Segmentation of anatomical layers and imaging artifacts in intravascular polarization sensitive optical coherence tomography using attending physician and boundary cardinality losses.
Biomedical optics express(2024)
摘要
Intravascular ultrasound and optical coherence tomography are widely available for assessing coronary stenoses and provide critical information to optimize percutaneous coronary intervention. Intravascular polarization-sensitive optical coherence tomography (PS-OCT) measures the polarization state of the light scattered by the vessel wall in addition to conventional cross-sectional images of subsurface microstructure. This affords reconstruction of tissue polarization properties and reveals improved contrast between the layers of the vessel wall along with insight into collagen and smooth muscle content. Here, we propose a convolutional neural network model, optimized using two new loss terms (Boundary Cardinality and Attending Physician), that takes advantage of the additional polarization contrast and classifies the lumen, intima, and media layers in addition to guidewire and plaque shadows. Our model segments the media boundaries through fibrotic plaques and continues to estimate the outer media boundary behind shadows of lipid-rich plaques. We demonstrate that our multi-class classification model outperforms existing methods that exclusively use conventional OCT data, predominantly segment the lumen, and consider subsurface layers at most in regions of minimal disease. Segmentation of all anatomical layers throughout diseased vessels may facilitate stent sizing and will enable automated characterization of plaque polarization properties for investigation of the natural history and significance of coronary atheromas.
更多查看译文
AI 理解论文
溯源树
样例
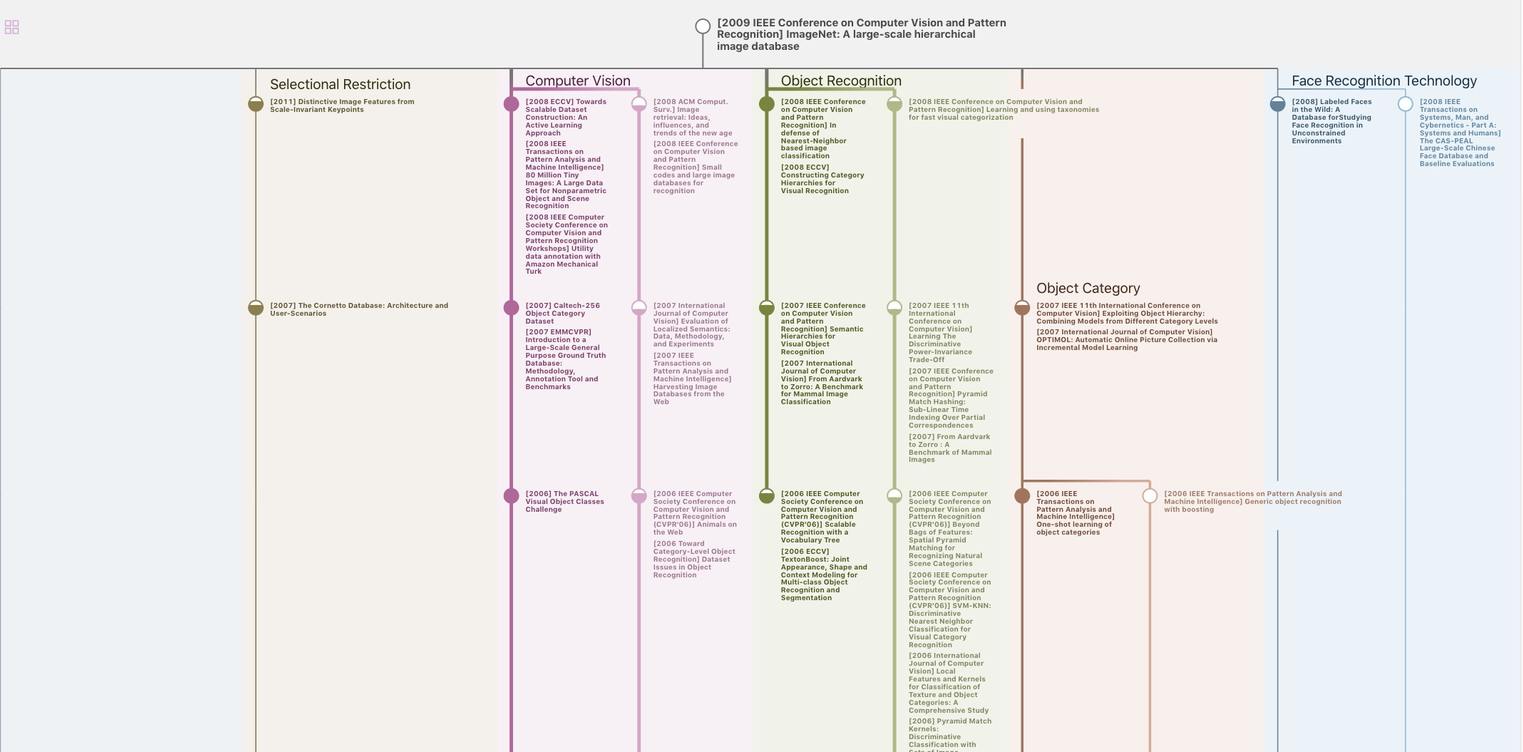
生成溯源树,研究论文发展脉络
Chat Paper
正在生成论文摘要