Can Gas Consumption Data Improve the Performance of Electricity Theft Detection?
IEEE TRANSACTIONS ON INDUSTRIAL INFORMATICS(2024)
摘要
Machine learning techniques have been extensively developed in the field of electricity theft detection. However, almost all typical models primarily rely on electricity consumption data to identify fraudulent users, often neglecting other pertinent household information such as gas consumption data. This article aims to explore the untapped potential of gas consumption data, a critical yet overlooked factor in electricity theft detection. In particular, we perform theoretical, qualitative, and quantitative correlation analyses between gas and electricity consumption data. Then, we propose two model-agnostic frameworks (i.e., multichannel network and twin network frameworks) to seamlessly integrate gas consumption data into machine learning models. Simulation results show a significant improvement in model performance when gas consumption data are incorporated using our proposed frameworks. Also, our proposed gas and electricity convolutional neural network, based on the proposed framework, demonstrates superior performance compared to classical and recent machine learning models on datasets with varying fraudulent ratios.
更多查看译文
关键词
Electricity,Correlation,Data models,Biological system modeling,Water heating,Power distribution,Informatics,Advanced metering infrastructure,deep learning,electricity theft,gas data,smart meter
AI 理解论文
溯源树
样例
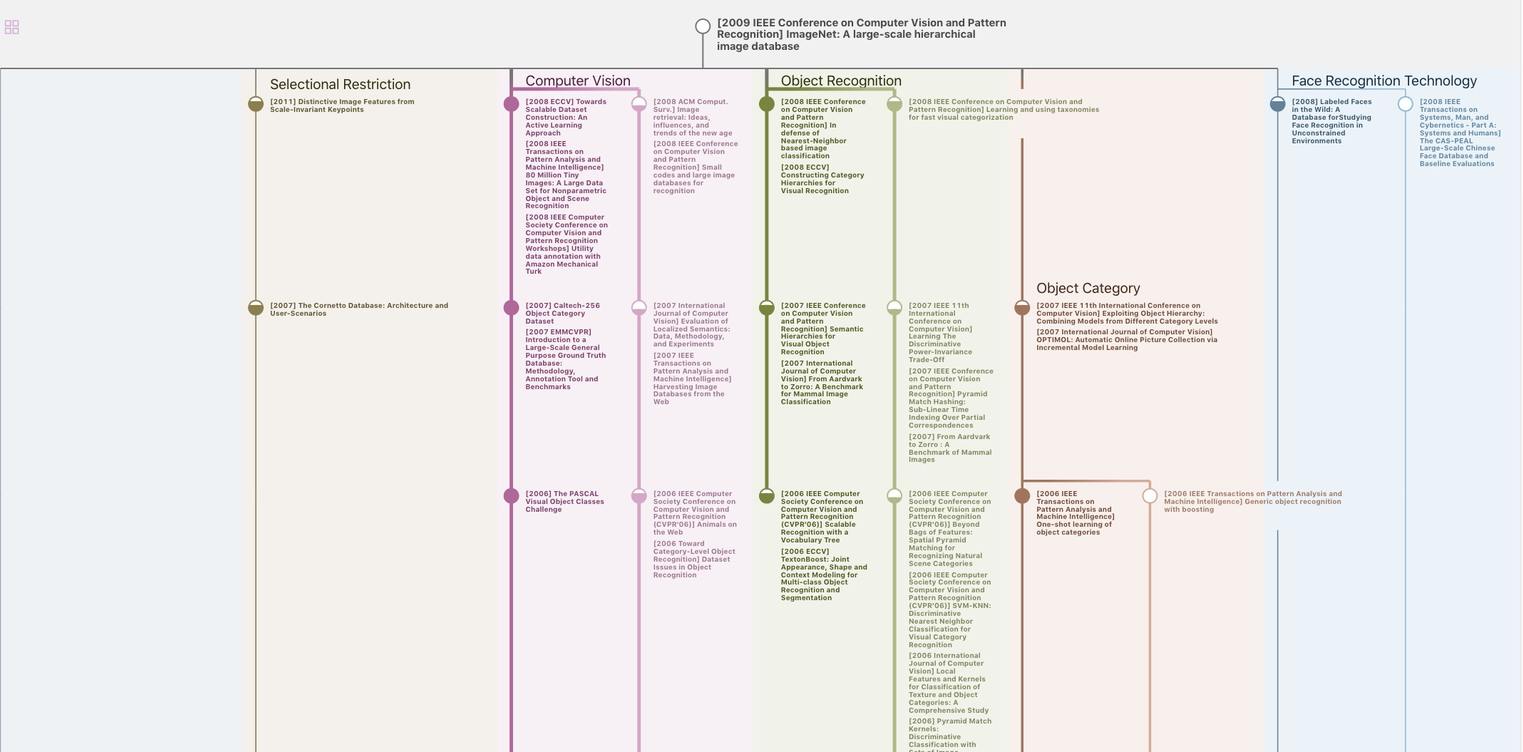
生成溯源树,研究论文发展脉络
Chat Paper
正在生成论文摘要