Inferring Time Varying Signals over Uncertain Graphs
ICASSP 2024 - 2024 IEEE International Conference on Acoustics, Speech and Signal Processing (ICASSP)(2024)
摘要
Inference of time varying data over graphs is of importance in real-world applications such as urban water networks, economics, and brain recordings. It typically relies on identifying a computationally affordable joint spatiotemporal method that can leverage the patterns in the data. While this per se is a challenging task, it becomes even more so when the network comes with uncertainties, which, if not accounted for, can lead to unpredictable consequences. To target this setting, we model graph uncertainties as Gaussian noise on the edges and design a stochastic partial differential equation (SPDE) based on it. We use this SPDE as a state equation to model the time varying signal evolution and extend it further to a state-space model where the observations are graph-filtered versions of the state. This allows us to have a joint spatiotemporal expressive kernel that can be estimated online via Kalman filtering and which parameters can also be estimated online via maximum likelihood principles, ultimately, reducing the computational cost. We corroborate the proposed approach on numerical experiments, showing a superior performance to approaches ignoring either the uncertainty or considering a separable spatiotemporal kernel.
更多查看译文
关键词
Time-varying graph signals,Stochastic partial differential equations,Gaussian processes on graphs
AI 理解论文
溯源树
样例
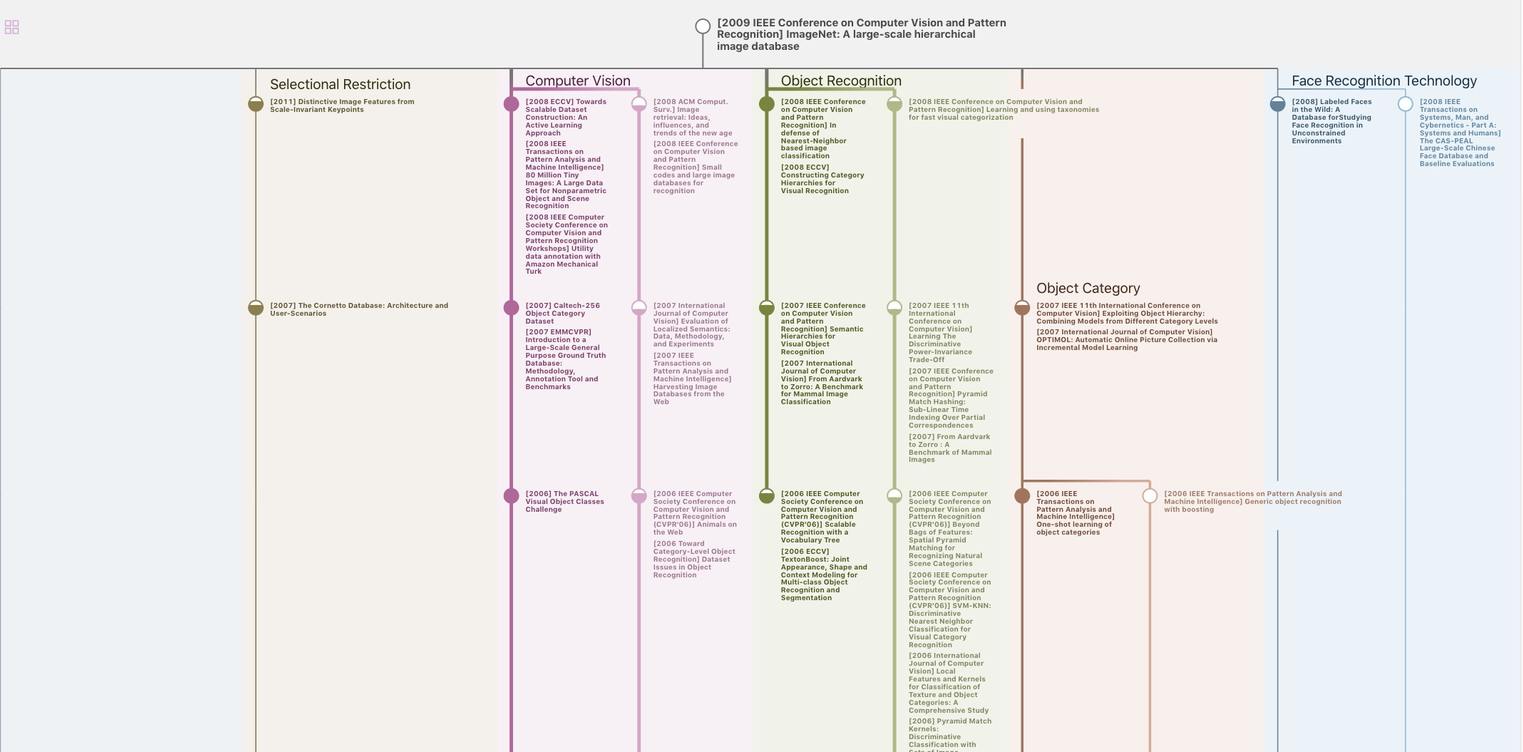
生成溯源树,研究论文发展脉络
Chat Paper
正在生成论文摘要