Exploring the Utility of Clip Priors for Visual Relationship Prediction
ICASSP 2024 - 2024 IEEE International Conference on Acoustics, Speech and Signal Processing (ICASSP)(2024)
摘要
This work explores the challenges of leveraging large-scale vision language models, such as CLIP, for visual relationship prediction (VRP), a task vital in understanding the relations between objects in a scene based on both image features and text descriptors. Despite its potential, we find that CLIP’s language priors are restrictive in effectively differentiating between various predicates for VRP. Towards this, we present CREPE (CLIP Representation Enhanced Predicate Estimation), which utilizes learnable prompts and a unique contrastive training strategy to derive reliable CLIP representations suited for VRP. CREPE can be seamlessly integrated into any VRP method. Our evaluations on the Visual Genome benchmark illustrate that using representations from CREPE significantly enhances the performance of vanilla VRP methods, such as UVTransE and VCTree. This enhancement is notable as CREPE can be seamlessly integrated into any VRP method, even without the need for additional calibration techniques, showcasing its efficacy as a powerful solution to VRP. CREPE’s performance on the Unrel benchmark reveals strong generalization to diverse and previously unseen predicate occurrences, despite lacking explicit training on such examples.
更多查看译文
关键词
Visual relationship prediction,vision-language models,CLIP,visual genome,prompt learning
AI 理解论文
溯源树
样例
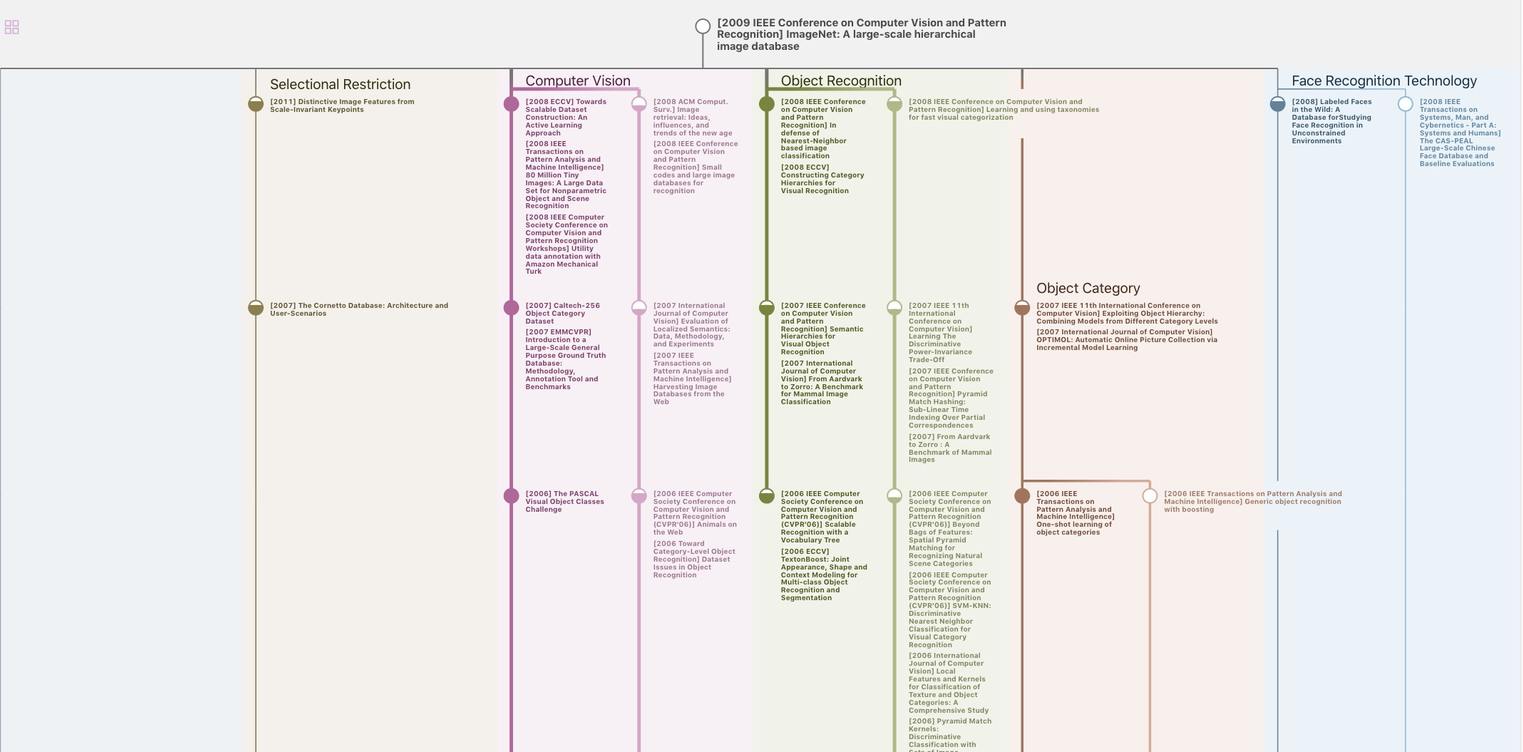
生成溯源树,研究论文发展脉络
Chat Paper
正在生成论文摘要