Unleashing Trigger-Free Event Detection: Revealing Event Correlations Via a Contrastive Derangement Framework
ICASSP 2024 - 2024 IEEE International Conference on Acoustics, Speech and Signal Processing (ICASSP)(2024)
摘要
Event detection (ED), detecting events with specified types observed in given texts, is critical to many downstream applications. Existing ED methods generally require high-quality triggers annotated by human experts, which is labor-intensive, especially for those nontrivial texts about breaking events. In this paper, we propose a novel trigger-free ED framework that detects multiple events from a given text without pre-defined triggers. Specifically, we first shed light on the event correlations with input texts using a joint embedding paradigm. Next, we devise derangement-based contrastive learning to model fine-grained correlations between multi-event instances. Since events in training benchmarks are usually imbalanced, we further design a simple yet effective event derangement module for balanced training. Experimental results on two benchmarks show that our trigger-free method is remarkably competitive to state-of-the-art trigger-based baselines.
更多查看译文
关键词
Event detection,trigger-free paradigm,multi-event correlations,balanced training
AI 理解论文
溯源树
样例
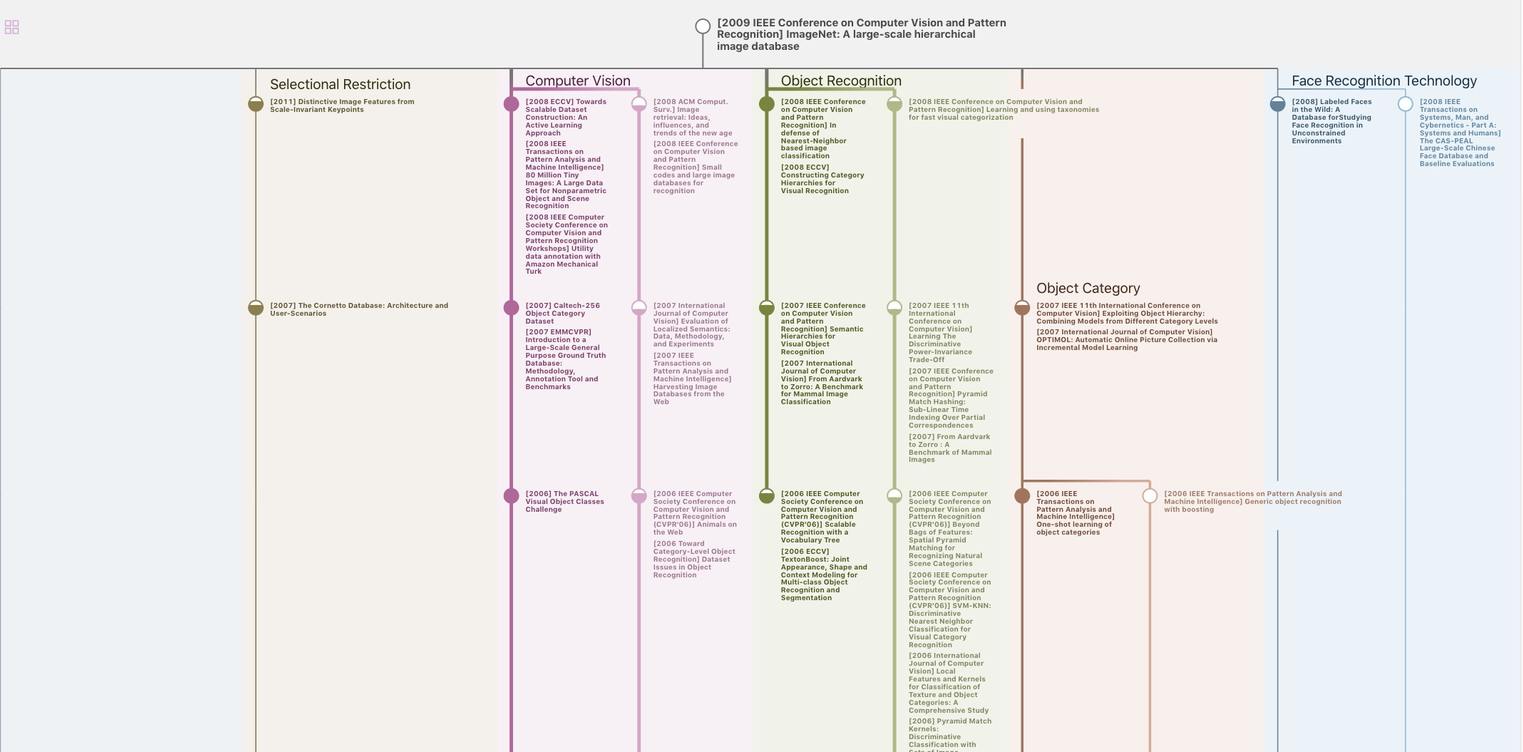
生成溯源树,研究论文发展脉络
Chat Paper
正在生成论文摘要