Machine learning prediction of refractory ventricular fibrillation in out-of-hospital cardiac arrest using features available to EMS
Resuscitation Plus(2024)
摘要
Background
Shock-refractory ventricular fibrillation (VF) or ventricular tachycardia (VT) is a treatment challenge in out-of-hospital cardiac arrest (OHCA). This study aimed to develop and validate machine learning models that could be implemented by emergency medical services (EMS) to predict refractory VF/VT in OHCA patients.
Methods
This was a retrospective study examining adult non-traumatic OHCA patients brought into the emergency department by Singapore EMS from the Pan-Asian Resuscitation Outcomes Study (PAROS) registry. Data from April 2010 to March 2020 were extracted for this study. Refractory VF/VT was defined as VF/VT persisting or recurring after at least one shock. Features were selected based on expert clinical opinion and availability to dispatch prior to arrival at scene. Multivariable logistic regression (MVR), LASSO and random forest (RF) models were investigated. Model performance was evaluated using receiver operator characteristic (ROC) area under curve (AUC) analysis and calibration plots.
Results
20,713 patients were included in this study, of which 860 (4.1%) fulfilled the criteria for refractory VF/VT. All models performed comparably and were moderately well-calibrated. ROC-AUC were 0.732 (95% CI, 0.695 – 0.769) for MVR, 0.738 (95% CI, 0.701 – 0.774) for LASSO, and 0.731 (95% CI, 0.690 – 0.773) for RF. The shared important predictors across all models included male gender and public location.
Conclusion
The machine learning models developed have potential clinical utility to improve outcomes in cases of refractory VF/VT OHCA. Prediction of refractory VF/VT prior to arrival at patient’s side may allow for increased options for intervention both by EMS and tertiary care centres.
更多查看译文
关键词
Machine learning,Prediction model,OHCA,ECPR,Refractory VF
AI 理解论文
溯源树
样例
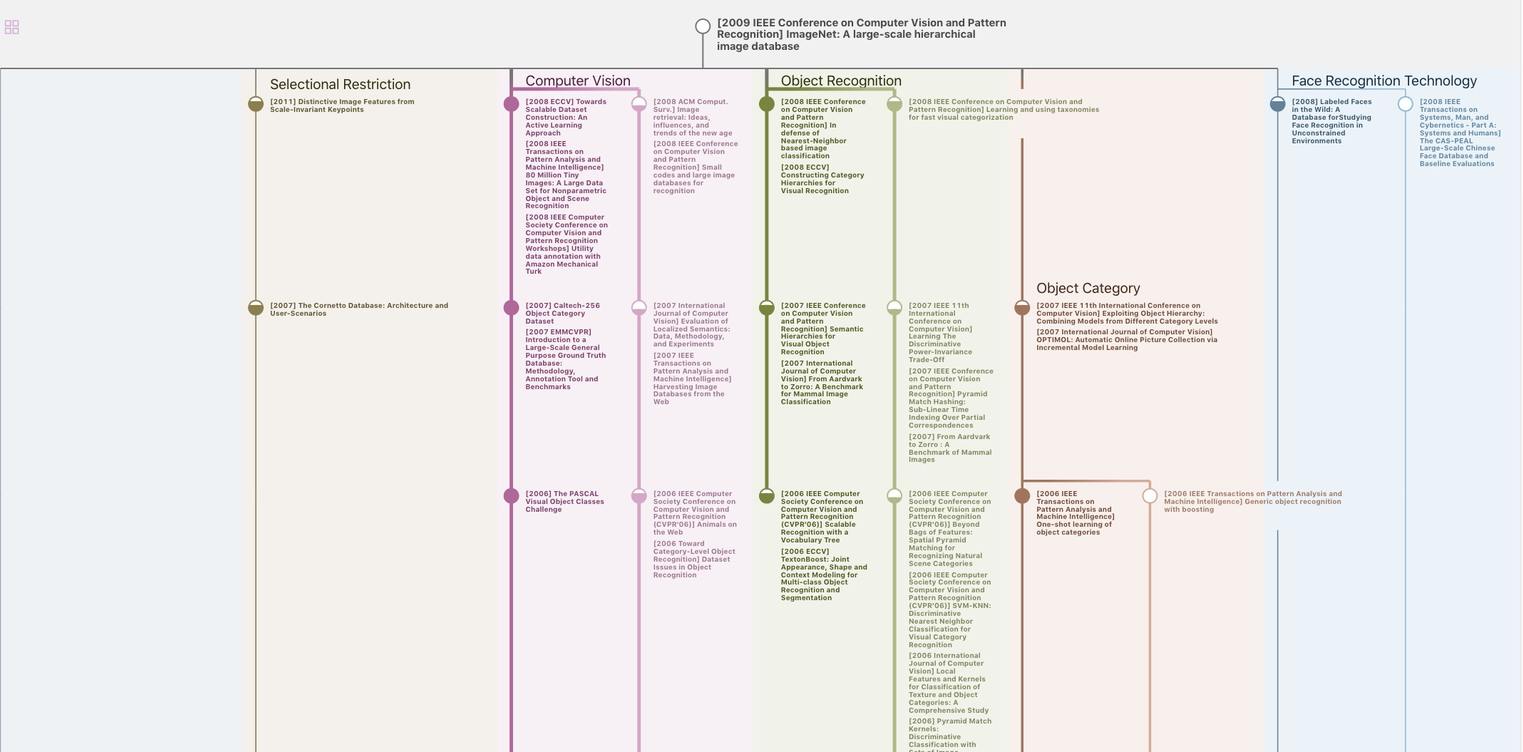
生成溯源树,研究论文发展脉络
Chat Paper
正在生成论文摘要