Robust Sparse Estimation for Gaussians with Optimal Error under Huber Contamination
arxiv(2024)
摘要
We study Gaussian sparse estimation tasks in Huber's contamination model with
a focus on mean estimation, PCA, and linear regression. For each of these
tasks, we give the first sample and computationally efficient robust estimators
with optimal error guarantees, within constant factors. All prior efficient
algorithms for these tasks incur quantitatively suboptimal error. Concretely,
for Gaussian robust k-sparse mean estimation on ℝ^d with
corruption rate ϵ>0, our algorithm has sample complexity
(k^2/ϵ^2)polylog(d/ϵ), runs in sample polynomial time,
and approximates the target mean within ℓ_2-error O(ϵ). Previous
efficient algorithms inherently incur error Ω(ϵ√(log(1/ϵ))). At the technical level, we develop a novel
multidimensional filtering method in the sparse regime that may find other
applications.
更多查看译文
AI 理解论文
溯源树
样例
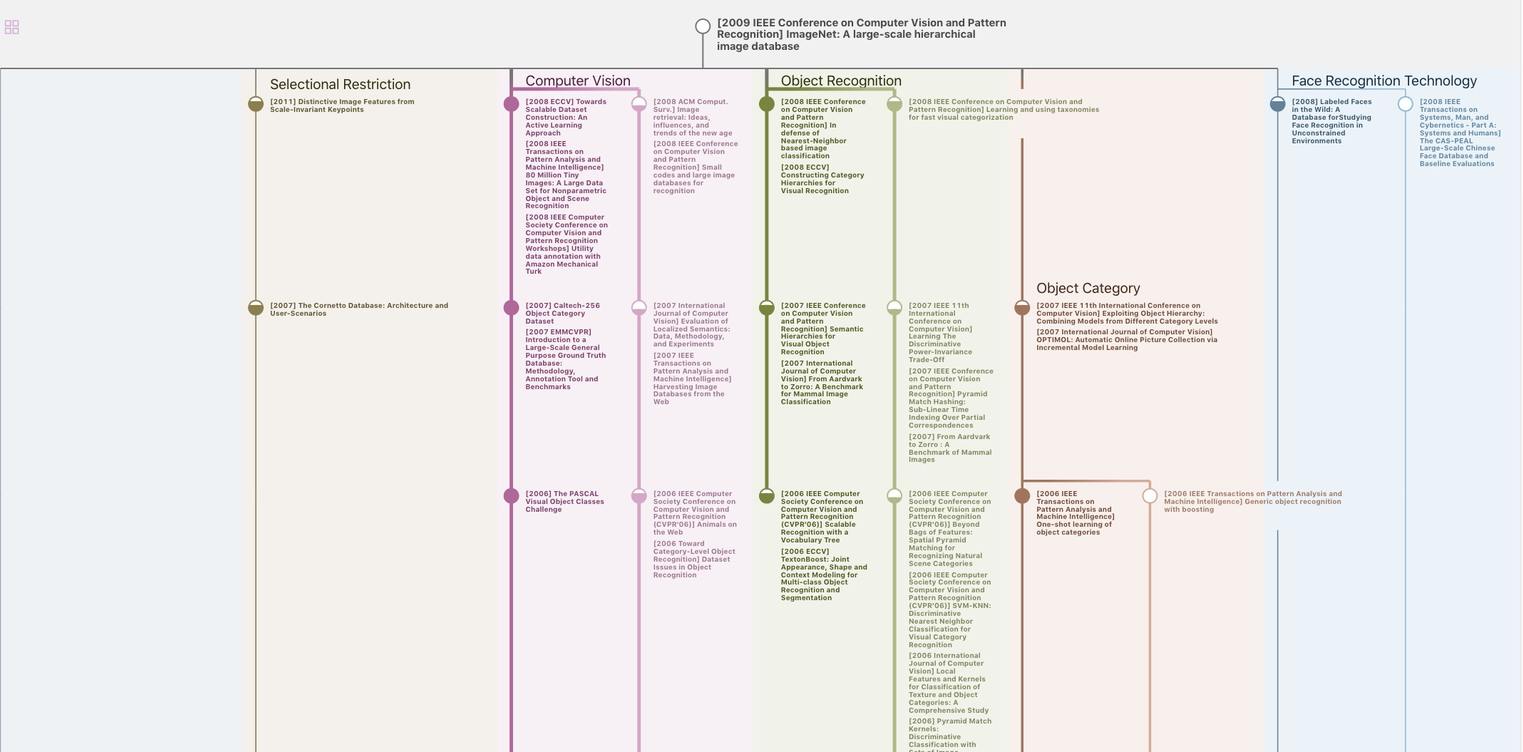
生成溯源树,研究论文发展脉络
Chat Paper
正在生成论文摘要