DualGCN-MIL: Whole Slide Image Classification Based on Double Relationship Graph Learning
ICASSP 2024 - 2024 IEEE International Conference on Acoustics, Speech and Signal Processing (ICASSP)(2024)
摘要
The resolution of a whole slide image (WSI) is too large to process directly, but WSI can be segmented into patches and be classified through multiple instance learning (MIL). Some patches have either close distances or similar pathological morphology, indicating that there are at least two types of relationships between patches. However, the existing MIL methods often deal with instance relationships simplistically. To solve this problem, we propose a new model named DualGCN-MIL. It analyzes the two types of relationships in WSI by constructing two different graphs in high-dimensional feature space and physical coordinate space and uses graph convolutional networks with unshared parameters for learning. Various experiments conducted on the Camelyon dataset have shown that compared to state-of-the-art methods, we can achieve better performance in multiple indicators, with accuracy of 88.89% and 85.11% on the 16 and 17 datasets, respectively, which proves that DualGCN-MIL has a higher accuracy in WSI classification. Implementation can be obtained from https://github.com/UnmatchedKatana/DualGCN-MIL.
更多查看译文
关键词
Whole slide image,classification,multiple instance learning,graph convolution
AI 理解论文
溯源树
样例
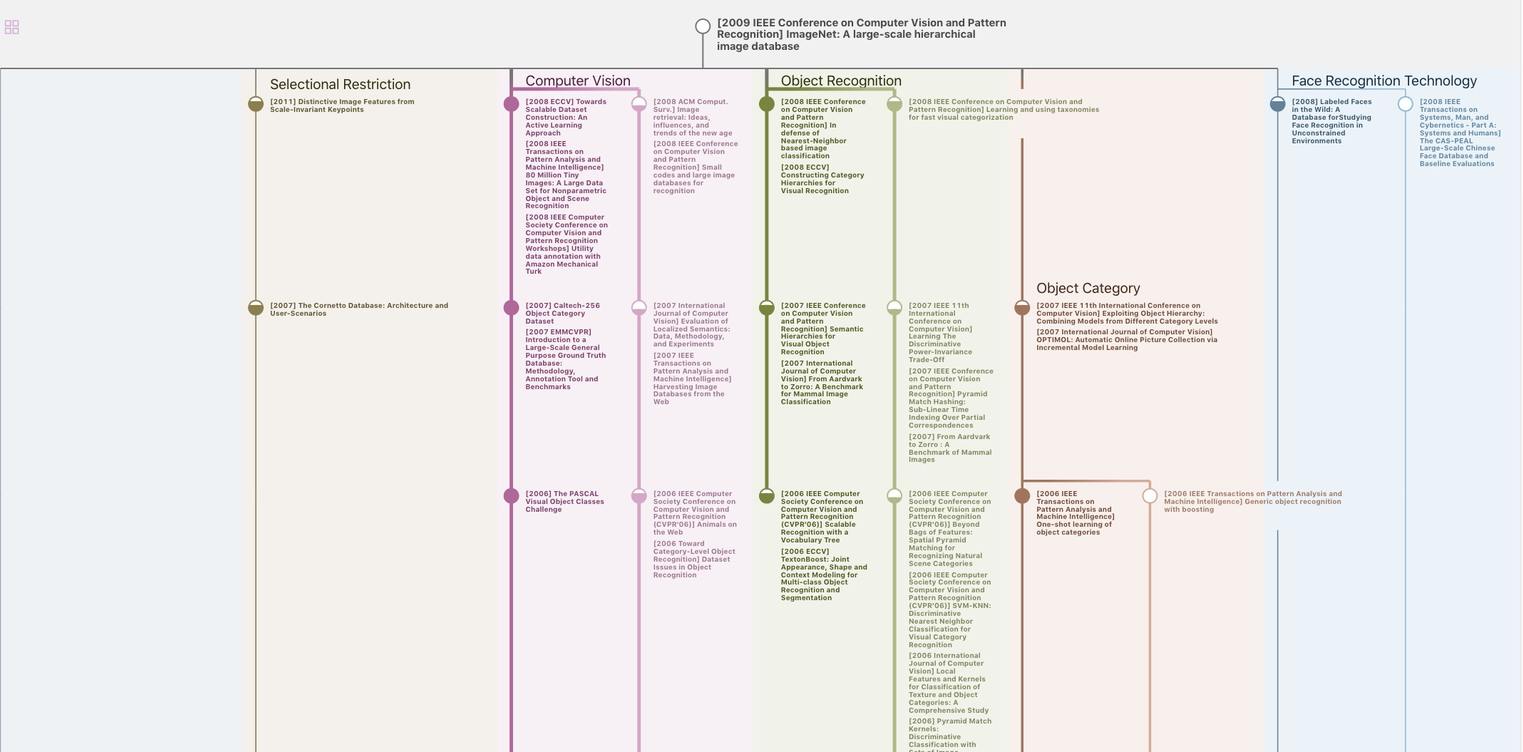
生成溯源树,研究论文发展脉络
Chat Paper
正在生成论文摘要