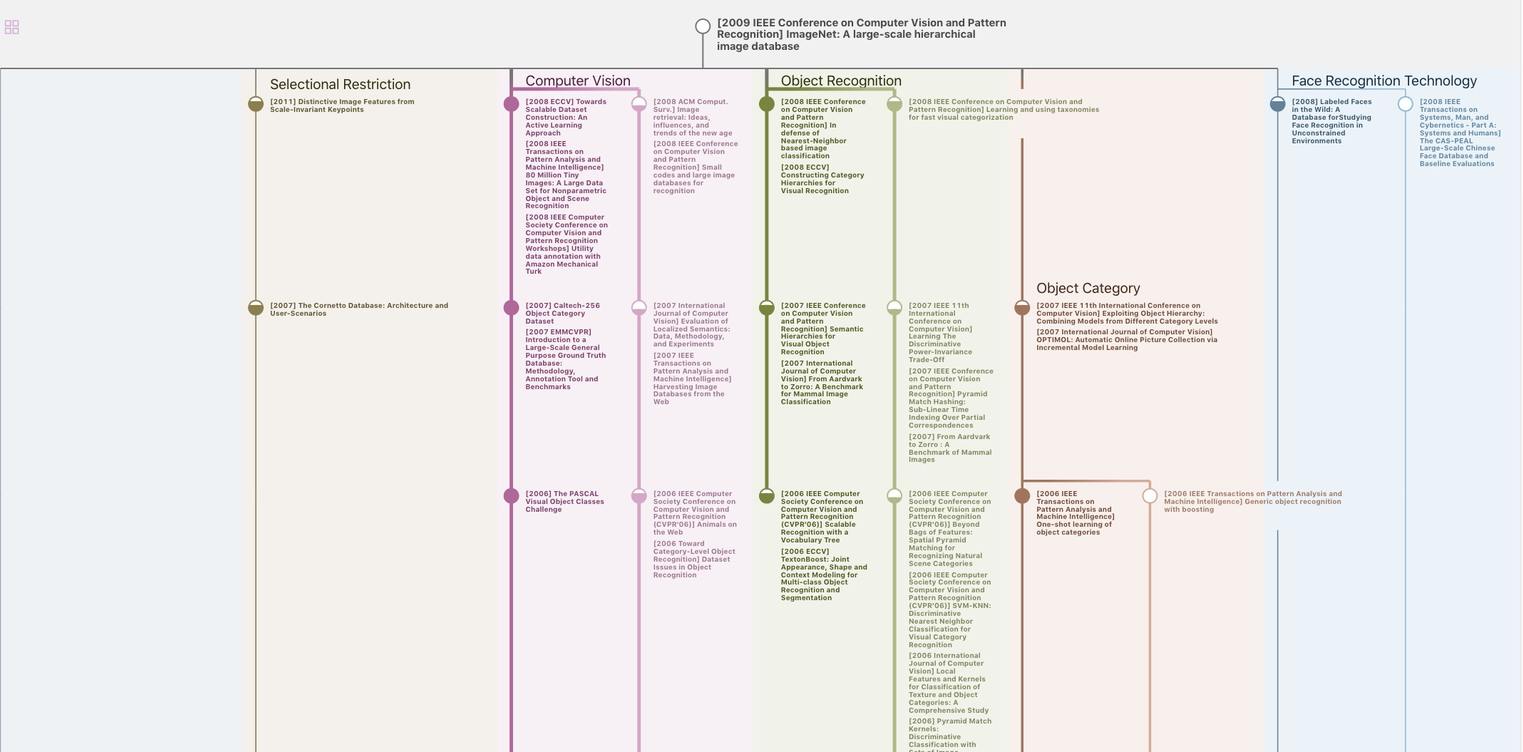
生成溯源树,研究论文发展脉络
Chat Paper
正在生成论文摘要